眯眼瞎说
甭管你查询接口咋实现,到最后也是拼 sql 打印查询结果
扯淡话题:
首先应该弄清楚线程/进程和并发/并行的区别吧??
Python 中的 mutiprocessing 模块是多进程模块、而非多线程模块;
其次,你应该弄清楚自己的任务是 CPU 密集型还是 I/O 密集型的:
如果是 CPU 密集型的,因为 CPython 中 GIL 的存在,多线程帮不了你什么,用 mutiprocessing 或者 concurrent.futures 进程池用多进程解决问题就好;
如果是 I/O 密集型,就用 Threading/concurrent.futures 线程池解决问题,或者用 asyncio 做异步
具体多线程/多进程和"多开"的效率我并没有比较过,我认为应该相差不大。
链接:https://www.zhihu.com/question/265723439/answer/298688700
1. 进程 是系统进行资源分配和调度的基础单位、也就是说进程有独立内存,只有进程能向CPU申请自己的执行时间
2. 线程 - 进程有很多子线程,反正意思就是线程系统能调配执行的最小的执行单位
3. IO、Bio、Nio、Aio
- IO:
inputoutput
- Bio:
阻塞
Blocking IO
- Nio
非阻塞
Non-Blocking IO
- Aio
异步
Asynchronous IO
4. 同步、异步、阻塞、非阻塞 之间的差异
概念:
- 同步阻塞
- 同步不阻塞
- 异步阻塞
- 异步不阻塞
关注点:
- 同步和异步 关注的是消息通信机制
- 阻塞和非阻塞 关注的是程序在等待调用结果(消息,返回值)时候的状态
存在的意义:
- 同步阻塞 : 自己取获取结果 这个过程不能干别的事情
- 同步非阻塞 : 自己取结果,在没有取到结果之前你可以去干别的事情
- 异步阻塞 : 不需要自己去取结果,别人送上门,在等的过程中自己不能干别的。
- 异步非阻塞:不需要自己取去结果, 别人把结果送上门,这个等结果的过程自己可干别的
个人建议:
- 异步和非阻塞一起配合使用才能发挥异步效果
- 反正就是这样用就对了
5. 并发和并行的区别(没错、任何难以解释都统称“玄学”)
英文:
- 并发: concurrency
- 并行: parallellism
在 Loadrunner 层面解释:
- 并发: 多个事件在同一时间间隔发生
- 并行: 多个事件在同一时刻发生
并发细分:
- 同时刻,在多个CPU上,多线程执行。
- 宏观: 同段时间段内多个线程在执行。
- 微观: 同时刻,只有一个线程在执行,同时间段内,多个线程不断轮换。
并行细分:
- 同时刻,在多个CPU上,多线程执行。
- 宏观 + 微观: 同时刻,多线程同时进行。
- 反正就是理解就对了,并非一类东西就对了
6. 集型分类:
- 计算密集型(CPU-Intensive)
1、特点:要进行大量的计算,消耗CPU资源。比如计算圆周率、对视频进行高清解码等等,全靠CPU的运算能力。
2、计算密集型任务虽然也可以用多任务完成,但是任务越多,花在任务切换的时间就越多,CPU执行任务的效率就越低,所以,要最高效地利用CPU,计算密集型任务同时进行的数量应当等于CPU的核心数。
3、计算密集型任务由于主要消耗CPU资源,因此,代码运行效率至关重要。
- IO密集型(IO-Intensive)
1、涉及到网络、磁盘IO的任务都是IO密集型任务。
2、特点:CPU消耗很少,任务的大部分时间都在等待IO操作完成(因为IO的速度远远低于CPU和内存的速度)。
3、对于IO密集型任务,任务越多,CPU效率越高,但也有一个限度。常见的大部分任务都是IO密集型任务,比如Web应用。
4、IO密集型任务执行期间,99%的时间都花在IO上,花在CPU上的时间很少。
- 数据密集(Data-Intensive)
1、大量独立的数据分析处理作业可以分布在松耦合的计算机集群系统的不同节点上运行;
2、高度密集的海量数据I/O吞吐需求;
3、大部分数据密集型应用都有个数据流驱动的流程。
7. 消息队列(Message Queue)具体是打酱油还是别有用处
介绍:https://www.jianshu.com/p/19a94c1c729b
不理解 ???
- 挽尊右转 ---→ _ < 百度一下,知不知看脸又看人品 > _
Unix/linux下⑤种I/O模型
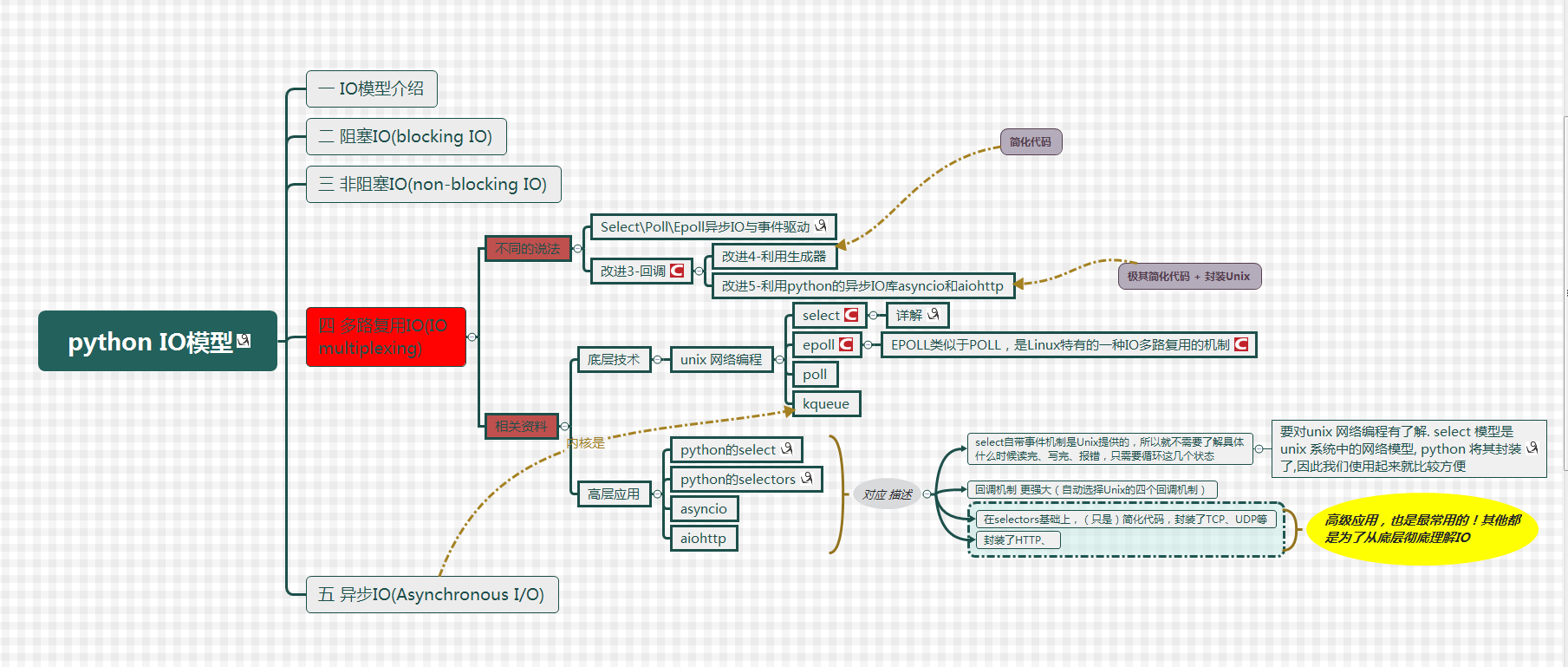
最大视角-了解相关概念(网上找到的流程图)
模型图 - 折叠
阻塞式 I/O
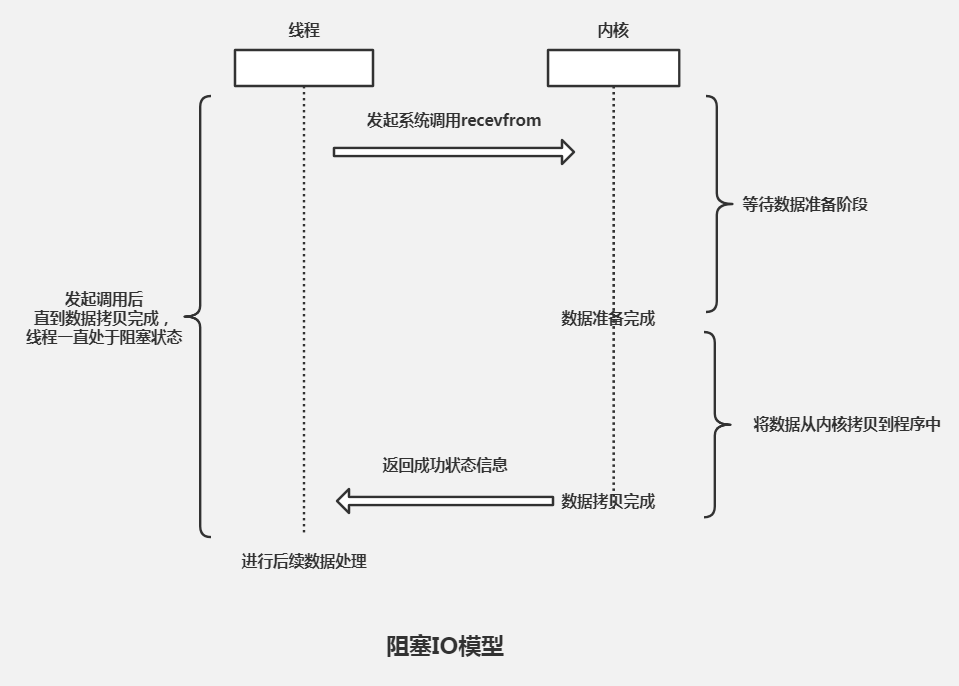
阻塞式 I/O
非阻塞式 I/O
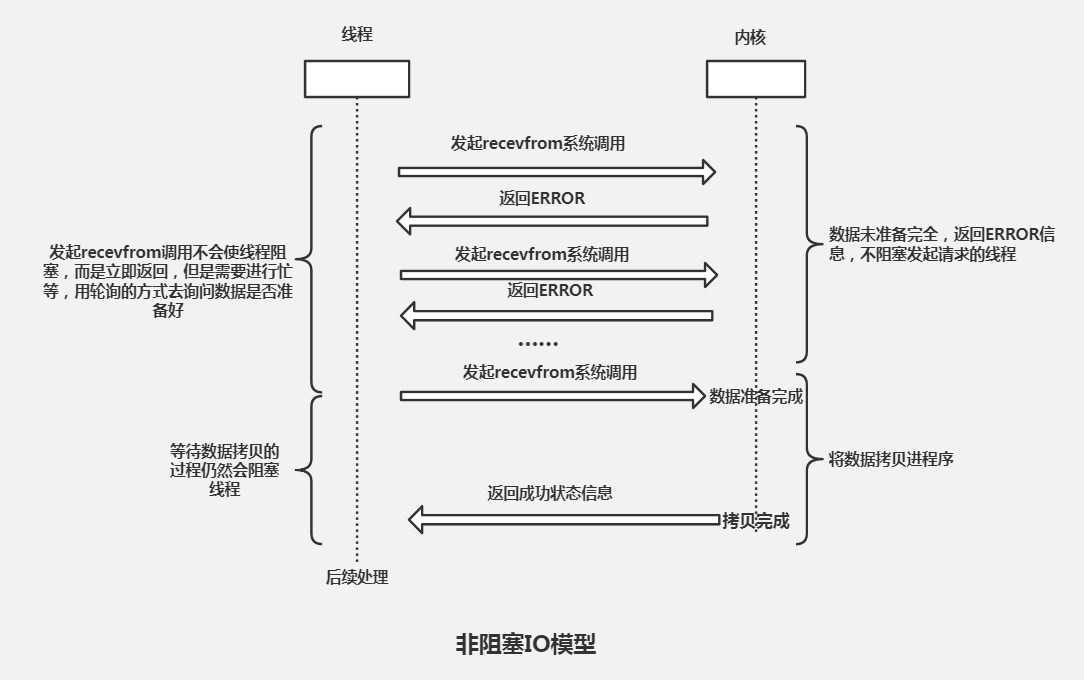
非阻塞式 I/O
I/O 多路复用
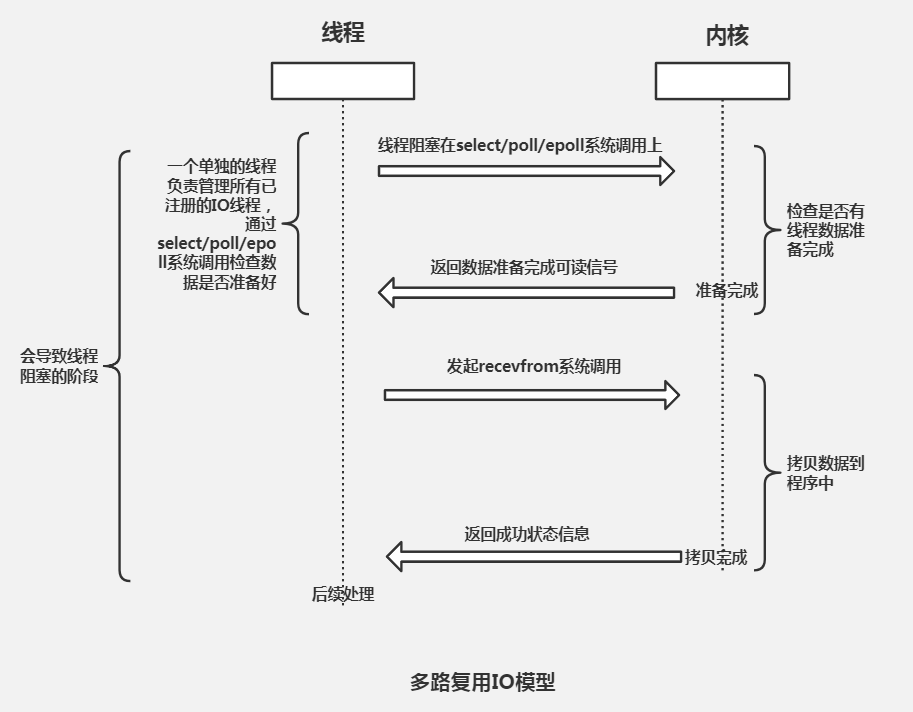
I/O 多路复用
信号驱动式 I/O
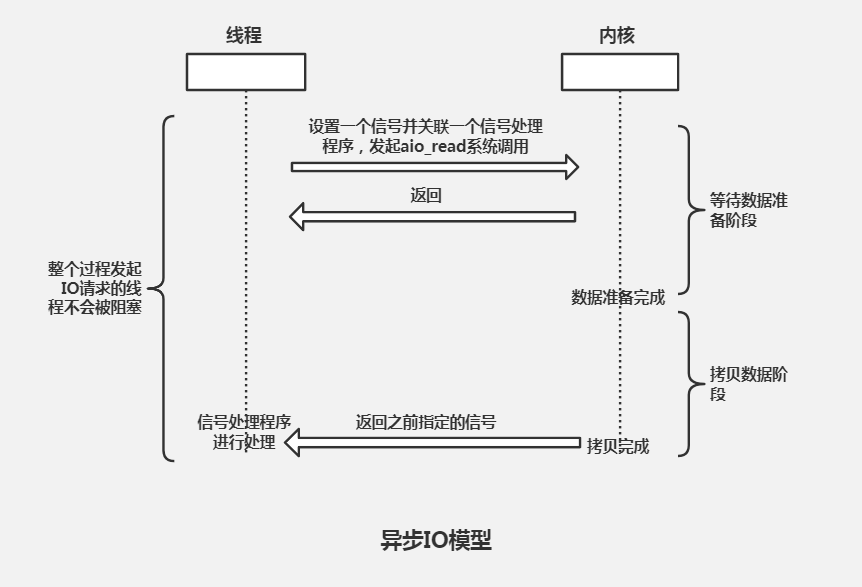
信号驱动式 I/O
异步 I/O(- asyncio)
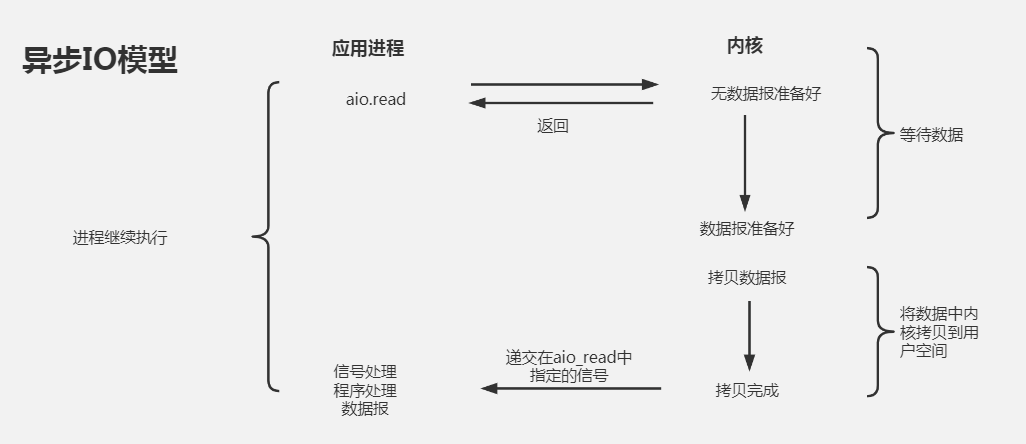
异步 I/O
总结
- 实际上I/O模型是对应发展的关系,最开始的时候都是 阻塞式 I/O 随着我们的需要慢慢的衍生出 非阻塞式 I/O 、 I/O 多路复用 等等
请求处理
A 类型请求:
[ /Getuser ] → [ User=DB.User_Search() ] -- "select * from User" → ≈≈ [ 数据库耗时:400 ms ] →查询结果→ [ User.json ] → {展示json}
B 类型请求:
[ /Getuser/1 ] → [ User1=DB.User_Search() ] -- "select * from User where id = 1" → ≈≈≈ [ 数据库耗时:10 ms ] →查询结果→ → {展示json}
[ /Getuser/2 ] → [ User2=DB.User_Search() ] -- "select * from User where id = 2" → ≈≈≈ [ 数据库耗时:10 ms ] →查询结果→ → {展示json}
从例子看出来,说明:
A 类型属于堵塞操作,中间什么事都干不了,一次性获取所有用户信息;
B 类型获取列表块,也叫“分页读取数据”
两种方式都是单进程|单线程执行,反正都是慢,非常尴尬
消息队列
[ /Getuser/1 ] → [ User1=DB.User_Search() ] → [ ↓↓ ] → -- "select * from User where id = 1" → ≈≈≈ [ 数据库耗时:10 ms ] →查询结果→ → {返回给 mq} -→ {展示结果}
[ /Getuser/2 ] → [ User2=DB.User_Search() ] → [ mq ] → -- "select * from User where id = 2" → ≈≈≈ [ 数据库耗时:10 ms ] →查询结果→ → {返回给 mq} -→ {展示结果}
[ /Getuser/3 ] → [ User3=DB.User_Search() ] → [ ↑↑ ] → -- "select * from User where id = 3" → ≈≈≈ [ 数据库耗时:10 ms ] →查询结果→ → {返回给 mq} -→ {展示结果}
说明:
消息队列(Message Queue)是一种进程间通信或同一进程的不同线程间的通信方式
从例子看所有请求都经过 [ mq ],也只有它能和数据库进行通讯与信息交互
消息队列工具:
通过 RedisKafka 中间件做缓存;
消息量不大,可以使用redis做mq;
Redis 有可能扛不住被击穿;
Kafka 比较适合大吞吐量的消息,例如系统日志,kafka+elk是经典的日志收集系统。
高并发
- 因大部分业务系统上都是从数据库或者其他存储设备上创建、读取、修改、删除内容(有没有很明显感觉到 sql 的知识点);
- 经过系统筛选过滤数据,给用户呈现展示最终的结果,例如上边说的“获取用户信息”;
- 根据不同的业务系统硬件层面的负载也不一样,例如咱们上边说的“获取用户信息”例子,是个很典型的数据密集计算类型
实现例子
前期
线程 - 阻塞
#!/usr/bin/env python
# -*- coding: utf-8 -*-
# @File : thread_not_blocking.py
# @Author : BenLam
# @Link : https://www.cnblogs.com/BenLam/
import time
import threading
def run():
print(f"线程- {threading.currentThread().name} 开始")
time.sleep(2)
print(f"线程- {threading.currentThread().name} 结束")
def main():
print("--- 主线程 ---")
li = []
for _ in range(2):
thread = threading.Thread(target=run, args=())
li.append(thread)
for _ in li:
_.start()
for _ in li:
_.join() # 相当于阻塞状态,Join意义就是等子线程执行完毕再走主线程 - 一直到主线程执行结束
print("--- 主线程 | 关闭 ---")
if __name__ == '__main__':
main()
"""
打印输出:
--- 主线程 ---
线程- Thread-1 开始
线程- Thread-2 开始
线程- Thread-2 结束
线程- Thread-1 结束
--- 主线程 | 关闭 ---
[Done] exited with code=0 in 2.563 seconds
"""
- Threading 加入 join() 方法相当于阻塞线程,具体分为两个步骤
- 第①步:主线程执行 → 通知 1号子线程 执行 → 通知 2号子线程 执行,并等所有子线程执行结束
- 第②步:第二个子线程执行结束 → 第一个子线程执行结束 → 主线程执行结束 → 打印消息
废弃代码 - 折叠
#!/usr/bin/env python
# -*- coding: utf-8 -*-
# @File : thread_blocking.py
# @Author : BenLam
# @Link : https://www.cnblogs.com/BenLam/
import time
# import threading
def run(t):
print("线程- %s 开始" %t)
time.sleep(1)
print("线程- %s 结束" %t)
def main():
restful = False
print("--- 主线程 ---")
for _ in range(1, 3):
run(_)
restful = True
print("--- 主线程 | 关闭 ---")
return restful
if __name__ == '__main__':
print(time.strftime("%Y-%m-%d %H:%M:%S", time.localtime()))
t = main()
print("返回结果为 - %s" %t)
print(time.strftime("%Y-%m-%d %H:%M:%S", time.localtime()))
"""
打印:
2020-04-16 14:06:09
--- 主进程 ---
线程- 1 开始
线程- 1 结束
线程- 2 开始
线程- 2 结束
--- 主进程 | 关闭 ---
返回结果为 - True
2020-04-16 14:06:11
[Finished in 2.5s]
"""
- 从上边单线程执行结果看,比较容易理解“同步-阻塞”是什么意思了
- main() 方法下通过 for 循环调用 2 次 run() 执行
- for 循环相当于水管, run() 则为管道中的水,唯有 run() 执行完毕才会再执行 print() 函数
- 别误认为加了 sleep 就影响运行结果
线程 - 非阻塞
# !/usr/bin/env python
# -*- coding: utf-8 -*-
# @File : thread_not_blocking.py
# @Author : BenLam
# @Link : https://www.cnblogs.com/BenLam/
import time
import requests
import threading
from concurrent.futures import ThreadPoolExecutor
url_list = [
"http://www.cnblogs.com/",
"http://huaban.com/favorite/beauty/",
"http://www.bing.com",
"http://www.zhihu.com",
"http://www.sina.com",
"https://www.baidu.com",
]
def request(url):
return requests.get(url)
def callback(future, *args, **kwargs):
_response = future.result()
print(f"当前线程名: - {threading.currentThread().name}|状态码: {_response.status_code}")
def job_2(count):
print(f"能干其他的事情......{count}")
def main():
print("--- 主线程 ---")
executor = ThreadPoolExecutor(max_workers=3)
for url in url_list:
executor.submit(request, url).add_done_callback(callback)
executor.shutdown(wait=False)
with ThreadPoolExecutor(max_workers=1) as executor:
executor.map(job_2, range(5))
print("--- 主线程 | 关闭 ---")
if __name__ == "__main__":
main()
"""
打印:
--- 主线程 ---
能干其他的事情......0
能干其他的事情......1
能干其他的事情......2
能干其他的事情......3
能干其他的事情......4
--- 主线程 | 关闭 ---
当前线程名: - ThreadPoolExecutor-0_0|状态码: 200
当前线程名: - ThreadPoolExecutor-0_1|状态码: 200
当前线程名: - ThreadPoolExecutor-0_2|状态码: 200
当前线程名: - ThreadPoolExecutor-0_2|状态码: 200
当前线程名: - ThreadPoolExecutor-0_1|状态码: 200
当前线程名: - ThreadPoolExecutor-0_0|状态码: 400
[Done] exited with code=0 in 6.144 seconds
"""
废弃代码 - 折叠
#!/usr/bin/env python
# -*- coding: utf-8 -*-
# @File : thread_not_blocking.py
# @Author : BenLam
# @Link : https://www.cnblogs.com/BenLam/
import time
import threading
def run():
print(f"线程- {threading.currentThread().name} 开始")
time.sleep(2)
print(f"线程- {threading.currentThread().name} 结束")
def main():
print("--- 主线程 ---")
li = []
for _ in range(2):
thread = threading.Thread(target=run, args=())
li.append(thread)
for _ in li:
_.start()
print("--- 主线程 | 关闭 ---")
if __name__ == '__main__':
main()
"""
打印输出:
--- 主线程 ---
线程- Thread-1 开始
线程- Thread-2 开始
--- 主线程 | 关闭 ---
线程- Thread-2 结束
线程- Thread-1 结束
[Done] exited with code=0 in 2.547 seconds
"""
- 先看打印结果,因没有加入 join() 方法
- 第①步:主线程执行 → 通知 1号子线程 执行 → 通知 2号子线程 执行,主线程不关心子线程干嘛继续往下执行
- 第②步:主线程执行结束后就会一直等待子线程结束,如果加入 [ setDaemon(True) - 守护线程 ] 结果就不一样了
- 第③步:主线程设置为 → 守护线程并执行完毕 → 就不管其它子线程是否完成一并和主线程退出
def main():
print("--- 主线程 ---")
li = []
for _ in range(2):
thread = threading.Thread(target=run, args=())
li.append(thread)
for _ in li:
_.setDaemon(True)
_.start()
print("--- 主线程 | 关闭 ---")
"""
打印输出:
--- 主线程 ---
线程- Thread-1 开始
线程- Thread-2 开始
--- 主线程 | 关闭 ---
[Done] exited with code=0 in 0.621 seconds
"""
- 结果就没有子线程关闭的打印输出
多路复用
- 参考例子: 跳转链接
异步
- 参考例子: 跳转链接