demo代码:
# _*_coding:UTF-8_*_ import numpy as np import sys import pandas as pd from pandas import Series,DataFrame import numpy as np import sys from sklearn import preprocessing from sklearn.ensemble import ExtraTreesClassifier import os from minepy import MINE def iterbrowse(path): for home, dirs, files in os.walk(path): for filename in files: yield os.path.join(home, filename) def get_data(filename): white_verify = [] with open(filename) as f: lines = f.readlines() data = {} for line in lines: a = line.split(" ") if len(a) != 78: print(line) raise Exception("fuck") white_verify.append([float(n) for n in a[3:]]) return white_verify if __name__ == '__main__': # pdb.set_trace() neg_file = "cc_data/black_all.txt" pos_file = "cc_data/white_all.txt" X = [] y = [] if os.path.isfile(pos_file): if pos_file.endswith('.txt'): pos_set = np.genfromtxt(pos_file) elif pos_file.endswith('.npy'): pos_set = np.load(pos_file) X.extend(pos_set) y += [0] * len(pos_set) if os.path.isfile(neg_file): if neg_file.endswith('.txt'): neg_set = np.genfromtxt(neg_file) elif neg_file.endswith('.npy'): neg_set = np.load(neg_file) ''' X.extend(list(neg_set) * 5) y += [1] * (5 * len(neg_set)) ''' X.extend(neg_set) y += [1] * len(neg_set) print("len of X:", len(X)) print("X sample:", X[:3]) print("len of y:", len(y)) print("y sample:", y[:3]) X = [x[3:] for x in X] print("filtered X sample:", X[:3]) cols = [str(i + 6) for i in range(len(X[0]))] clf = ExtraTreesClassifier() clf.fit(X, y) print (clf.feature_importances_) print "Features sorted by their score:" print sorted(zip(clf.feature_importances_, cols), reverse=True) black_verify = [] for f in iterbrowse("todo/top"): print(f) black_verify += get_data(f) # ValueError: operands could not be broadcast together with shapes (1,74) (75,) (1,74) print(black_verify) black_verify_labels = [3] * len(black_verify) white_verify = get_data("todo/white_verify.txt") print(white_verify) white_verify_labels = [2] * len(white_verify) unknown_verify = get_data("todo/pek_feature74.txt") print(unknown_verify) # extend data X = np.concatenate((X, black_verify)) y += black_verify_labels X = np.concatenate((X, white_verify)) y += white_verify_labels #################################### plot #################################### data_train = pd.DataFrame(X) # cols = [str(i) for i in range(6, 81)] data_train.columns = cols # add label column # data_train = data_train.assign(label=pd.Series(y)) data_train["label"] = pd.Series(y) print(data_train.info()) print(data_train.columns) import matplotlib.pyplot as plt for col in cols: fig = plt.figure(figsize=(20, 16), dpi=8) fig.set(alpha=0.2) plt.figure() data_train[data_train.label == 0.0][col].plot() data_train[data_train.label == 1.0][col].plot() data_train[data_train.label == 2.0][col].plot() data_train[data_train.label == 3.0][col].plot() plt.xlabel(u"sample data id") plt.ylabel(u"value") plt.title(col) plt.legend((u'white', u'black', u"white-todo", u"black-todo"), loc='best') plt.show() print "calculate MINE mic value:" for col in cols: print col, mine = MINE(alpha=0.6, c=15, est="mic_approx") # http://minepy.readthedocs.io/en/latest/python.html#second-example mine.compute_score(data_train[col], y) print "MIC=", mine.mic() sys.exit(-1)
extend data 表示待预测的数据
关于mic:
from __future__ import division
import numpy as np
import matplotlib.pyplot as plt
from minepy import MINE
rs = np.random.RandomState(seed=0)
def mysubplot(x, y, numRows, numCols, plotNum,
xlim=(-4, 4), ylim=(-4, 4)):
r = np.around(np.corrcoef(x, y)[0, 1], 1)
mine = MINE(alpha=0.6, c=15, est="mic_approx")
mine.compute_score(x, y)
mic = np.around(mine.mic(), 1)
ax = plt.subplot(numRows, numCols, plotNum,
xlim=xlim, ylim=ylim)
ax.set_title('Pearson r=%.1f
MIC=%.1f' % (r, mic),fontsize=10)
ax.set_frame_on(False)
ax.axes.get_xaxis().set_visible(False)
ax.axes.get_yaxis().set_visible(False)
ax.plot(x, y, ',')
ax.set_xticks([])
ax.set_yticks([])
return ax
def rotation(xy, t):
return np.dot(xy, [[np.cos(t), -np.sin(t)], [np.sin(t), np.cos(t)]])
def mvnormal(n=1000):
cors = [1.0, 0.8, 0.4, 0.0, -0.4, -0.8, -1.0]
for i, cor in enumerate(cors):
cov = [[1, cor],[cor, 1]]
xy = rs.multivariate_normal([0, 0], cov, n)
mysubplot(xy[:, 0], xy[:, 1], 3, 7, i+1)
def rotnormal(n=1000):
ts = [0, np.pi/12, np.pi/6, np.pi/4, np.pi/2-np.pi/6,
np.pi/2-np.pi/12, np.pi/2]
cov = [[1, 1],[1, 1]]
xy = rs.multivariate_normal([0, 0], cov, n)
for i, t in enumerate(ts):
xy_r = rotation(xy, t)
mysubplot(xy_r[:, 0], xy_r[:, 1], 3, 7, i+8)
def others(n=1000):
x = rs.uniform(-1, 1, n)
y = 4*(x**2-0.5)**2 + rs.uniform(-1, 1, n)/3
mysubplot(x, y, 3, 7, 15, (-1, 1), (-1/3, 1+1/3))
y = rs.uniform(-1, 1, n)
xy = np.concatenate((x.reshape(-1, 1), y.reshape(-1, 1)), axis=1)
xy = rotation(xy, -np.pi/8)
lim = np.sqrt(2+np.sqrt(2)) / np.sqrt(2)
mysubplot(xy[:, 0], xy[:, 1], 3, 7, 16, (-lim, lim), (-lim, lim))
xy = rotation(xy, -np.pi/8)
lim = np.sqrt(2)
mysubplot(xy[:, 0], xy[:, 1], 3, 7, 17, (-lim, lim), (-lim, lim))
y = 2*x**2 + rs.uniform(-1, 1, n)
mysubplot(x, y, 3, 7, 18, (-1, 1), (-1, 3))
y = (x**2 + rs.uniform(0, 0.5, n)) *
np.array([-1, 1])[rs.random_integers(0, 1, size=n)]
mysubplot(x, y, 3, 7, 19, (-1.5, 1.5), (-1.5, 1.5))
y = np.cos(x * np.pi) + rs.uniform(0, 1/8, n)
x = np.sin(x * np.pi) + rs.uniform(0, 1/8, n)
mysubplot(x, y, 3, 7, 20, (-1.5, 1.5), (-1.5, 1.5))
xy1 = np.random.multivariate_normal([3, 3], [[1, 0], [0, 1]], int(n/4))
xy2 = np.random.multivariate_normal([-3, 3], [[1, 0], [0, 1]], int(n/4))
xy3 = np.random.multivariate_normal([-3, -3], [[1, 0], [0, 1]], int(n/4))
xy4 = np.random.multivariate_normal([3, -3], [[1, 0], [0, 1]], int(n/4))
xy = np.concatenate((xy1, xy2, xy3, xy4), axis=0)
mysubplot(xy[:, 0], xy[:, 1], 3, 7, 21, (-7, 7), (-7, 7))
plt.figure(facecolor='white')
mvnormal(n=800)
rotnormal(n=200)
others(n=800)
plt.tight_layout()
plt.show()
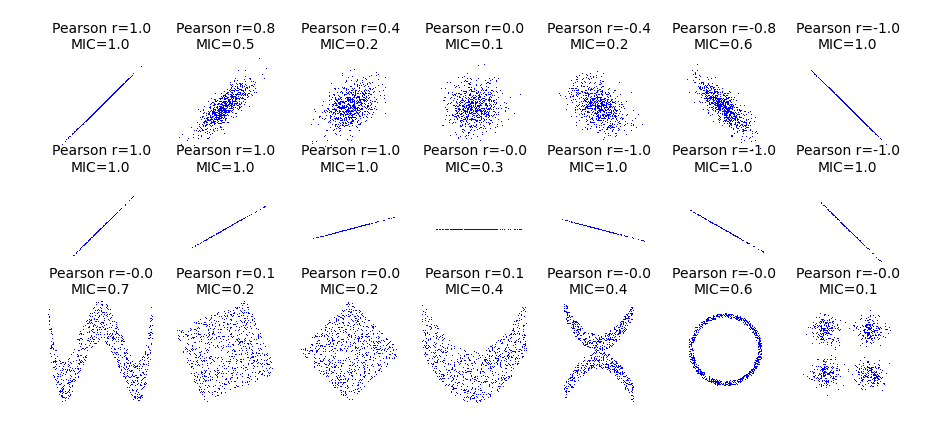