先看数据:
Number of seconds elapsed between each transaction (over two days)
abc
Amount of money for this transaction
Fraud or Not-Fraud
Introduction
from:https://www.kaggle.com/nikitaivanov/getting-high-sensitivity-for-imbalanced-data 主要使用了smote和聚类两种思路!
In this notebook we will try to predict fraud transactions from a given data set. Given that the data is imbalanced, standard metrics for evaluating classification algorithm (such as accuracy) are invalid. We will focus on the following metrics: Sensitivity (true positive rate) and Specificity (true negative rate). Of course, they are dependent on each other, so we want to find optimal trade-off between them. Such trade-off usually depends on the application of the algorithm, and in case of fraud detection I would prefer to see high sensitivity (e.g. given that a transaction is fraud, I want to be able to detect it with high probability).
For dealing with skewed data I am going to use SMOTE algorithm. In two words, the idea is to create synthetic samples (in opposite to oversampling with replacement) through finding nearest examples (KNN), calculating difference between them, multiplying this difference by a random number between 0 and 1 and adding the result to the initial sample. For this purpose we are going to use SMOTE
function from DMwR
package.
Algorithms I am going to implement are Support Vector Machine (SVM), Logistic regression and Random Forest. Models will be trained on the original and SMOTEd data and their performance will be measured on the entire data set.
As a bonus, we are going to have some fun and use K-means centroids of the negative examples together with the original positive examples as a new dataset and train our algorithm on it. We then compare results.
##Loading required packeges
library(ggplot2) #visualization
library(caret) #train model
library(dplyr) #data manipulation
library(kernlab) #svm
library(nnet) #models (logit, neural nets)
library(DMwR) #SMOTE data
##Load data
d = read.csv("../input/creditcard.csv")
n = ncol(d)
str(d)
d$Class = ifelse(d$Class == 0, 'No', 'Yes') %>% as.factor()
It is always a good idea first to plot a response variable to check for skewness in data:
qplot(x = d$Class, geom = 'bar') + xlab('Fraud (Yes/No)') + ylab('Number of transactions')
Classification on the original data
Keeping in mind that the data is highly skewed we proceed. First split the data into training and test sets.
idx = createDataPartition(d$Class, p = 0.7, list = F)
d[, -n] = scale(d[, -n]) #perform scaling
train = d[idx, ]
test = d[-idx, ]
Calculate baseline accuracy for future reference
blacc = nrow(d[d$Class == 'No', ])/nrow(d)*100
cat('Baseline accuracy:', blacc)
To begin with, let's train our models on the original dataset to see what we get if use unbalanced data. Due to computational limitations of my laptop, I will only run logistic regression for this purpose.
m1 = multinom(data = train, Class ~ .)
p1 = predict(m1, test[, -n], type = 'class')
cat(' Accuracy of the model', mean(p1 == test[, n])*100, '
', 'Baseline accuracy', blacc)
Though accuracy (99.92%) of the model might look impressive at a first glance, in fact it isn't. Simply predicting 'not a fraud' for all transactions will give 99.83% accuracy. To really evaluate model's perfomance we need to check confusion matrix.
confusionMatrix(p1, test[, n], positive = 'Yes')
From the confusion matrix we see that while model has high accuracy (99.92%) and high specificity (99.98%), it has low sensitivity of 64%. In other words, only 64% of all fraudulent transactions were detected.
Classification on the SMOTEd data
Now let's preprocess our data using SMOTE algorithm:
table(d$Class) #check initial distribution
newData <- SMOTE(Class ~ ., d, perc.over = 500,perc.under=100)
table(newData$Class) #check SMOTed distribution
To train SVM (with RBF kernel) we are going to use train
function from caret
package. It allows to choose optimal parameters of the model (cost and sigma in this case). Cost refers to penalty for misclassifying examples and sigma is a parameter of RBF which measures similarity between examples. To choose best model we use 5-fold cross-validation. We then evaluate our model on the entire data set.
gr = expand.grid(C = c(1, 50, 150), sigma = c(0.01, 0.05, 1))
tr = trainControl(method = 'cv', number = 5)
m2 = train(data = newData, Class ~ ., method = 'svmRadial', trControl = tr, tuneGrid = gr)
m2
As wee see, best tuning parameters are C = 50 and sigma = 0.05
Let's look at a confusion matrix
p2 = predict(m2, d[, -n])
confusionMatrix(p2, d[, n], positive = 'Yes')
(Numbers may differ due to randomness of k-fold cv)
As expected we were able to achieve sensitivity of 99.59%. In other words, out of all fraudulent transactions we correctly detected 99.59% of them. This came in price of slightly lower accuracy (in comparison to the first model) - 97.95% vs. 99.92% and lower specificity 97.94% vs. 99.98%. The main disadvantage is low level of positive predicted value (i.e. given that prediction is positive, what is probability that the true state is positive) which this case is 7.74% vs. 85% for initial (unbalanced dataset) model. As was mentioned in the beginning, one should choose a model that matches certain goals. If the goal is to correctly identify fraudulent transactions even in price of low positive predicted value (which I believe the case), then the latter model (based on SMOTed data) should be used. Looking at confusion matrix we see that almost all fraudulent transactions were correctly identified and only 2.5% were mislabeled as fraudulent.
I'm planning to try couple more models and also use more sophisticated algorithm that uses K-means centroids of the majority class as samples for non fraudulent transactions.
m3 = randomForest(data = newData, Class ~ .)
p3 = predict(m3, d[, -n])
confusionMatrix(p3, d[, n], positive = 'Yes')
library(randomForest)
m3 = randomForest(data = newData, Class ~ .)
p3 = predict(m3, d[, -n])
confusionMatrix(p3, d[, n], positive = 'Yes')
Random forest performs really well. Sensitivity 100% and high specificity (more than 99%). All fraudulent transactions were detected and less than 1% of all transactions were falsely classified as fraud. Hence, Random Forest + SMOTE algorithm shloud be considered as final model.
K-means centroids as a new sample
For curiosity, let's take another approach in dealing with imbalanced data. We are going to separate the examples for positive and negative and from the latter one extract centroids (generated using K-means clustering). Number of clusters will be equal to the number of positive examples. We then use these centroids together with positive examples as a new sample.(思路就是聚类,将major class聚类为k个点,其中k为欺诈信用卡的样本数!)
neg = d[d$Class == 'No', ] #negative examples
pos = d[d$Class == 'Yes', ] #positive examples
n_pos = sum(d$Class == 'Yes') #calculate number of positive examples
clus = kmeans(neg[, -n], centers = n_pos, iter.max = 100) #perform K-means
neg = as.data.frame(clus$centers) #extract centroids as new sample
neg$Class = 'No'
newData = rbind(neg, pos) #merge positive and negative examples
newData$Class = factor(newData$Class)
We run random forest on the new dataset, newData
, and check confusion matrix.
m4 = randomForest(data = newData, Class ~ .)
p4 = predict(m4, d[, -n])
confusionMatrix(p4, d[, n], positive = 'Yes')
Well, while sensitivity is still 100%, specificity dropped to 72% leading to a big fraction of false positive predictions. Learning on the data that was transformed using SMOTE algorithm gave much better results.
from:https://www.kaggle.com/themlguy/undersample-and-oversample-approach-explored
# This Python 3 environment comes with many helpful analytics libraries installed
# It is defined by the kaggle/python docker image: https://github.com/kaggle/docker-python
# For example, here's several helpful packages to load in
import numpy as np # linear algebra
import pandas as pd # data processing, CSV file I/O (e.g. pd.read_csv)
# Input data files are available in the "../input/" directory.
# For example, running this (by clicking run or pressing Shift+Enter) will list the files in the input directory
import os
print(os.listdir("../input"))
# Any results you write to the current directory are saved as output.
import matplotlib.pyplot as plt
from sklearn.cross_validation import train_test_split
from sklearn.linear_model import LogisticRegression
from sklearn.model_selection import GridSearchCV
from sklearn.svm import SVC
from sklearn.ensemble import RandomForestClassifier
from sklearn.preprocessing import StandardScaler
import seaborn as sns
from sklearn.metrics import confusion_matrix,recall_score,precision_recall_curve,auc,roc_curve,roc_auc_score,classification_report
creditcard_data=pd.read_csv("../input/creditcard.csv")
creditcard_data['Amount']=StandardScaler().fit_transform(creditcard_data['Amount'].values.reshape(-1, 1))
creditcard_data.drop(['Time'], axis=1, inplace=True)
def generatePerformanceReport(clf,X_train,y_train,X_test,y_test,bool_):
if bool_==True:
clf.fit(X_train,y_train.values.ravel())
pred=clf.predict(X_test)
cnf_matrix=confusion_matrix(y_test,pred)
tn, fp, fn, tp=cnf_matrix.ravel()
print('---------------------------------')
print('Length of training data:',len(X_train))
print('Length of test data:', len(X_test))
print('---------------------------------')
print('True positives:',tp)
print('True negatives:',tn)
print('False positives:',fp)
print('False negatives:',fn)
#sns.heatmap(cnf_matrix,cmap="coolwarm_r",annot=True,linewidths=0.5)
print('----------------------Classification report--------------------------')
print(classification_report(y_test,pred))
#generate 50%, 66%, 75% proportions of normal indices to be combined with fraud indices 也就是说采样后的黑白样本比例是:0.5,0.66,0.75
#undersampled data
normal_indices=creditcard_data[creditcard_data['Class']==0].index
fraud_indices=creditcard_data[creditcard_data['Class']==1].index
for i in range(1,4):
normal_sampled_data=np.array(np.random.choice(normal_indices, i*len(fraud_indices),replace=False)) #a random sample is generated from normal_indices 主要是随机欠采样
undersampled_data=np.concatenate([fraud_indices, normal_sampled_data])
undersampled_data=creditcard_data.iloc[undersampled_data]
print('length of undersampled data ', len(undersampled_data))
print('% of fraud transactions in undersampled data ',len(undersampled_data.loc[undersampled_data['Class']==1])/len(undersampled_data))
#get feature and label data
feature_data=undersampled_data.loc[:,undersampled_data.columns!='Class']
label_data=undersampled_data.loc[:,undersampled_data.columns=='Class']
X_train, X_test, y_train, y_test=train_test_split(feature_data,label_data,test_size=0.30)
for j in [LogisticRegression(),SVC(),RandomForestClassifier(n_estimators=100)]:
clf=j
print(j)
generatePerformanceReport(clf,X_train,y_train,X_test,y_test,True)
#the above code classifies X_test which is part of undersampled data
#now, let us consider the remaining rows of dataset and use that as test set
remaining_indices=[i for i in creditcard_data.index if i not in undersampled_data.index]
testdf=creditcard_data.iloc[remaining_indices]
testdf_label=creditcard_data.loc[:,testdf.columns=='Class']
testdf_feature=creditcard_data.loc[:,testdf.columns!='Class']
generatePerformanceReport(clf,X_train,y_train,testdf_feature,testdf_label,False)
#oversampled_data data
normal_sampled_indices=creditcard_data.loc[creditcard_data['Class']==0].index
oversampled_data=creditcard_data.iloc[normal_sampled_indices]
fraud_data=creditcard_data.loc[creditcard_data['Class']==1]
oversampled_data=oversampled_data.append([fraud_data]*300, ignore_index=True) #此处过采样处理是直接将欺诈样本复制300份!!!
print('length of oversampled_data data ', len(oversampled_data))
print('% of fraud transactions in oversampled_data data ',len(oversampled_data.loc[oversampled_data['Class']==1])/len(oversampled_data))
#get feature and label data
feature_data=oversampled_data.loc[:,oversampled_data.columns!='Class']
label_data=oversampled_data.loc[:,oversampled_data.columns=='Class']
X_train, X_test, y_train, y_test=train_test_split(feature_data,label_data,test_size=0.30)
for j in [LogisticRegression(),RandomForestClassifier(n_estimators=100)]:
clf=j
print(j)
generatePerformanceReport(clf,X_train,y_train,X_test,y_test,True)
#the above code classifies X_test which is part of undersampled data
#now, let us consider the remaining rows of dataset and use that as test set
remaining_indices=[i for i in creditcard_data.index if i not in oversampled_data.index]
testdf=creditcard_data.iloc[remaining_indices]
testdf_label=creditcard_data.loc[:,testdf.columns=='Class']
testdf_feature=creditcard_data.loc[:,testdf.columns!='Class']
generatePerformanceReport(clf,X_train,y_train,testdf_feature,testdf_label,False)
Random forest classifier with oversampled approach performs better compared to undersampled approach!!!
from:https://www.kaggle.com/gargmanish/how-to-handle-imbalance-data-study-in-detail
Hi all as we know credit card fraud detection will have a imbalanced data i.e having more number of normal class than the number of fraud class
In this I will use Basic method of handling imbalance data which are
This all I have done by using Analytics Vidya's blog please find the link Analytics Vidya
Undersampling:- it means taking the less number of majority class (In our case taking less number of Normal transactions so that our new data will be balanced
Oversampling: it means using replicating the data of minority class (fraud class) so that we can have a balanced data
SMOTE: it is also a type of oversampling but in this we will make the synthetic example of Minority data and will give as a balanced data
First I will start with the Undersampling and will try to classify using these Models
-
Decision Tree Classifier/ Random Forest Classifier
-
Logistic regression
-
SVM
-
XGboost
# This Python 3 environment comes with many helpful analytics libraries installed
# It is defined by the kaggle/python docker image: https://github.com/kaggle/docker-python
# For example, here's several helpful packages to load in
import numpy as np # linear algebra
import pandas as pd # data processing, CSV file I/O (e.g. pd.read_csv)
# Input data files are available in the "../input/" directory.
# For example, running this (by clicking run or pressing Shift+Enter) will list the files in the input directory
from subprocess import check_output
print(check_output(["ls", "../input"]).decode("utf8"))
# Any results you write to the current directory are saved as output.
Lets start with Importing Libraries and data
import pandas as pd # to import csv and for data manipulation
import matplotlib.pyplot as plt # to plot graph
import seaborn as sns # for intractve graphs
import numpy as np # for linear algebra
import datetime # to dela with date and time
%matplotlib inline
from sklearn.preprocessing import StandardScaler # for preprocessing the data
from sklearn.ensemble import RandomForestClassifier # Random forest classifier
from sklearn.tree import DecisionTreeClassifier # for Decision Tree classifier
from sklearn.svm import SVC # for SVM classification
from sklearn.linear_model import LogisticRegression
from sklearn.cross_validation import train_test_split # to split the data
from sklearn.cross_validation import KFold # For cross vbalidation
from sklearn.model_selection import GridSearchCV # for tunnig hyper parameter it will use all combination of given parameters
from sklearn.model_selection import RandomizedSearchCV # same for tunning hyper parameter but will use random combinations of parameters
from sklearn.metrics import confusion_matrix,recall_score,precision_recall_curve,auc,roc_curve,roc_auc_score,classification_report
import warnings
warnings.filterwarnings('ignore')
data = pd.read_csv("../input/creditcard.csv",header = 0)
Now explore the data to get insight in it
data.info()
- Hence we can see there are 284,807 rows and 31 columns which is a huge data
- Time is also in float here mean it can be only seconds starting from a particular time
# Now lets check the class distributions
sns.countplot("Class",data=data)
- As we know data is imbalanced and this graph also confirmed it
# now let us check in the number of Percentage
Count_Normal_transacation = len(data[data["Class"]==0]) # normal transaction are repersented by 0
Count_Fraud_transacation = len(data[data["Class"]==1]) # fraud by 1
Percentage_of_Normal_transacation = Count_Normal_transacation/(Count_Normal_transacation+Count_Fraud_transacation)
print("percentage of normal transacation is",Percentage_of_Normal_transacation*100)
Percentage_of_Fraud_transacation= Count_Fraud_transacation/(Count_Normal_transacation+Count_Fraud_transacation)
print("percentage of fraud transacation",Percentage_of_Fraud_transacation*100)
- Hence in data there is only 0.17 % are the fraud transcation while 99.83 are valid transcation
- So now we have to do resampling of this data
- before doing resampling lets have look at the amount related to valid transcation and fraud transcation
Fraud_transacation = data[data["Class"]==1]
Normal_transacation= data[data["Class"]==0]
plt.figure(figsize=(10,6))
plt.subplot(121)
Fraud_transacation.Amount.plot.hist(title="Fraud Transacation")
plt.subplot(122)
Normal_transacation.Amount.plot.hist(title="Normal Transaction")
# the distribution for Normal transction is not clear and it seams that all transaction are less than 2.5 K
# So plot graph for same
Fraud_transacation = data[data["Class"]==1]
Normal_transacation= data[data["Class"]==0]
plt.figure(figsize=(10,6))
plt.subplot(121)
Fraud_transacation[Fraud_transacation["Amount"]<= 2500].Amount.plot.hist(title="Fraud Tranascation")
plt.subplot(122)
Normal_transacation[Normal_transacation["Amount"]<=2500].Amount.plot.hist(title="Normal Transaction")
- Here now after exploring data we can say there is no pattern in data
- Now lets start with resmapling of data
ReSampling - Under Sampling
Before re sampling lets have look at the different accuracy matrices
Accuracy = TP+TN/Total
Precison = TP/(TP+FP)
Recall = TP/(TP+FN)
TP = True possitive means no of possitve cases which are predicted possitive
TN = True negative means no of negative cases which are predicted negative
FP = False possitve means no of negative cases which are predicted possitive
FN= False Negative means no of possitive cases which are predicted negative
Now for our case recall will be a better option because in these case no of normal transacations will be very high than the no of fraud cases and sometime a fraud case will be predicted as normal. So, recall will give us a sense of only fraud cases
Resampling
in this we will resample our data with different size
then we will try to use this resampled data to train our model
then we will use this model to predict for our original data
# for undersampling we need a portion of majority class and will take whole data of minority class
# count fraud transaction is the total number of fraud transaction
# now lets us see the index of fraud cases
fraud_indices= np.array(data[data.Class==1].index)
normal_indices = np.array(data[data.Class==0].index)
#now let us a define a function for make undersample data with different proportion
#different proportion means with different proportion of normal classes of data
def undersample(normal_indices,fraud_indices,times):#times denote the normal data = times*fraud data
Normal_indices_undersample = np.array(np.random.choice(normal_indices,(times*Count_Fraud_transacation),replace=False)) #和上面例子是一样的!!!
undersample_data= np.concatenate([fraud_indices,Normal_indices_undersample])
undersample_data = data.iloc[undersample_data,:]
print("the normal transacation proportion is :",len(undersample_data[undersample_data.Class==0])/len(undersample_data[undersample_data.Class]))
print("the fraud transacation proportion is :",len(undersample_data[undersample_data.Class==1])/len(undersample_data[undersample_data.Class]))
print("total number of record in resampled data is:",len(undersample_data[undersample_data.Class]))
return(undersample_data)
## first make a model function for modeling with confusion matrix
def model(model,features_train,features_test,labels_train,labels_test):
clf= model
clf.fit(features_train,labels_train.values.ravel())
pred=clf.predict(features_test)
cnf_matrix=confusion_matrix(labels_test,pred)
print("the recall for this model is :",cnf_matrix[1,1]/(cnf_matrix[1,1]+cnf_matrix[1,0]))
fig= plt.figure(figsize=(6,3))# to plot the graph
print("TP",cnf_matrix[1,1,]) # no of fraud transaction which are predicted fraud
print("TN",cnf_matrix[0,0]) # no. of normal transaction which are predited normal
print("FP",cnf_matrix[0,1]) # no of normal transaction which are predicted fraud
print("FN",cnf_matrix[1,0]) # no of fraud Transaction which are predicted normal
sns.heatmap(cnf_matrix,cmap="coolwarm_r",annot=True,linewidths=0.5)
plt.title("Confusion_matrix")
plt.xlabel("Predicted_class")
plt.ylabel("Real class")
plt.show()
print("
----------Classification Report------------------------------------")
print(classification_report(labels_test,pred))
def data_prepration(x): # preparing data for training and testing as we are going to use different data
#again and again so make a function
x_features= x.ix[:,x.columns != "Class"]
x_labels=x.ix[:,x.columns=="Class"]
x_features_train,x_features_test,x_labels_train,x_labels_test = train_test_split(x_features,x_labels,test_size=0.3) #30%用于测试
print("length of training data")
print(len(x_features_train))
print("length of test data")
print(len(x_features_test))
return(x_features_train,x_features_test,x_labels_train,x_labels_test)
# before starting we should standridze our ampount column
data["Normalized Amount"] = StandardScaler().fit_transform(data['Amount'].reshape(-1, 1))
data.drop(["Time","Amount"],axis=1,inplace=True)
data.head()
Logistic Regression with Undersample Data
# Now make undersample data with differnt portion
# here i will take normal trasaction in 0..5 %, 0.66% and 0.75 % proportion of total data now do this for
for i in range(1,4):
print("the undersample data for {} proportion".format(i))
print()
Undersample_data = undersample(normal_indices,fraud_indices,i)
print("------------------------------------------------------------")
print()
print("the model classification for {} proportion".format(i))
print()
undersample_features_train,undersample_features_test,undersample_labels_train,undersample_labels_test=data_prepration(Undersample_data)
print()
clf=LogisticRegression()
model(clf,undersample_features_train,undersample_features_test,undersample_labels_train,undersample_labels_test)
print("________________________________________________________________________________________________________")
# here 1st proportion conatain 50% normal transaction
#Proportion 2nd contains 66% noraml transaction
#proportion 3rd contains 75 % normal transaction
- As the number of normal transaction is increasing the recall for fraud transcation is decreasing
- TP = no of fraud transaction which are predicted fraud
- TN = no. of normal transaction which are predicted normal
- FP = no of normal transaction which are predicted fraud
- FN =no of fraud Transaction which are predicted normal
#let us train this model using undersample data and test for the whole data test set #用欠采样训练的模型来预测全量数据集
for i in range(1,4):
print("the undersample data for {} proportion".format(i))
print()
Undersample_data = undersample(normal_indices,fraud_indices,i)
print("------------------------------------------------------------")
print()
print("the model classification for {} proportion".format(i))
print()
undersample_features_train,undersample_features_test,undersample_labels_train,undersample_labels_test=data_prepration(Undersample_data)
data_features_train,data_features_test,data_labels_train,data_labels_test=data_prepration(data)
#the partion for whole data
print()
clf=LogisticRegression()
model(clf,undersample_features_train,data_features_test,undersample_labels_train,data_labels_test)
# here training for the undersample data but tatsing for whole data
print("_________________________________________________________________________________________")
-
Here we can see it is following same recall pattern as it was for under sample data that's sounds good but if we have look at the precision is very less
-
So we should built a model which is correct overall
-
Precision is less means we are predicting other class wrong like as for our third part there were 953 transaction are predicted fraud it means we and recall is good then it means we are catching fraud transaction very well but we are catching innocent transaction also i.e which are not fraud.
-
So with recall our precision should be better
-
if we go by this model then we are going to put 953 innocents in jail with the all criminal who have actually done this
- Hence we are mainly lacking in the precision how can we increase our precision
- Don't get confuse with above output showing that the two training data and two test data first one is for undersample data while another one is for our whole data
1.Try with SVM and then Random Forest in same Manner
- from Random forest we can get which features are more important
SVM with Undersample data
for i in range(1,4):
print("the undersample data for {} proportion".format(i))
print()
Undersample_data = undersample(normal_indices,fraud_indices,i)
print("------------------------------------------------------------")
print()
print("the model classification for {} proportion".format(i))
print()
undersample_features_train,undersample_features_test,undersample_labels_train,undersample_labels_test=data_prepration(Undersample_data)
print()
clf= SVC()# here we are just changing classifier
model(clf,undersample_features_train,undersample_features_test,undersample_labels_train,undersample_labels_test)
print("________________________________________________________________________________________________________")
-
Here recall and precision are approximately equal to Logistic Regression
-
Lets try for whole data
#let us train this model using undersample data and test for the whole data test set
for i in range(1,4):
print("the undersample data for {} proportion".format(i))
print()
Undersample_data = undersample(normal_indices,fraud_indices,i)
print("------------------------------------------------------------")
print()
print("the model classification for {} proportion".format(i))
print()
undersample_features_train,undersample_features_test,undersample_labels_train,undersample_labels_test=data_prepration(Undersample_data)
data_features_train,data_features_test,data_labels_train,data_labels_test=data_prepration(data)
#the partion for whole data
print()
clf=SVC()
model(clf,undersample_features_train,data_features_test,undersample_labels_train,data_labels_test)
# here training for the undersample data but tatsing for whole data
print("_________________________________________________________________________________________")
- A better recall but precision is not improving much
2 .so to improve precision we must have to tune the hyper parameter of these models
3 That I will do in next version
4 For now lets try with my favorite Random Forest classifier
# Random Forest Classifier with undersample data only
for i in range(1,4):
print("the undersample data for {} proportion".format(i))
print()
Undersample_data = undersample(normal_indices,fraud_indices,i)
print("------------------------------------------------------------")
print()
print("the model classification for {} proportion".format(i))
print()
undersample_features_train,undersample_features_test,undersample_labels_train,undersample_labels_test=data_prepration(Undersample_data)
print()
clf= RandomForestClassifier(n_estimators=100)# here we are just changing classifier
model(clf,undersample_features_train,undersample_features_test,undersample_labels_train,undersample_labels_test)
print("________________________________________________________________________________________________________")
#let us train this model using undersample data and test for the whole data test set
for i in range(1,4):
print("the undersample data for {} proportion".format(i))
print()
Undersample_data = undersample(normal_indices,fraud_indices,i)
print("------------------------------------------------------------")
print()
print("the model classification for {} proportion".format(i))
print()
undersample_features_train,undersample_features_test,undersample_labels_train,undersample_labels_test=data_prepration(Undersample_data)
data_features_train,data_features_test,data_labels_train,data_labels_test=data_prepration(data)
#the partion for whole data
print()
clf=RandomForestClassifier(n_estimators=100)
model(clf,undersample_features_train,data_features_test,undersample_labels_train,data_labels_test)
# here training for the undersample data but tatsing for whole data
print("_________________________________________________________________________________________")
-
for the third proportion the precision is 0.33 which is better than others
-
Lets try to get only import features using Random Forest Classifier
-
After it i will do analysis only for one portion that is 0.5 %
featimp = pd.Series(clf.feature_importances_,index=data_features_train.columns).sort_values(ascending=False)
print(featimp) # this is the property of Random Forest classifier that it provide us the importance
# of the features use
-
we can see this is showing the importance of feature for the making decision
-
V14 is having a very good importance compare to other features
-
Lets use only top 5 (V14,V10,V12,V17,V4) feature to predict using Random forest classifier only for 0.5 % 特征选择使用top 5特征
# make a new data with only class and V14
data1=data[["V14","V10","V12","V17","V4","Class"]]
data1.head()
Undersample_data1 = undersample(normal_indices,fraud_indices,1)
#only for 50 % proportion it means normal transaction and fraud transaction are equal so passing
Undersample_data1_features_train,Undersample_data1_features_test,Undersample_data1_labels_train,Undersample_data1_labels_test = data_prepration(Undersample_data1)
clf= RandomForestClassifier(n_estimators=100)
model(clf,Undersample_data1_features_train,Undersample_data1_features_test,Undersample_data1_labels_train,Undersample_data1_labels_test)
Over Sampling
-
In my previous version I got the 100 recall and 98 % precision by using Random forest with the over sampled data but in real it was due to over fitting because i was taking whole fraud data and was training for that and I was doing the testing on the same data.
-
Please find link of previous version for more understanding Link
- Thanks to Mr. Dominik Stuerzer for help
# now we will divied our data sets into two part and we will train and test and will oversample the train data and predict for test data
# lets import data again
data = pd.read_csv("../input/creditcard.csv",header = 0)
print("length of training data",len(data))
print("length of normal data",len(data[data["Class"]==0]))
print("length of fraud data",len(data[data["Class"]==1]))
data_train_X,data_test_X,data_train_y,data_test_y=data_prepration(data)
data_train_X.columns
data_train_y.columns
# ok Now we have a traing data
data_train_X["Class"]= data_train_y["Class"] # combining class with original data
data_train = data_train_X.copy() # for naming conevntion
print("length of training data",len(data_train))
# Now make data set of normal transction from train data
normal_data = data_train[data_train["Class"]==0]
print("length of normal data",len(normal_data))
fraud_data = data_train[data_train["Class"]==1]
print("length of fraud data",len(fraud_data))
# Now start oversamoling of training data
# means we will duplicate many times the value of fraud data #直接复制365份!!!
for i in range (365): # the number is choosen by myself on basis of nnumber of fraud transaction
normal_data= normal_data.append(fraud_data)
os_data = normal_data.copy()
print("length of oversampled data is ",len(os_data))
print("Number of normal transcation in oversampled data",len(os_data[os_data["Class"]==0]))
print("No.of fraud transcation",len(os_data[os_data["Class"]==1]))
print("Proportion of Normal data in oversampled data is ",len(os_data[os_data["Class"]==0])/len(os_data))
print("Proportion of fraud data in oversampled data is ",len(os_data[os_data["Class"]==1])/len(os_data))
- The proportion now becomes the 60 % and 40 % that is good now
# before applying any model standerdize our data amount
os_data["Normalized Amount"] = StandardScaler().fit_transform(os_data['Amount'].reshape(-1, 1))
os_data.drop(["Time","Amount"],axis=1,inplace=True) 其实随机森林对特征是否标准化无感,但是svm和LR就非常非常关键了
os_data.head()
# Now use this oversampled data for trainig the model and predict value for the test data that we created before
# now let us try within the the oversampled data itself
# for that we need to split our oversampled data into train and test
# so call our function data Prepration with oversampled data
os_train_X,os_test_X,os_train_y,os_test_y=data_prepration(os_data)
clf= RandomForestClassifier(n_estimators=100)
model(clf,os_train_X,os_test_X,os_train_y,os_test_y)
Observations
- As it have too many sample of same fraud data so may be the all which are present in train data are present in test data also so we can say it is over fitting #重复样本太多,过拟合严重
- So lets try with test data that one which we created in starting of oversampling segment no fraud transaction from that data have been repeated here #在过采样前先拿出一点数据出来做测试,而不是过采样之后!!!
- Lets try
# now take all over sampled data as trainging and test it for test data
os_data_X = os_data.ix[:,os_data.columns != "Class"]
os_data_y = os_data.ix[:,os_data.columns == "Class"]
#for that we have to standrdize the normal amount and drop the time from it
data_test_X["Normalized Amount"] = StandardScaler().fit_transform(data_test_X['Amount'].reshape(-1, 1))
data_test_X.drop(["Time","Amount"],axis=1,inplace=True)
data_test_X.head()
# now use it for modeling
clf= RandomForestClassifier(n_estimators=100)
model(clf,os_data_X,data_test_X,os_data_y,data_test_y)
Observations
- Now here we can see recall decrease to only 83 % which is not bad but not good also
- The precision is 0.93 which is good
- from these observation we can say that the oversampling is better than the Under sampling because on Under sampling we were loosing a large amount of data or we can say a good amount of information so why the there precision was very low
SMOTE
# Lets Use SMOTE for Sampling
# As I mentioned it is also a type of oversampling but in this the data is not replicated but they are created
#lets start with importing libraries
from imblearn.over_sampling import SMOTE
data = pd.read_csv('../input/creditcard.csv')
os = SMOTE(random_state=0) # We are using SMOTE as the function for oversampling
# now we can devided our data into training and test data
# Call our method data prepration on our dataset
data_train_X,data_test_X,data_train_y,data_test_y=data_prepration(data)
columns = data_train_X.columns
# now use SMOTE to oversample our train data which have features data_train_X and labels in data_train_y
os_data_X,os_data_y=os.fit_sample(data_train_X,data_train_y)
os_data_X = pd.DataFrame(data=os_data_X,columns=columns )
os_data_y= pd.DataFrame(data=os_data_y,columns=["Class"])
# we can Check the numbers of our data
print("length of oversampled data is ",len(os_data_X))
print("Number of normal transcation in oversampled data",len(os_data_y[os_data_y["Class"]==0]))
print("No.of fraud transcation",len(os_data_y[os_data_y["Class"]==1]))
print("Proportion of Normal data in oversampled data is ",len(os_data_y[os_data_y["Class"]==0])/len(os_data_X))
print("Proportion of fraud data in oversampled data is ",len(os_data_y[os_data_y["Class"]==1])/len(os_data_X))
-
By using Smote we are getting a 50 - 50 each
-
No need of checking here in over sampled data itself from previous we know it will be overfitting
-
let us check with the test data direct
# Let us first do our amount normalised and other that we are doing above #过采样前一定一定要标准化!!!
os_data_X["Normalized Amount"] = StandardScaler().fit_transform(os_data_X['Amount'].reshape(-1, 1))
os_data_X.drop(["Time","Amount"],axis=1,inplace=True)
data_test_X["Normalized Amount"] = StandardScaler().fit_transform(data_test_X['Amount'].reshape(-1, 1))
data_test_X.drop(["Time","Amount"],axis=1,inplace=True)
# Now start modeling
clf= RandomForestClassifier(n_estimators=100)
# train data using oversampled data and predict for the test data
model(clf,os_data_X,data_test_X,os_data_y,data_test_y)
observation
- The recall is nearby the previous one done by over sampling
- The precision decrease in this case
综合结论就是:随机森林+过采样(直接复制或者smote后,黑白比例1:3)效果比较好!
from:http://www.dataguru.cn/article-11449-1.html
用Python作信用卡欺诈预测 ——欠采样、效果不好
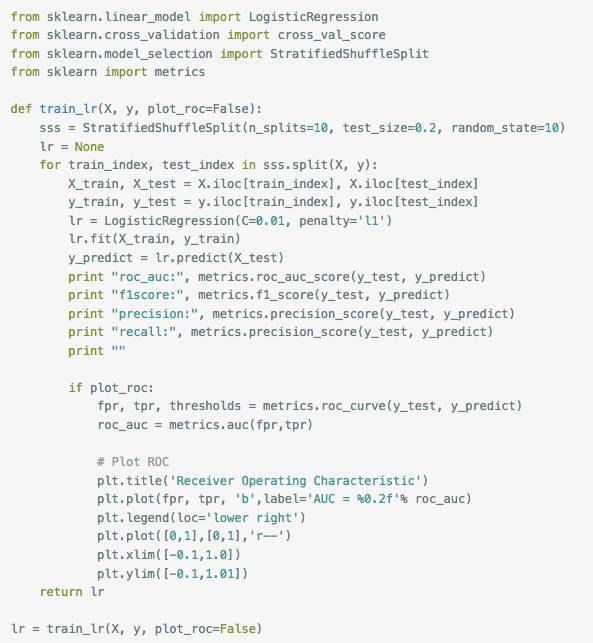
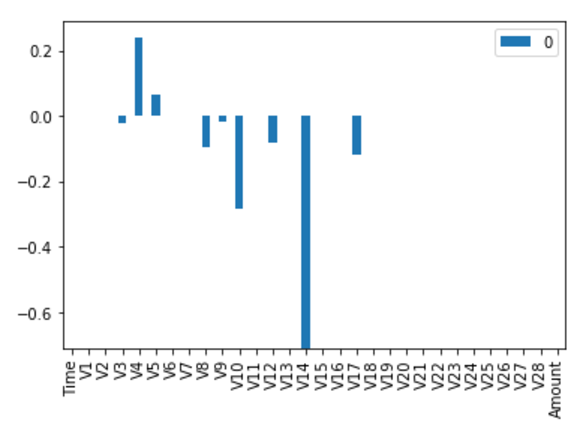
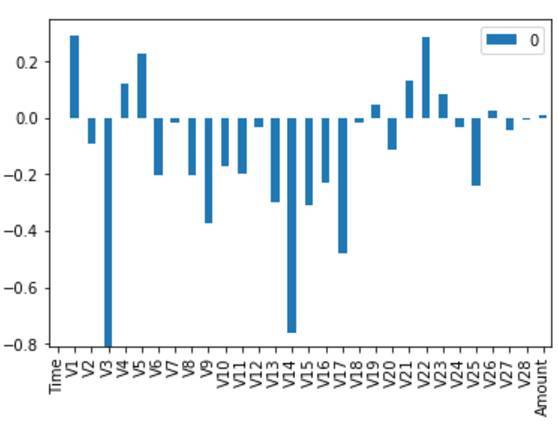
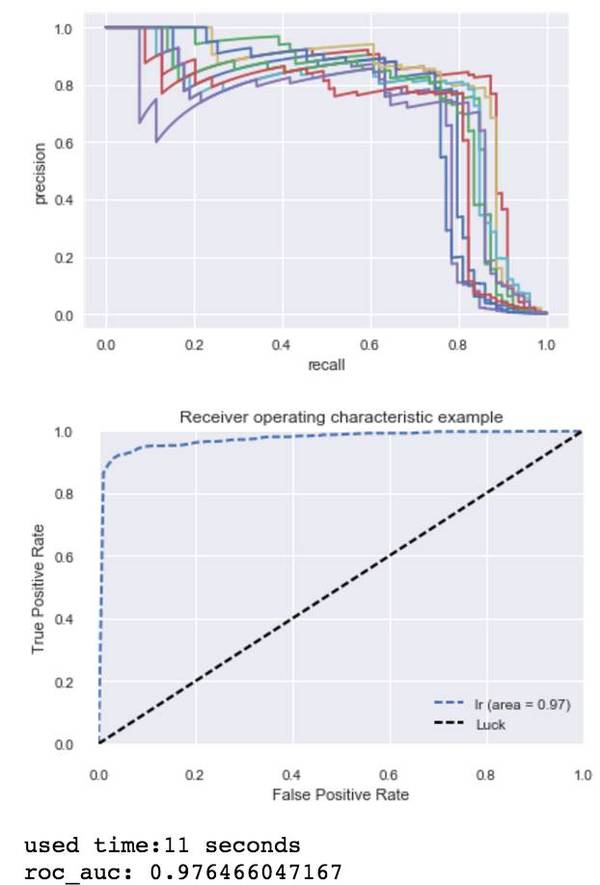
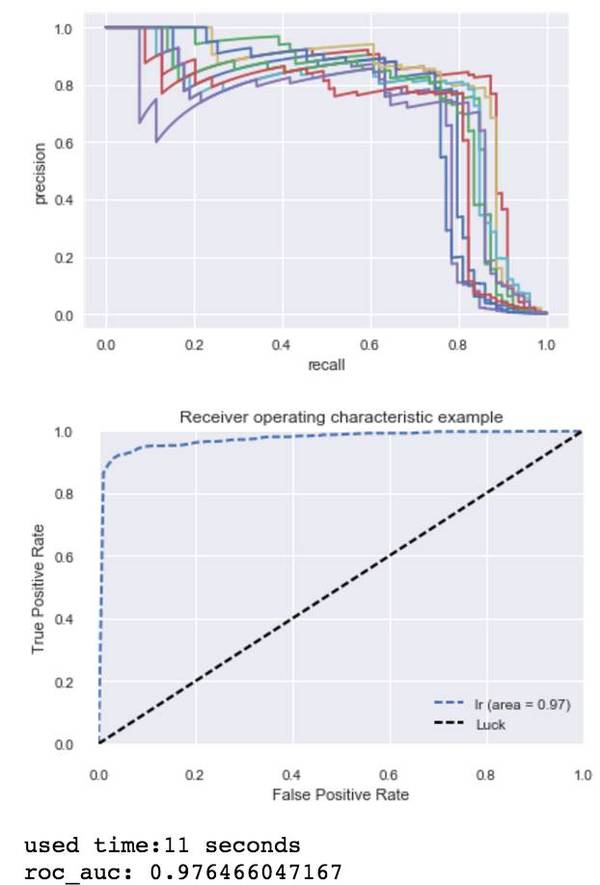
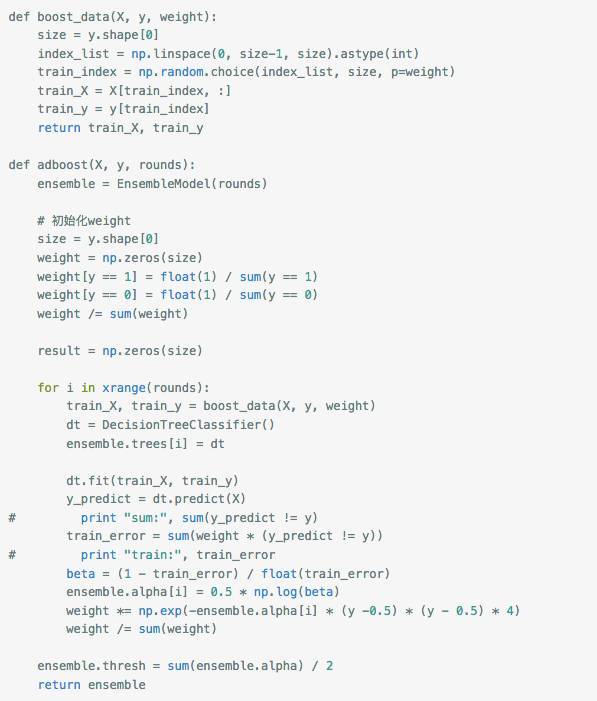
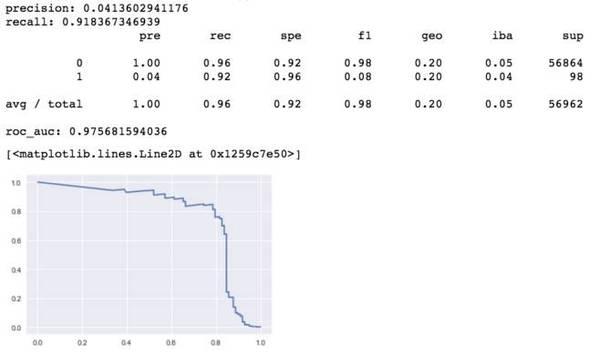
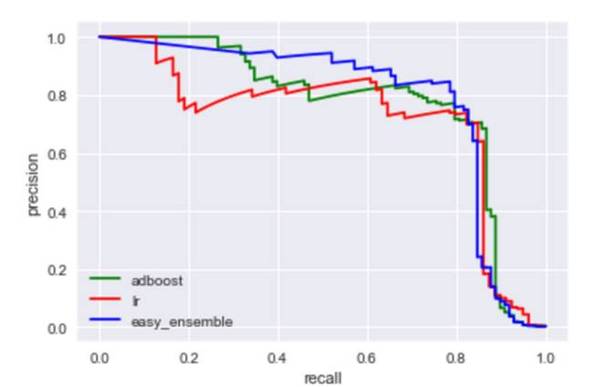
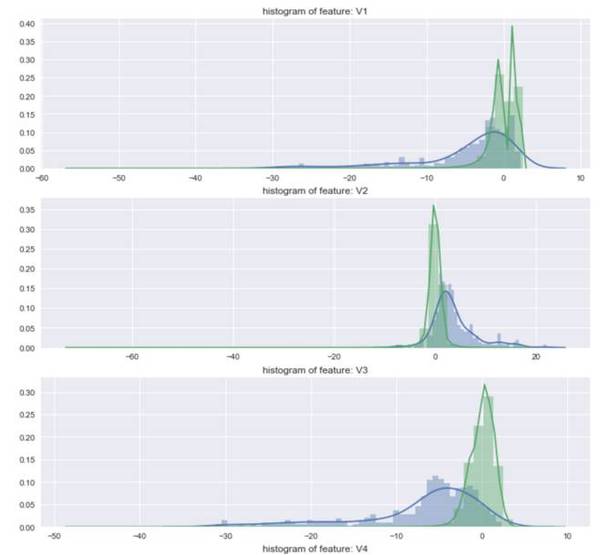
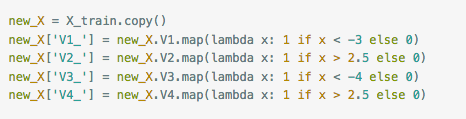
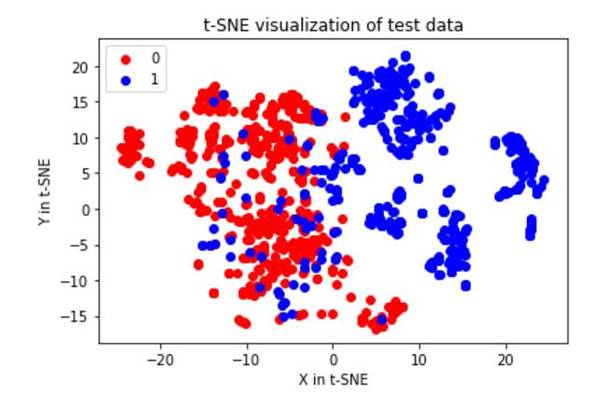