sum_weights 可以通过参数设置。 如果不设置,那么值就是样本的个数。 指定每个样本的权重。
我突然想到基金预测,可以设置样本的权重。 真实涨幅越高,权重越小。 反之,权重越高。 因为如果预测偏低,那么loss 损失越大。
"rmse":
sum_loss = 和 (score - label)*(score - label)
loss = std::sqrt(sum_loss / sum_weights);
"l2": 误差的平方:
sum_loss = 和 (score - label)*(score - label)
loss = sum_loss / sum_weights
"l1": 误差的绝对值:
sum_loss = 和 fabs(score - label)
loss = sum_loss / sum_weights
"quantile": 分位数损失
inline static double LossOnPoint(label_t label, double score, const Config& config) { double delta = label - score; if (delta < 0) { return (config.alpha - 1.0f) * delta; } else { return config.alpha * delta; } }
从c++ 代码可以看到, 如果alpha为0.5, 那么就是误差的绝对值* 0.5. 如果为0.2。 那么就是负误差越小,损失越大。 从图中可以看出,alpha值越大,正误差损失斜率越低。 为0.5时正负斜率相等
sum_loss = 和 LossOnPoint(label, score)
loss = sum_loss / sum_weights
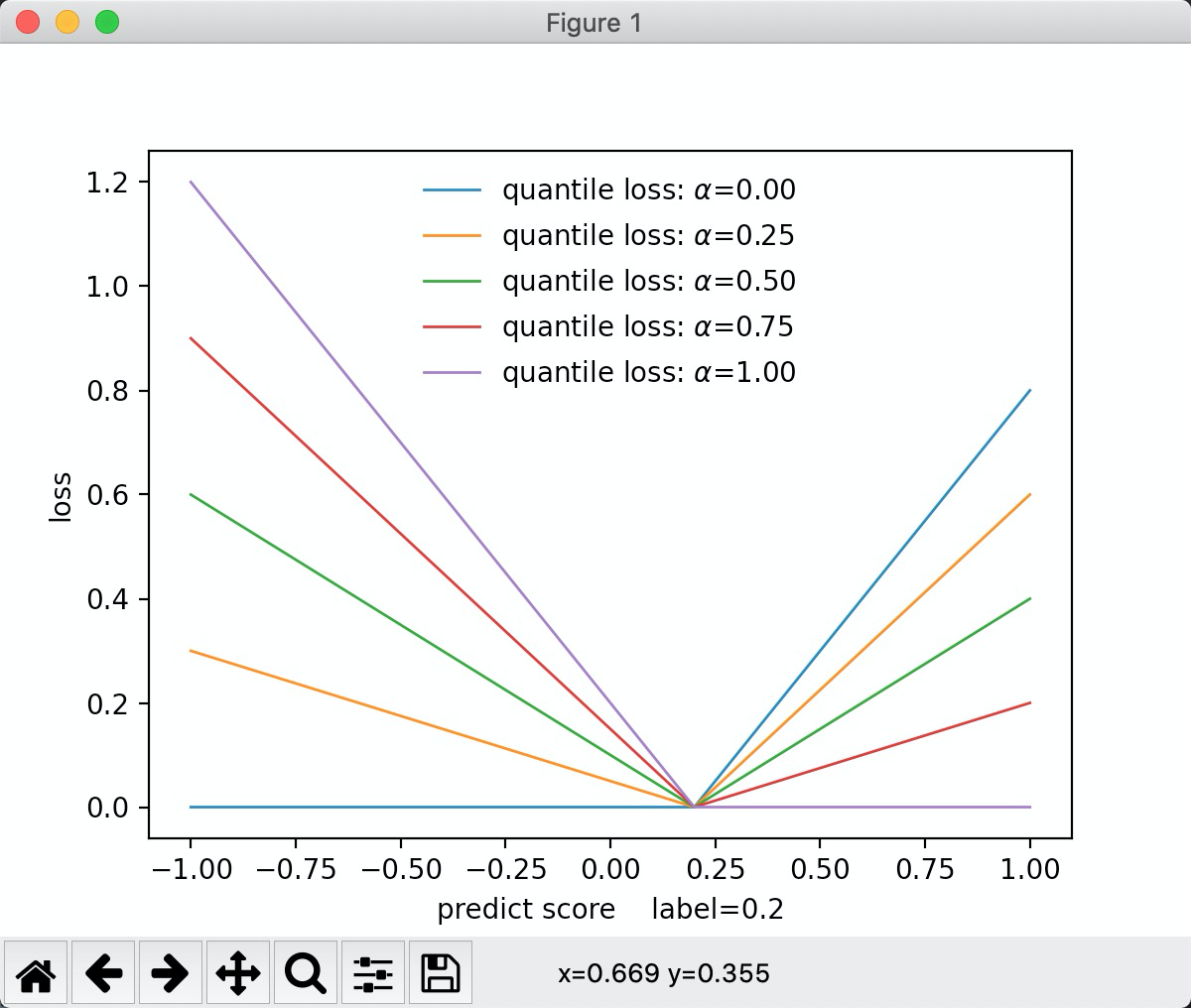
"huber":
inline static double LossOnPoint(label_t label, double score, const Config& config) { const double diff = score - label; if (std::abs(diff) <= config.alpha) { return 0.5f * diff * diff; } else { return config.alpha * (std::abs(diff) - 0.5f * config.alpha); } }
预测的分数离标签值越近,越趋近0. 从图中可以看出, 就是平方损失函数的压缩版。 huber损失值相对平方损失压小了。 alpha越小, Huber损失值压的越狠。
"fair":公允价值变动损失
inline static double LossOnPoint(label_t label, double score, const Config& config) { const double x = std::fabs(score - label); const double c = config.fair_c; return c * x - c * c * std::log(1.0f + x / c); }
从图中可以看出, fair_c 越小, 损失越小。 fair_c 越大损失越大
"poisson": 柏松回归
inline static double LossOnPoint(label_t label, double score, const Config&) { const double eps = 1e-10f; if (score < eps) { score = eps; } return score - label * std::log(score); }
如果出现负误差, 那么损失值非常大。
mape:
inline static double LossOnPoint(label_t label, double score, const Config&) { return std::fabs((label - score)) / std::max(1.0f, std::fabs(label)); }
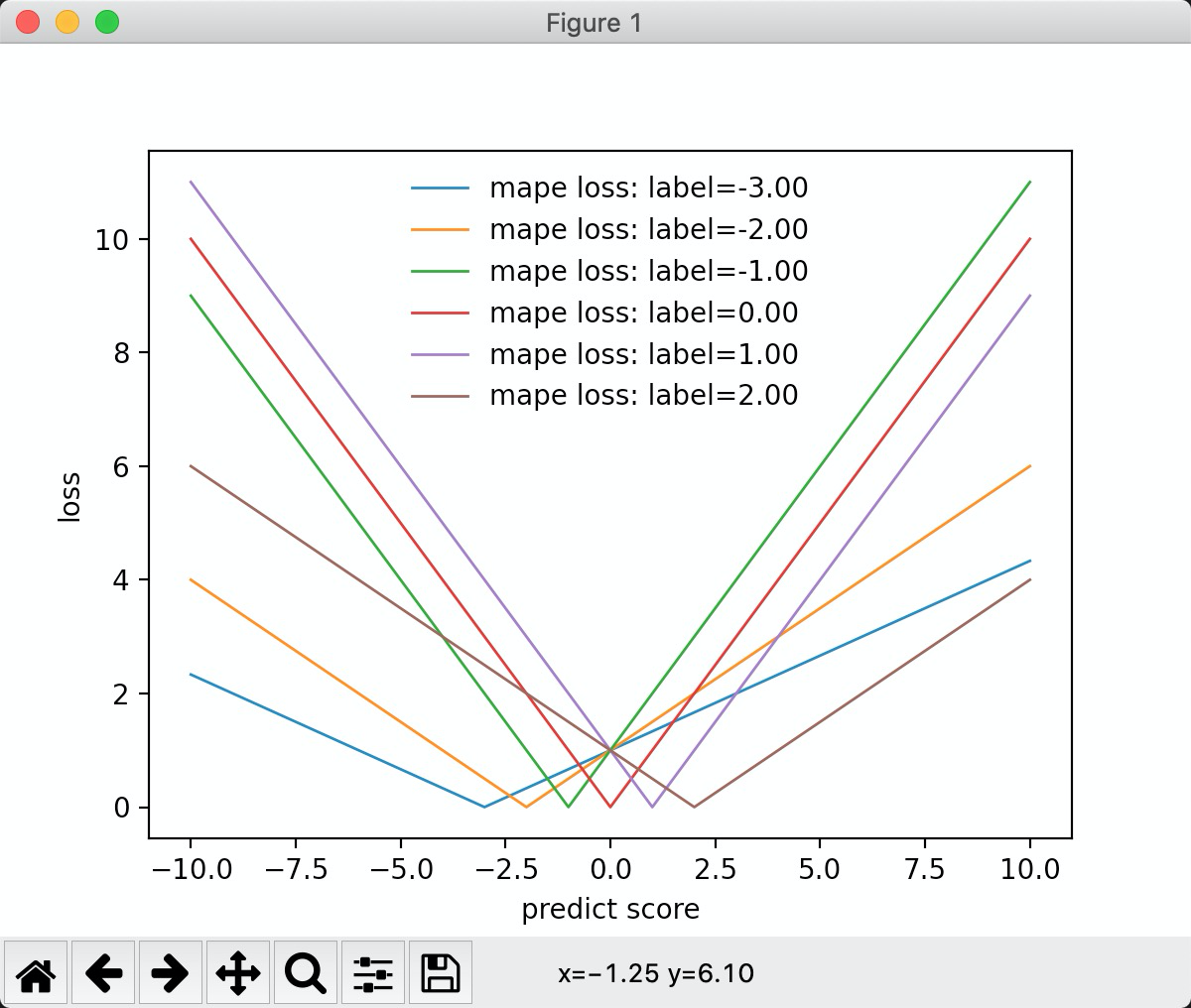
gamma: 分布
inline static double LossOnPoint(label_t label, double score, const Config&) { const double psi = 1.0; const double theta = -1.0 / score; const double a = psi; const double b = -Common::SafeLog(-theta); const double c = 1. / psi * Common::SafeLog(label / psi) - Common::SafeLog(label) - 0; // 0 = std::lgamma(1.0 / psi) = std::lgamma(1.0); return -((label * theta - b) / a + c); }
限制条件: label 必须大于0
从图中可以看到, 标签值越大, 预测值离标签值越近,损失值越小。 在预测值在标签值附近的时候,损失值差别不大
"gamma_deviance":
inline static double LossOnPoint(label_t label, double score, const Config&) { const double epsilon = 1.0e-9; const double tmp = label / (score + epsilon); return tmp - Common::SafeLog(tmp) - 1; }
从图中可以看出, 当标签为1的时候,损失值是线性的, 标签值大于0的时候。 标签为2的时候,是分段线性的。 负误差的斜率和正误差的斜率要陡。
"tweedie" 类分布
inline static double LossOnPoint(label_t label, double score, const Config& config) { const double rho = config.tweedie_variance_power; const double eps = 1e-10f; if (score < eps) { score = eps; } const double a = label * std::exp((1 - rho) * std::log(score)) / (1 - rho); const double b = std::exp((2 - rho) * std::log(score)) / (2 - rho); return -a + b; }
tweedie_variance_power = 0.2 时, 函数图形如下: