# load MNIST data
import input_data
mnist = input_data.read_data_sets("Mnist_data/", one_hot=True)
# start tensorflow interactiveSession
import tensorflow as tf
sess = tf.InteractiveSession()
# weight initialization
def weight_variable(shape):
initial = tf.truncated_normal(shape, stddev=0.1)
return tf.Variable(initial)
def bias_variable(shape):
initial = tf.constant(0.1, shape = shape)
return tf.Variable(initial)
# convolution
def conv2d(x, W):
return tf.nn.conv2d(x, W, strides=[1, 1, 1, 1], padding='SAME')
# pooling
def max_pool_2x2(x):
return tf.nn.max_pool(x, ksize=[1, 2, 2, 1], strides=[1, 2, 2, 1], padding='SAME')
# Create the model
# placeholder
x = tf.placeholder("float", [None, 784])
y_ = tf.placeholder("float", [None, 10])
# variables
W = tf.Variable(tf.zeros([784,10]))
b = tf.Variable(tf.zeros([10]))
y = tf.nn.softmax(tf.matmul(x,W) + b)
# first convolutinal layer
w_conv1 = weight_variable([5, 5, 1, 32])
b_conv1 = bias_variable([32])
x_image = tf.reshape(x, [-1, 28, 28, 1])
h_conv1 = tf.nn.relu(conv2d(x_image, w_conv1) + b_conv1)
h_pool1 = max_pool_2x2(h_conv1)
# second convolutional layer
w_conv2 = weight_variable([5, 5, 32, 64])
b_conv2 = bias_variable([64])
h_conv2 = tf.nn.relu(conv2d(h_pool1, w_conv2) + b_conv2)
h_pool2 = max_pool_2x2(h_conv2)
# densely connected layer
w_fc1 = weight_variable([7*7*64, 1024])
b_fc1 = bias_variable([1024])
h_pool2_flat = tf.reshape(h_pool2, [-1, 7*7*64])
h_fc1 = tf.nn.relu(tf.matmul(h_pool2_flat, w_fc1) + b_fc1)
# dropout
keep_prob = tf.placeholder("float")
h_fc1_drop = tf.nn.dropout(h_fc1, keep_prob)
# readout layer
w_fc2 = weight_variable([1024, 10])
b_fc2 = bias_variable([10])
y_conv = tf.nn.softmax(tf.matmul(h_fc1_drop, w_fc2) + b_fc2)
# train and evaluate the model
cross_entropy = -tf.reduce_sum(y_*tf.log(y_conv))
train_step = tf.train.AdagradOptimizer(1e-4).minimize(cross_entropy)
correct_prediction = tf.equal(tf.argmax(y_conv, 1), tf.argmax(y_, 1))
accuracy = tf.reduce_mean(tf.cast(correct_prediction, "float"))
sess.run(tf.initialize_all_variables())
for i in range(20000):
batch = mnist.train.next_batch(50)
if i%100 == 0:
train_accuracy = accuracy.eval(feed_dict={x:batch[0], y_:batch[1], keep_prob:1.0})
print "step %d, train accuracy %g" %(i, train_accuracy)
train_step.run(feed_dict={x:batch[0], y_:batch[1], keep_prob:0.5})
print "test accuracy %g" % accuracy.eval(feed_dict={x:mnist.test.images, y_:mnist.test.labels, keep_prob:1.0})
运行结果:未完成20000步的过程截图
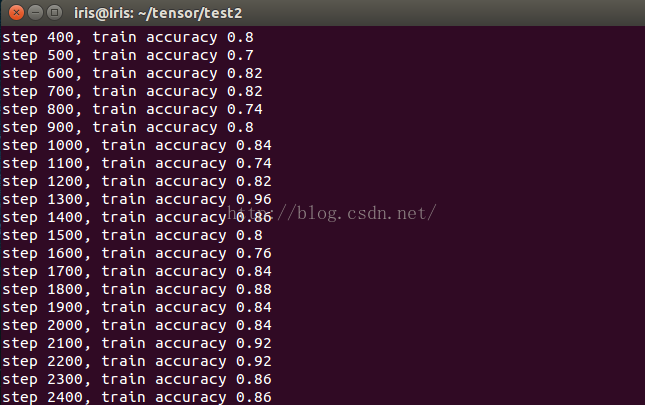