一、多车辆识别可能和车辆车牌分割;
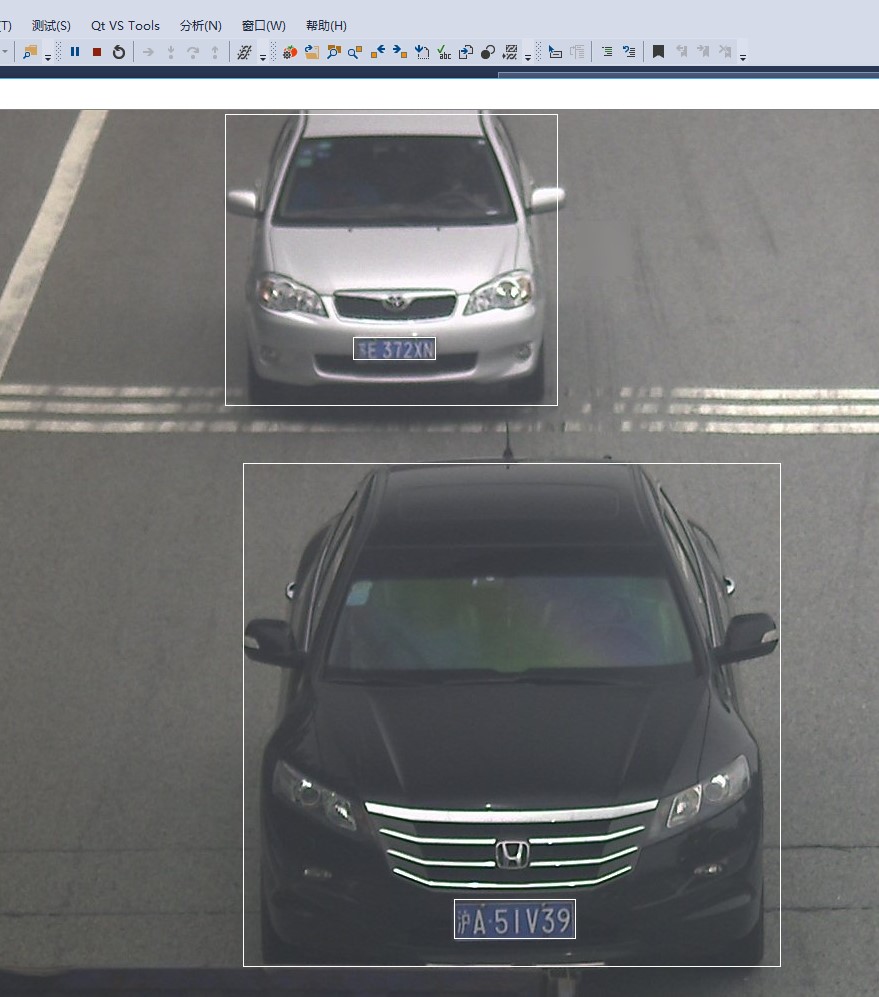
这样一张图,可以识别多车辆和车牌,问题是如何区分并且配对。
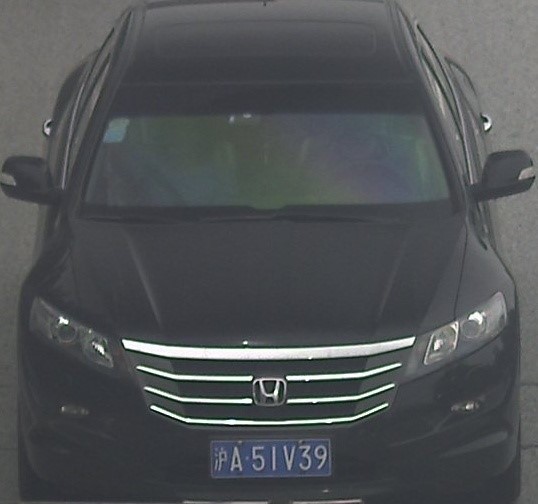
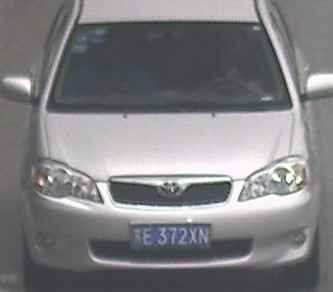
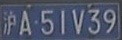

是否是车牌可以通过图片的大小进行判断。而配对是前后顺序的。
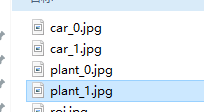
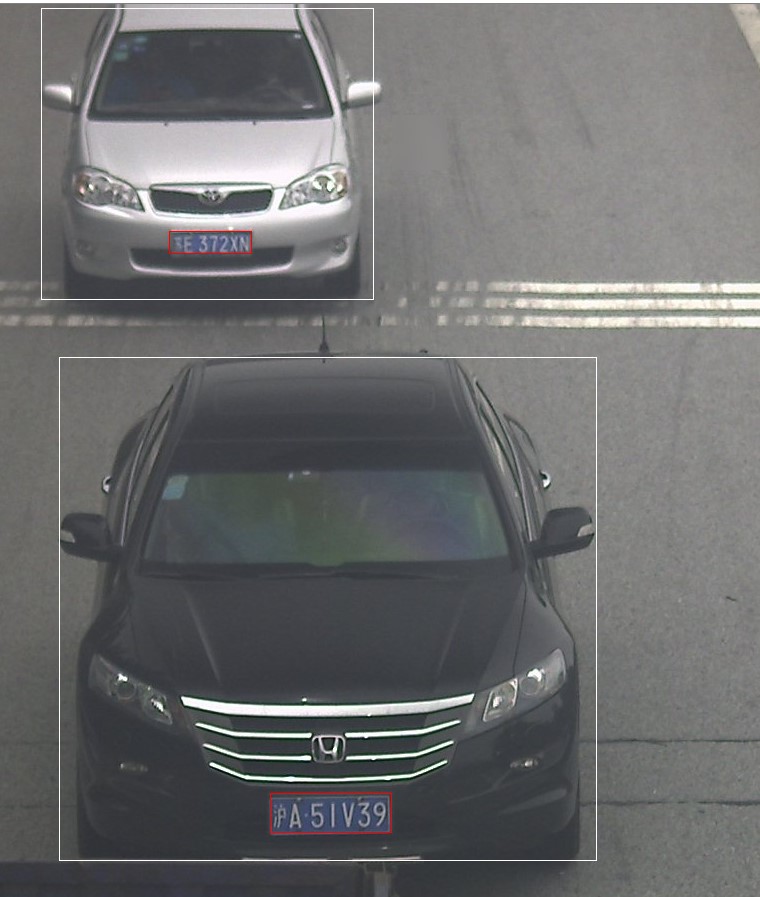
// --------------------------- 8. 处理结果-------------------------------------------------------
const float *detections = infer_request.GetBlob(firstOutputName)->buffer().as<float *>();
int i_car = 0;
int i_plate = 0;
for (int i = 0; i < 200; i++)
{
float confidence = detections[i * objectSize + 2];
float x_min = static_cast<int>(detections[i * objectSize + 3] * src.cols);
float y_min = static_cast<int>(detections[i * objectSize + 4] * src.rows);
float x_max = static_cast<int>(detections[i * objectSize + 5] * src.cols);
float y_max = static_cast<int>(detections[i * objectSize + 6] * src.rows);
Rect rect = cv::Rect(cv::Point(x_min, y_min), cv::Point(x_max, y_max));
if (confidence > 0.5)
{
if (rect.width > 150)//车辆
{
char cbuf[255];
sprintf_s(cbuf, "E:/OpenVINO_modelZoo/car_%d.jpg", i_car);
Mat roi = src(rect);
imwrite(cbuf, roi);
cv::rectangle(src, rect, cv::Scalar(255, 255, 255));
i_car++;
}
else//车牌
{
char cbuf[255];
sprintf_s(cbuf, "E:/OpenVINO_modelZoo/plant_%d.jpg", i_plate);
Mat roi = src(rect);
imwrite(cbuf, roi);
cv::rectangle(src, rect, cv::Scalar(0, 0, 255));
i_plate++;
}
}
}这种处理的方法,后面应该还是要用的
二、函数化封装和合并;
以下是原始代码
#include <algorithm>
#include <fstream>
#include <iomanip>
#include <vector>
#include <string>
#include <chrono>
#include <memory>
#include <utility>
#include <format_reader_ptr.h>
#include <inference_engine.hpp>
#include <ext_list.hpp>
#include <samples/slog.hpp>
#include <samples/ocv_common.hpp>
#include "segmentation_demo.h"
using namespace InferenceEngine;
using namespace std;
using namespace cv;
//从图片中获得车和车牌(这里没有输出模型的定位结果,如果需要可以适当修改)
vector< pair<Mat, Mat> > GetCarAndPlate(Mat src)
{
vector<pair<Mat, Mat>> resultVector;
// 模型准备
InferencePlugin plugin(PluginDispatcher().getSuitablePlugin(TargetDevice::eCPU));
plugin.AddExtension(std::make_shared<Extensions::Cpu::CpuExtensions>());//Extension,useful
//读取模型(xml和bin
CNNNetReader networkReader;
networkReader.ReadNetwork("E:/OpenVINO_modelZoo/vehicle-license-plate-detection-barrier-0106.xml");
networkReader.ReadWeights("E:/OpenVINO_modelZoo/vehicle-license-plate-detection-barrier-0106.bin");
CNNNetwork network = networkReader.getNetwork();
network.setBatchSize(1);
// 输入输出准备
InputsDataMap inputInfo(network.getInputsInfo());//获得输入信息
if (inputInfo.size() != 1) throw std::logic_error("错误,该模型应该为单输入");
string inputName = inputInfo.begin()->first;
OutputsDataMap outputInfo(network.getOutputsInfo());//获得输出信息
DataPtr& _output = outputInfo.begin()->second;
const SizeVector outputDims = _output->getTensorDesc().getDims();
string firstOutputName = outputInfo.begin()->first;
int maxProposalCount = outputDims[2];
int objectSize = outputDims[3];
if (objectSize != 7) {
throw std::logic_error("Output should have 7 as a last dimension");
}
if (outputDims.size() != 4) {
throw std::logic_error("Incorrect output dimensions for SSD");
}
_output->setPrecision(Precision::FP32);
_output->setLayout(Layout::NCHW);
// 模型读取和推断
ExecutableNetwork executableNetwork = plugin.LoadNetwork(network, {});
InferRequest infer_request = executableNetwork.CreateInferRequest();
Blob::Ptr lrInputBlob = infer_request.GetBlob(inputName); //data这个名字是我看出来的,实际上这里可以更统一一些
matU8ToBlob<float_t>(src, lrInputBlob, 0);//重要的转换函数,第3个参数是batchSize,应该是自己+1的
infer_request.Infer();
// --------------------------- 8. 处理结果-------------------------------------------------------
const float *detections = infer_request.GetBlob(firstOutputName)->buffer().as<float *>();
int i_car = 0;
int i_plate = 0;
for (int i = 0; i < 200; i++)
{
float confidence = detections[i * objectSize + 2];
float x_min = static_cast<int>(detections[i * objectSize + 3] * src.cols);
float y_min = static_cast<int>(detections[i * objectSize + 4] * src.rows);
float x_max = static_cast<int>(detections[i * objectSize + 5] * src.cols);
float y_max = static_cast<int>(detections[i * objectSize + 6] * src.rows);
Rect rect = cv::Rect(cv::Point(x_min, y_min), cv::Point(x_max, y_max));
if (confidence > 0.5)
{
if (rect.width > 150)//车辆
{
Mat roi = src(rect);
pair<Mat, Mat> aPair;
aPair.first = roi.clone();
resultVector.push_back(aPair);
i_car++;
}
else//车牌
{
Mat roi = src(rect);
resultVector[i_plate].second = roi.clone();
i_plate++;
}
}
}
return resultVector;
}
//从车的图片中识别车型
pair<string,string> GetCarAttributes(Mat src)
{
pair<string, string> resultPair;
// --------------------------- 1.为IE准备插件-------------------------------------
InferencePlugin plugin(PluginDispatcher().getSuitablePlugin(TargetDevice::eCPU));
printPluginVersion(plugin, std::cout);//正确回显表示成功
plugin.AddExtension(std::make_shared<Extensions::Cpu::CpuExtensions>());//Extension,useful
// --------------------------- 2.读取IR模型(xml和bin)---------------------------------
CNNNetReader networkReader;
networkReader.ReadNetwork("E:/OpenVINO_modelZoo/vehicle-attributes-recognition-barrier-0039.xml");
networkReader.ReadWeights("E:/OpenVINO_modelZoo/vehicle-attributes-recognition-barrier-0039.bin");
CNNNetwork network = networkReader.getNetwork();
// --------------------------- 3. 准备输入输出的------------------------------------------
InputsDataMap inputInfo(network.getInputsInfo());//获得输入信息
BlobMap inputBlobs; //保持所有输入的blob数据
if (inputInfo.size() != 1) throw std::logic_error("错误,该模型应该为单输入");
auto lrInputInfoItem = *inputInfo.begin();//开始读入
int w = static_cast<int>(lrInputInfoItem.second->getTensorDesc().getDims()[3]); //这种写法也是可以的,它的first就是data
int h = static_cast<int>(lrInputInfoItem.second->getTensorDesc().getDims()[2]);
network.setBatchSize(1);//只有1副图片,故BatchSize = 1
// --------------------------- 4. 读取模型 ------------------------------------------(后面这些操作应该可以合并了)
ExecutableNetwork executableNetwork = plugin.LoadNetwork(network, {});
// --------------------------- 5. 创建推断 -------------------------------------------------
InferRequest infer_request = executableNetwork.CreateInferRequest();
// --------------------------- 6. 将数据塞入模型 -------------------------------------------------
Blob::Ptr lrInputBlob = infer_request.GetBlob("input"); //data这个名字是我看出来的,实际上这里可以更统一一些
matU8ToBlob<float_t>(src, lrInputBlob, 0);//重要的转换函数,第3个参数是batchSize,应该是自己+1的
// --------------------------- 7. 推断结果 -------------------------------------------------
infer_request.Infer();//多张图片多次推断
// --------------------------- 8. 处理结果-------------------------------------------------------
// 7 possible colors for each vehicle and we should select the one with the maximum probability
auto colorsValues = infer_request.GetBlob("color")->buffer().as<float*>();
// 4 possible types for each vehicle and we should select the one with the maximum probability
auto typesValues = infer_request.GetBlob("type")->buffer().as<float*>();
const auto color_id = std::max_element(colorsValues, colorsValues + 7) - colorsValues;
const auto type_id = std::max_element(typesValues, typesValues + 4) - typesValues;
static const std::string colors[] = {
"white", "gray", "yellow", "red", "green", "blue", "black"
};
static const std::string types[] = {
"car", "bus", "truck", "van"
};
resultPair.first = colors[color_id];
resultPair.second = types[type_id];
return resultPair;
}
//识别车牌
string GetPlateNumber(Mat src)
{
// --------------------------- 1.为IE准备插件-------------------------------------
InferencePlugin plugin(PluginDispatcher().getSuitablePlugin(TargetDevice::eCPU));
plugin.AddExtension(std::make_shared<Extensions::Cpu::CpuExtensions>());//Extension,useful
// --------------------------- 2.读取IR模型(xml和bin)---------------------------------
CNNNetReader networkReader;
networkReader.ReadNetwork("E:/OpenVINO_modelZoo/license-plate-recognition-barrier-0001.xml");
networkReader.ReadWeights("E:/OpenVINO_modelZoo/license-plate-recognition-barrier-0001.bin");
CNNNetwork network = networkReader.getNetwork();
network.setBatchSize(1);//只有1副图片,故BatchSize = 1
// --------------------------- 3. 准备输入输出的------------------------------------------
InputsDataMap inputInfo(network.getInputsInfo());//获得输入信息
BlobMap inputBlobs; //保持所有输入的blob数据
string inputSeqName;
if (inputInfo.size() == 2) {
auto sequenceInput = (++inputInfo.begin());
inputSeqName = sequenceInput->first;
}
else if (inputInfo.size() == 1) {
inputSeqName = "";
}
else {
throw std::logic_error("LPR should have 1 or 2 inputs");
}
InputInfo::Ptr& inputInfoFirst = inputInfo.begin()->second;
inputInfoFirst->setInputPrecision(Precision::U8);
string inputName = inputInfo.begin()->first;
//准备输出数据
OutputsDataMap outputInfo(network.getOutputsInfo());//获得输出信息
if (outputInfo.size() != 1) {
throw std::logic_error("LPR should have 1 output");
}
string firstOutputName = outputInfo.begin()->first;
DataPtr& _output = outputInfo.begin()->second;
const SizeVector outputDims = _output->getTensorDesc().getDims();
// --------------------------- 4. 读取模型 ------------------------------------------(后面这些操作应该可以合并了)
ExecutableNetwork executableNetwork = plugin.LoadNetwork(network, {});
// --------------------------- 5. 创建推断 -------------------------------------------------
InferRequest infer_request = executableNetwork.CreateInferRequest();
// --------------------------- 6. 将数据塞入模型 -------------------------------------------------
Blob::Ptr lrInputBlob = infer_request.GetBlob(inputName); //data这个名字是我看出来的,实际上这里可以更统一一些
matU8ToBlob<uint8_t>(src, lrInputBlob, 0);//重要的转换函数,第3个参数是batchSize,应该是自己+1的
// --------------------------- 7. 推断结果 -------------------------------------------------
infer_request.Infer();//多张图片多次推断
// --------------------------- 8. 处理结果-------------------------------------------------------
static std::vector<std::string> items = {
"0", "1", "2", "3", "4", "5", "6", "7", "8", "9",
"<Anhui>", "<Beijing>", "<Chongqing>", "<Fujian>",
"<Gansu>", "<Guangdong>", "<Guangxi>", "<Guizhou>",
"<Hainan>", "<Hebei>", "<Heilongjiang>", "<Henan>",
"<HongKong>", "<Hubei>", "<Hunan>", "<InnerMongolia>",
"<Jiangsu>", "<Jiangxi>", "<Jilin>", "<Liaoning>",
"<Macau>", "<Ningxia>", "<Qinghai>", "<Shaanxi>",
"<Shandong>", "<Shanghai>", "<Shanxi>", "<Sichuan>",
"<Tianjin>", "<Tibet>", "<Xinjiang>", "<Yunnan>",
"<Zhejiang>", "<police>",
"A", "B", "C", "D", "E", "F", "G", "H", "I", "J",
"K", "L", "M", "N", "O", "P", "Q", "R", "S", "T",
"U", "V", "W", "X", "Y", "Z"
};
const auto data = infer_request.GetBlob(firstOutputName)->buffer().as<float*>();
std::string result;
for (size_t i = 0; i < 88; i++) {
if (data[i] == -1)
break;
result += items[static_cast<size_t>(data[i])];
}
return result;
}
void main()
{
string imageNames = "E:/OpenVINO_modelZoo/沪A51V39.jpg";
Mat src = imread(imageNames);
if (src.empty())
return;
vector<pair<Mat, Mat>> CarAndPlateVector = GetCarAndPlate(src);
for (int i=0;i<CarAndPlateVector.size();i++)
{
pair<Mat, Mat> aPair = CarAndPlateVector[i];
pair<string, string> ColorAndType = GetCarAttributes(aPair.first);
string PlateNumber = GetPlateNumber(aPair.second);
cout << ColorAndType.first <<" "<<ColorAndType.second <<" "<< PlateNumber << endl;
}
cv::waitKey();
}
#include <fstream>
#include <iomanip>
#include <vector>
#include <string>
#include <chrono>
#include <memory>
#include <utility>
#include <format_reader_ptr.h>
#include <inference_engine.hpp>
#include <ext_list.hpp>
#include <samples/slog.hpp>
#include <samples/ocv_common.hpp>
#include "segmentation_demo.h"
using namespace InferenceEngine;
using namespace std;
using namespace cv;
//从图片中获得车和车牌(这里没有输出模型的定位结果,如果需要可以适当修改)
vector< pair<Mat, Mat> > GetCarAndPlate(Mat src)
{
vector<pair<Mat, Mat>> resultVector;
// 模型准备
InferencePlugin plugin(PluginDispatcher().getSuitablePlugin(TargetDevice::eCPU));
plugin.AddExtension(std::make_shared<Extensions::Cpu::CpuExtensions>());//Extension,useful
//读取模型(xml和bin
CNNNetReader networkReader;
networkReader.ReadNetwork("E:/OpenVINO_modelZoo/vehicle-license-plate-detection-barrier-0106.xml");
networkReader.ReadWeights("E:/OpenVINO_modelZoo/vehicle-license-plate-detection-barrier-0106.bin");
CNNNetwork network = networkReader.getNetwork();
network.setBatchSize(1);
// 输入输出准备
InputsDataMap inputInfo(network.getInputsInfo());//获得输入信息
if (inputInfo.size() != 1) throw std::logic_error("错误,该模型应该为单输入");
string inputName = inputInfo.begin()->first;
OutputsDataMap outputInfo(network.getOutputsInfo());//获得输出信息
DataPtr& _output = outputInfo.begin()->second;
const SizeVector outputDims = _output->getTensorDesc().getDims();
string firstOutputName = outputInfo.begin()->first;
int maxProposalCount = outputDims[2];
int objectSize = outputDims[3];
if (objectSize != 7) {
throw std::logic_error("Output should have 7 as a last dimension");
}
if (outputDims.size() != 4) {
throw std::logic_error("Incorrect output dimensions for SSD");
}
_output->setPrecision(Precision::FP32);
_output->setLayout(Layout::NCHW);
// 模型读取和推断
ExecutableNetwork executableNetwork = plugin.LoadNetwork(network, {});
InferRequest infer_request = executableNetwork.CreateInferRequest();
Blob::Ptr lrInputBlob = infer_request.GetBlob(inputName); //data这个名字是我看出来的,实际上这里可以更统一一些
matU8ToBlob<float_t>(src, lrInputBlob, 0);//重要的转换函数,第3个参数是batchSize,应该是自己+1的
infer_request.Infer();
// --------------------------- 8. 处理结果-------------------------------------------------------
const float *detections = infer_request.GetBlob(firstOutputName)->buffer().as<float *>();
int i_car = 0;
int i_plate = 0;
for (int i = 0; i < 200; i++)
{
float confidence = detections[i * objectSize + 2];
float x_min = static_cast<int>(detections[i * objectSize + 3] * src.cols);
float y_min = static_cast<int>(detections[i * objectSize + 4] * src.rows);
float x_max = static_cast<int>(detections[i * objectSize + 5] * src.cols);
float y_max = static_cast<int>(detections[i * objectSize + 6] * src.rows);
Rect rect = cv::Rect(cv::Point(x_min, y_min), cv::Point(x_max, y_max));
if (confidence > 0.5)
{
if (rect.width > 150)//车辆
{
Mat roi = src(rect);
pair<Mat, Mat> aPair;
aPair.first = roi.clone();
resultVector.push_back(aPair);
i_car++;
}
else//车牌
{
Mat roi = src(rect);
resultVector[i_plate].second = roi.clone();
i_plate++;
}
}
}
return resultVector;
}
//从车的图片中识别车型
pair<string,string> GetCarAttributes(Mat src)
{
pair<string, string> resultPair;
// --------------------------- 1.为IE准备插件-------------------------------------
InferencePlugin plugin(PluginDispatcher().getSuitablePlugin(TargetDevice::eCPU));
printPluginVersion(plugin, std::cout);//正确回显表示成功
plugin.AddExtension(std::make_shared<Extensions::Cpu::CpuExtensions>());//Extension,useful
// --------------------------- 2.读取IR模型(xml和bin)---------------------------------
CNNNetReader networkReader;
networkReader.ReadNetwork("E:/OpenVINO_modelZoo/vehicle-attributes-recognition-barrier-0039.xml");
networkReader.ReadWeights("E:/OpenVINO_modelZoo/vehicle-attributes-recognition-barrier-0039.bin");
CNNNetwork network = networkReader.getNetwork();
// --------------------------- 3. 准备输入输出的------------------------------------------
InputsDataMap inputInfo(network.getInputsInfo());//获得输入信息
BlobMap inputBlobs; //保持所有输入的blob数据
if (inputInfo.size() != 1) throw std::logic_error("错误,该模型应该为单输入");
auto lrInputInfoItem = *inputInfo.begin();//开始读入
int w = static_cast<int>(lrInputInfoItem.second->getTensorDesc().getDims()[3]); //这种写法也是可以的,它的first就是data
int h = static_cast<int>(lrInputInfoItem.second->getTensorDesc().getDims()[2]);
network.setBatchSize(1);//只有1副图片,故BatchSize = 1
// --------------------------- 4. 读取模型 ------------------------------------------(后面这些操作应该可以合并了)
ExecutableNetwork executableNetwork = plugin.LoadNetwork(network, {});
// --------------------------- 5. 创建推断 -------------------------------------------------
InferRequest infer_request = executableNetwork.CreateInferRequest();
// --------------------------- 6. 将数据塞入模型 -------------------------------------------------
Blob::Ptr lrInputBlob = infer_request.GetBlob("input"); //data这个名字是我看出来的,实际上这里可以更统一一些
matU8ToBlob<float_t>(src, lrInputBlob, 0);//重要的转换函数,第3个参数是batchSize,应该是自己+1的
// --------------------------- 7. 推断结果 -------------------------------------------------
infer_request.Infer();//多张图片多次推断
// --------------------------- 8. 处理结果-------------------------------------------------------
// 7 possible colors for each vehicle and we should select the one with the maximum probability
auto colorsValues = infer_request.GetBlob("color")->buffer().as<float*>();
// 4 possible types for each vehicle and we should select the one with the maximum probability
auto typesValues = infer_request.GetBlob("type")->buffer().as<float*>();
const auto color_id = std::max_element(colorsValues, colorsValues + 7) - colorsValues;
const auto type_id = std::max_element(typesValues, typesValues + 4) - typesValues;
static const std::string colors[] = {
"white", "gray", "yellow", "red", "green", "blue", "black"
};
static const std::string types[] = {
"car", "bus", "truck", "van"
};
resultPair.first = colors[color_id];
resultPair.second = types[type_id];
return resultPair;
}
//识别车牌
string GetPlateNumber(Mat src)
{
// --------------------------- 1.为IE准备插件-------------------------------------
InferencePlugin plugin(PluginDispatcher().getSuitablePlugin(TargetDevice::eCPU));
plugin.AddExtension(std::make_shared<Extensions::Cpu::CpuExtensions>());//Extension,useful
// --------------------------- 2.读取IR模型(xml和bin)---------------------------------
CNNNetReader networkReader;
networkReader.ReadNetwork("E:/OpenVINO_modelZoo/license-plate-recognition-barrier-0001.xml");
networkReader.ReadWeights("E:/OpenVINO_modelZoo/license-plate-recognition-barrier-0001.bin");
CNNNetwork network = networkReader.getNetwork();
network.setBatchSize(1);//只有1副图片,故BatchSize = 1
// --------------------------- 3. 准备输入输出的------------------------------------------
InputsDataMap inputInfo(network.getInputsInfo());//获得输入信息
BlobMap inputBlobs; //保持所有输入的blob数据
string inputSeqName;
if (inputInfo.size() == 2) {
auto sequenceInput = (++inputInfo.begin());
inputSeqName = sequenceInput->first;
}
else if (inputInfo.size() == 1) {
inputSeqName = "";
}
else {
throw std::logic_error("LPR should have 1 or 2 inputs");
}
InputInfo::Ptr& inputInfoFirst = inputInfo.begin()->second;
inputInfoFirst->setInputPrecision(Precision::U8);
string inputName = inputInfo.begin()->first;
//准备输出数据
OutputsDataMap outputInfo(network.getOutputsInfo());//获得输出信息
if (outputInfo.size() != 1) {
throw std::logic_error("LPR should have 1 output");
}
string firstOutputName = outputInfo.begin()->first;
DataPtr& _output = outputInfo.begin()->second;
const SizeVector outputDims = _output->getTensorDesc().getDims();
// --------------------------- 4. 读取模型 ------------------------------------------(后面这些操作应该可以合并了)
ExecutableNetwork executableNetwork = plugin.LoadNetwork(network, {});
// --------------------------- 5. 创建推断 -------------------------------------------------
InferRequest infer_request = executableNetwork.CreateInferRequest();
// --------------------------- 6. 将数据塞入模型 -------------------------------------------------
Blob::Ptr lrInputBlob = infer_request.GetBlob(inputName); //data这个名字是我看出来的,实际上这里可以更统一一些
matU8ToBlob<uint8_t>(src, lrInputBlob, 0);//重要的转换函数,第3个参数是batchSize,应该是自己+1的
// --------------------------- 7. 推断结果 -------------------------------------------------
infer_request.Infer();//多张图片多次推断
// --------------------------- 8. 处理结果-------------------------------------------------------
static std::vector<std::string> items = {
"0", "1", "2", "3", "4", "5", "6", "7", "8", "9",
"<Anhui>", "<Beijing>", "<Chongqing>", "<Fujian>",
"<Gansu>", "<Guangdong>", "<Guangxi>", "<Guizhou>",
"<Hainan>", "<Hebei>", "<Heilongjiang>", "<Henan>",
"<HongKong>", "<Hubei>", "<Hunan>", "<InnerMongolia>",
"<Jiangsu>", "<Jiangxi>", "<Jilin>", "<Liaoning>",
"<Macau>", "<Ningxia>", "<Qinghai>", "<Shaanxi>",
"<Shandong>", "<Shanghai>", "<Shanxi>", "<Sichuan>",
"<Tianjin>", "<Tibet>", "<Xinjiang>", "<Yunnan>",
"<Zhejiang>", "<police>",
"A", "B", "C", "D", "E", "F", "G", "H", "I", "J",
"K", "L", "M", "N", "O", "P", "Q", "R", "S", "T",
"U", "V", "W", "X", "Y", "Z"
};
const auto data = infer_request.GetBlob(firstOutputName)->buffer().as<float*>();
std::string result;
for (size_t i = 0; i < 88; i++) {
if (data[i] == -1)
break;
result += items[static_cast<size_t>(data[i])];
}
return result;
}
void main()
{
string imageNames = "E:/OpenVINO_modelZoo/沪A51V39.jpg";
Mat src = imread(imageNames);
if (src.empty())
return;
vector<pair<Mat, Mat>> CarAndPlateVector = GetCarAndPlate(src);
for (int i=0;i<CarAndPlateVector.size();i++)
{
pair<Mat, Mat> aPair = CarAndPlateVector[i];
pair<string, string> ColorAndType = GetCarAttributes(aPair.first);
string PlateNumber = GetPlateNumber(aPair.second);
cout << ColorAndType.first <<" "<<ColorAndType.second <<" "<< PlateNumber << endl;
}
cv::waitKey();
}
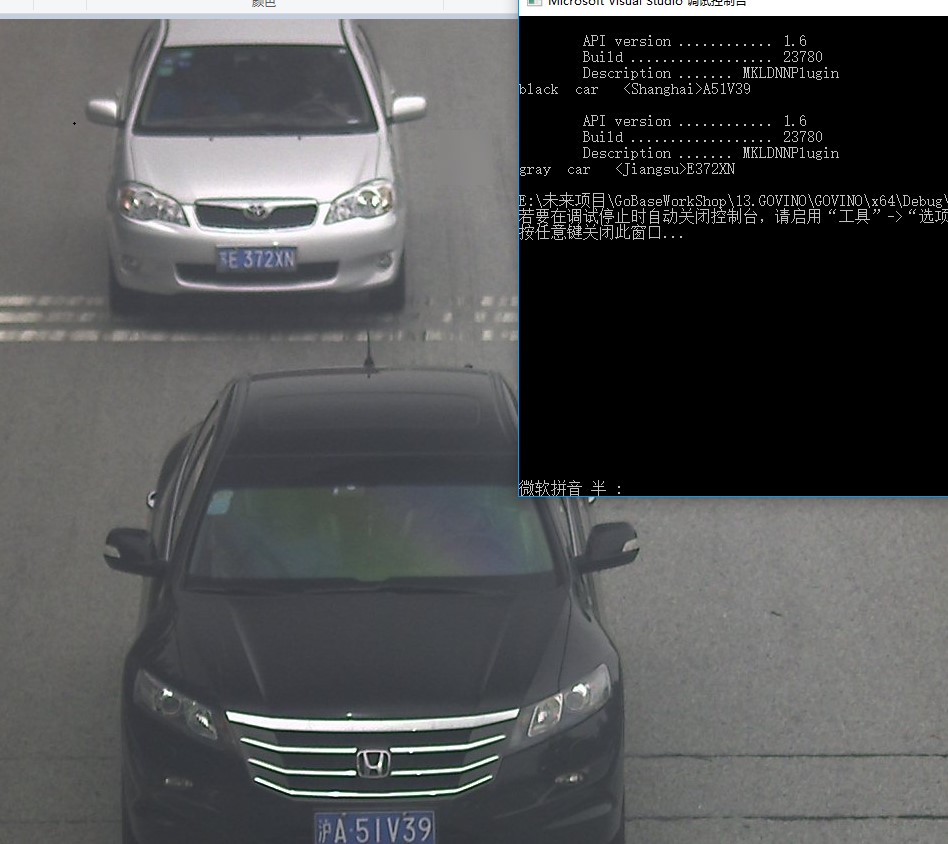
能够合并到这种程度是很有价值的,下一步可以思考找到更多的数据集进行训练,并且将这个结果进行转换。
从结果上来看,已经实现了级联问题,从这个层面是没有问题的;只有在具体的需求面前才可能看出问题。
我需要拓展一下车牌识别的真实需求,也许这个会成为我真正DL4CV的开始。
三、异步机制探索;
一直以来,我都为视频处理的速度问题所困扰,在“视频流”的处理过程中,必须首先获得一帧的数据,然后才能够处理这一帧的数据,并且得到增强的结果——那么最后处理的速度必然同时受到视频采集和图像处理的限制。
这可能类似于CPU中流的处理,而且据我所知,这方面的研究不仅开始很久,而且效果显著,但是苦于一直没有一个可以参考的实现。现在OpenVINO中对于视频的处理,应该说是解决燃眉之急。
OpenVINO中相关函数
使用StartAsync和Wait来实现异步操作
(Do inference by calling the InferenceEngine::InferRequest::StartAsync and InferenceEngine::InferRequest::Wait methods for asynchronous request):
infer_request->StartAsync();
infer_request.Wait(IInferRequest::WaitMode::RESULT_READY);
infer_request.Wait(IInferRequest::WaitMode::RESULT_READY);
或者采用Infer 来实现同步操作(or by calling the InferenceEngine::InferRequest::Infer method for synchronous request):
sync_infer_request->Infer();
在同步模式下推断函数Infer会一直阻塞,直到执行结束;在异步模式下推断调用函数StartAsync会立即返回,通过检查。
对于视频分析、视频中对象检测,OpenVINO官方建议通过异步方式可以实现更快的帧率处理.
异步虽然好,但是如果仅是这样改写

则价值不大,和同步没有区分。必须建立相应的机制:
在例子中,是这样建立的:
首先是创建:
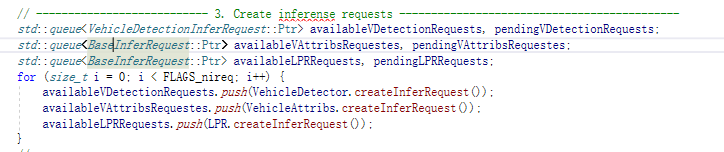
每一个检测,都包含available和pending两个部分,在创建的初期,根据FLAGS_nireq这个参数,来设定开几个available(可以理解开几个线程)
在每一个推断的开始,首先判断available是否还有,如果所有的available都已经被使用,那么就必须要开始运算
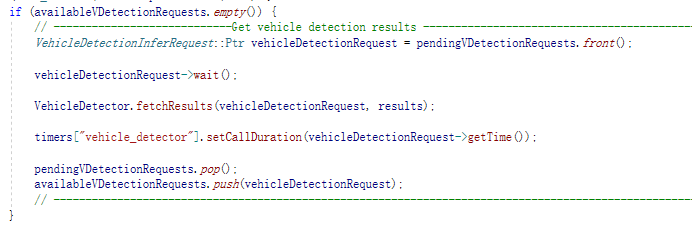
其中的

肯定就是在这个地方等待结果的;
如果avaiable还有,直接将推断送到下一个avaiable中去:
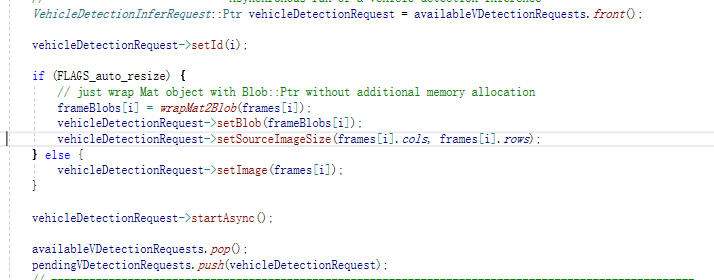
并且立刻开始推断。这样的话,就可以实现多个pending都在推断的状况。
在FLAGS_nireq被设置为1的时候
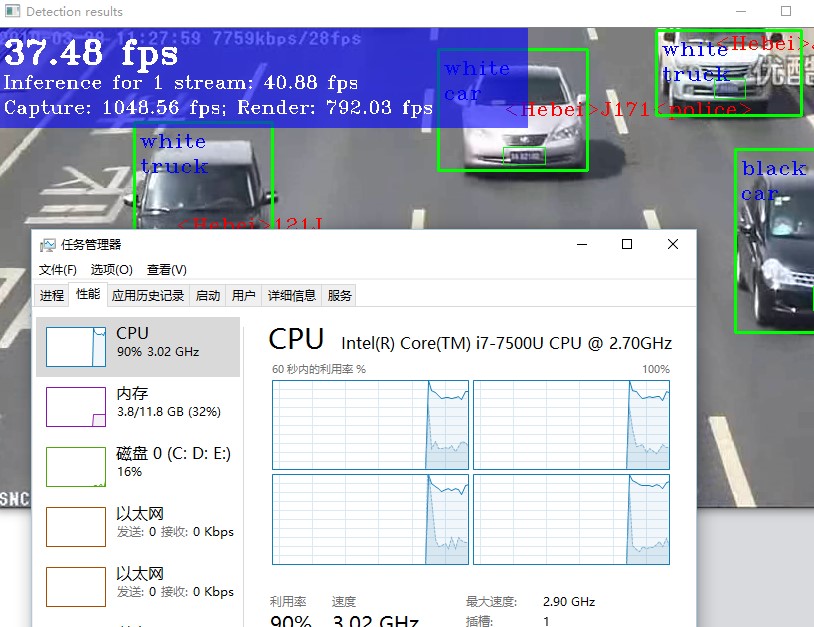
设置为3的时候
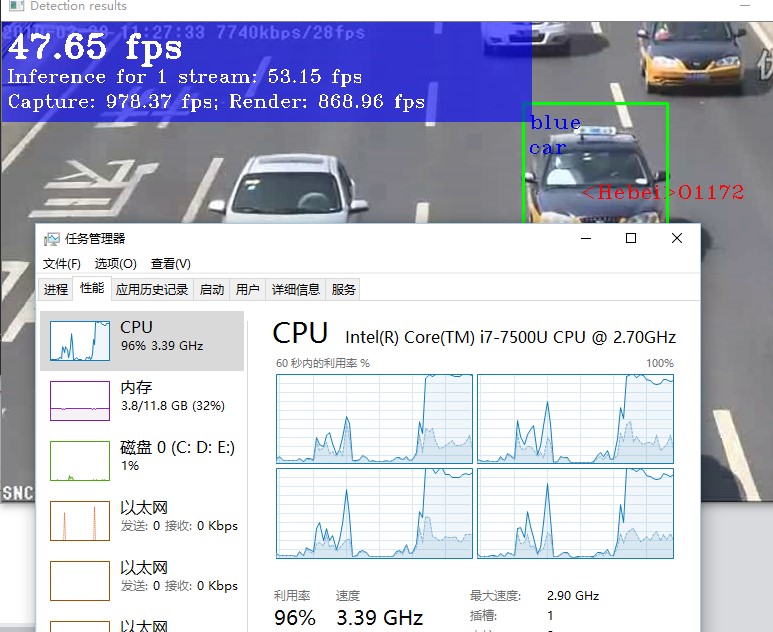
有所提高,但是不明显。
做一个4宫格
-nireq 3 -i E:/未来项目/炼数成金/(录制中)端到端/L9/道路监控数据集/1.avi E:/未来项目/炼数成金/(录制中)端到端/L9/道路监控数据集/2.avi E:/未来项目/炼数成金/(录制中)端到端/L9/道路监控数据集/1.avi E:/未来项目/炼数成金/(录制中)端到端/L9/道路监控数据集/2.avi -m E:/OpenVINO_modelZoo/vehicle-license-plate-detection-barrier-0106.xml -m_va E:/OpenVINO_modelZoo/vehicle-attributes-recognition-barrier-0039.xml -m_lpr E:/OpenVINO_modelZoo/license-plate-recognition-barrier-0001.xml
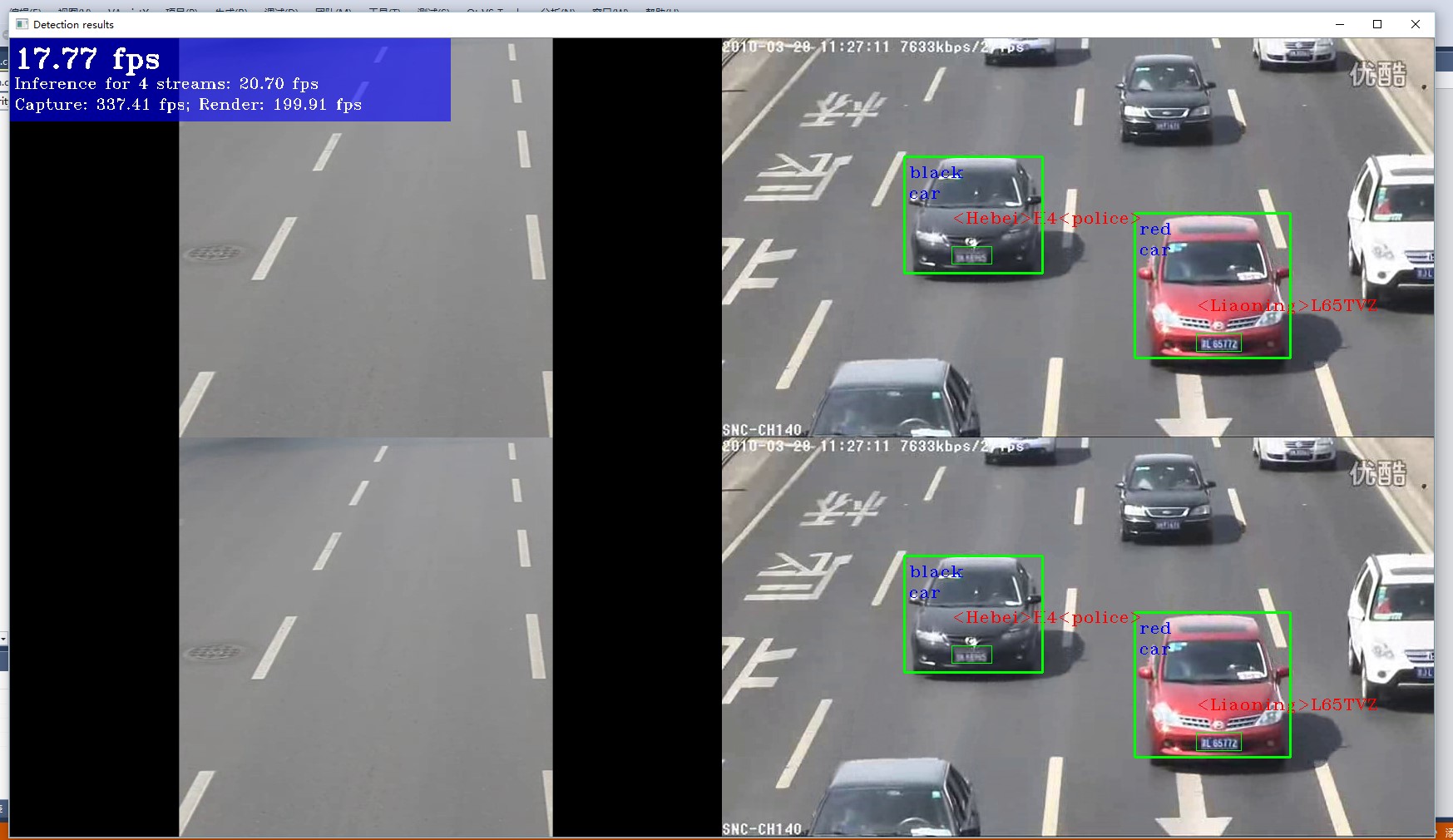
但是为了进一步研究问题,需要具体做例子来实验。
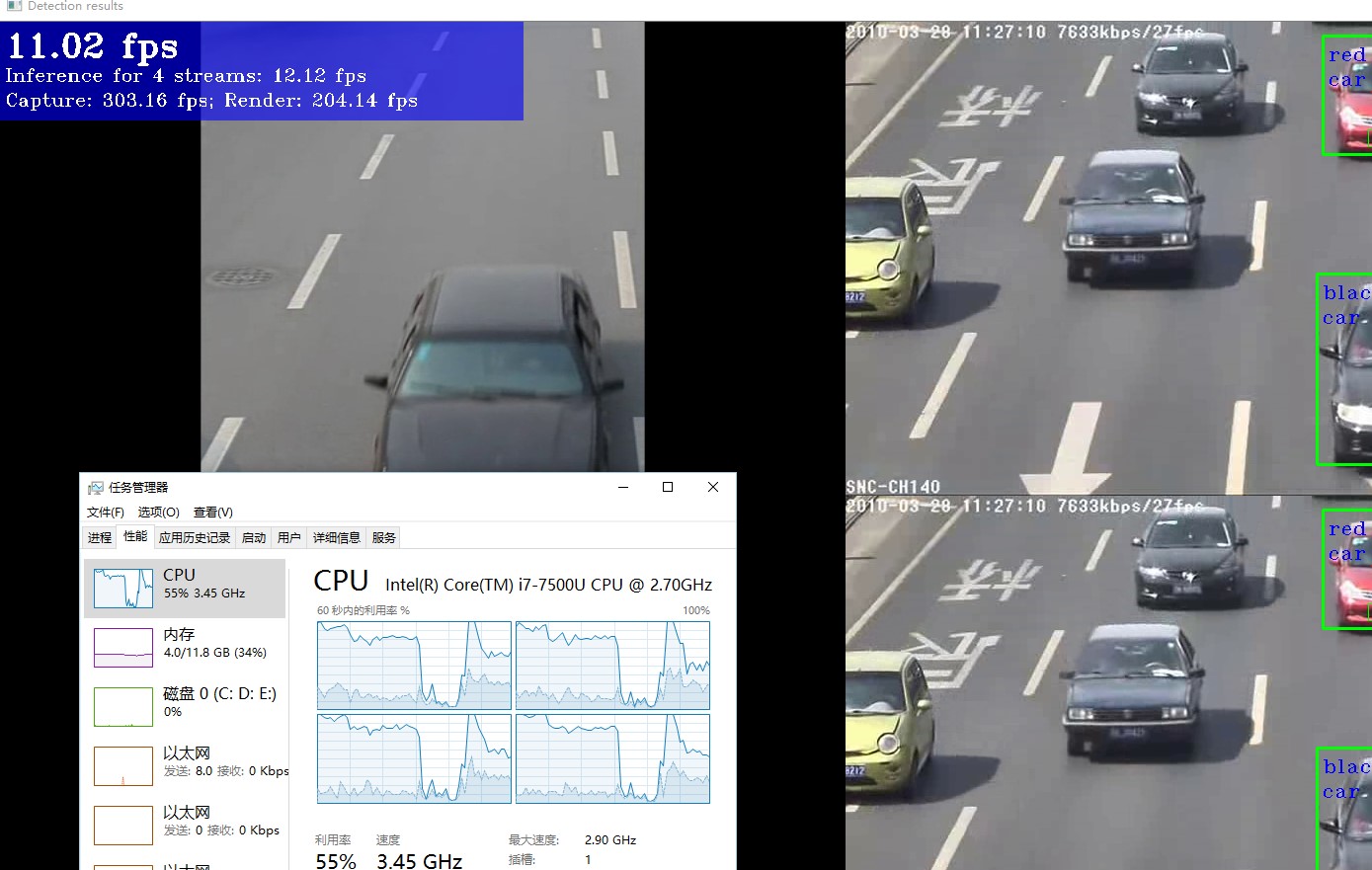
好吧,还是有所差异的。
四、类的封装其价值
最终该机制的速度不会快于单个推断速度,我们至少可以将整个操作分为”数据准备“”数据推断“和”数据显示“3个部分。
我们需要的是打开VideoCapture的相关代码
VideoCapture capture("E:/未来项目/炼数成金/(录制中)端到端/L9/道路监控数据集/2.avi");
Mat src;
while (true)
{
if (!capture.read(src))
break;
……
Mat src;
while (true)
{
if (!capture.read(src))
break;
……
然后代码必须经过函数化(过程化无法被集成)和结构化(初始化的东西必须被独立出来,甚至可能会导致错误),参考现有例子是最方便的方式。然后对于生成的结果,我们需要做较为精确的测量。
其中有一个非常重要的“保护机制”,一定要注意对这个“机制”的理解,否则很容易出现下图问题
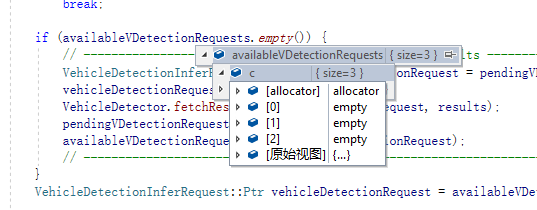
相比较之下,正确调用产生的结果
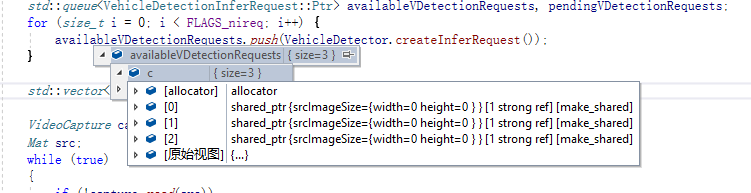
其来源
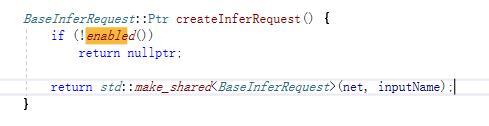
也就是在我们调用createInferRequest的时候,会首先判断当前detection的enabled,如果这个enabled为false,则直接退出为空。
而这个定义是被写死的
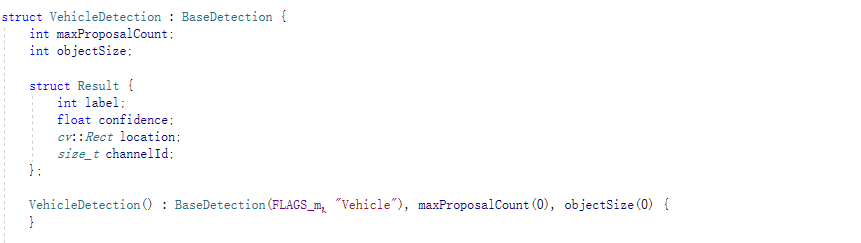
它在当前Detection(比如VehicleDetection)被创建的时候产生。由于原代码中FLAGS_m是作为参数输入的,则会定义;但是我们将代码独立出来,则这个地方是没有定义的,那么久比需将其规避掉。包括,将这个FLAGS_m直接写入

和将模型调用的参数直接写入
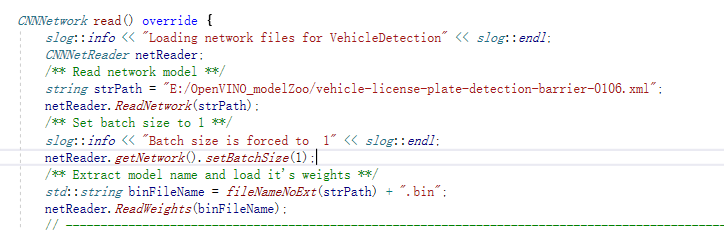
现在回顾这里的异步机制,它之所以能够提高速度,本质上还是较好的架构,我们通过画图来说明。
我们将整个处理的时间分为3个部分


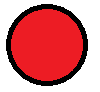
其中红、黄、蓝分别代表第1、2、3帧
原机制为
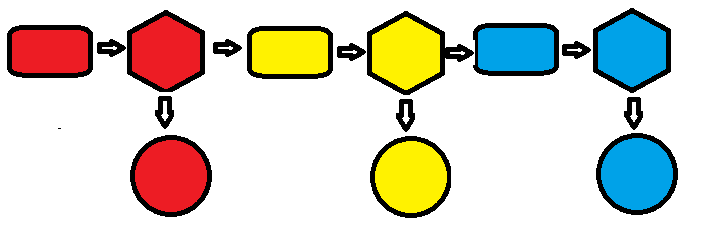
时间为C1+P1+C2+P2+C3+P3
使用机制进行了乱序
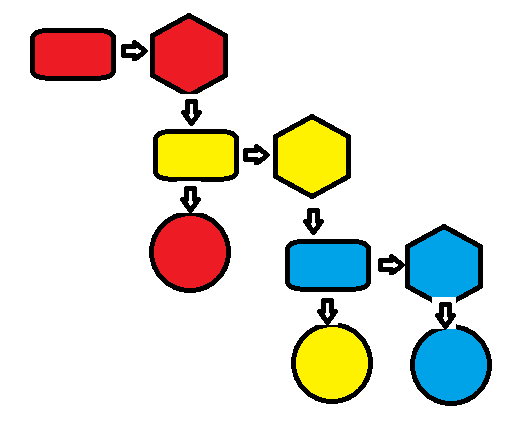
消耗时间不会大于(一般认为P>>C),C1+P1+P2+P3。能够将部分时间进行重叠,从而达到提高速度目的。
小结:
1、OpenVINO的推断操作比较快(最终该机制的速度不会快于单个推断速度,它只是将数据准备和数据显示进行重叠);
2、它的原子操作提供了这种“线程独立安全”的运算;
3、只有在满足"原子操作线程独立“的基础上,才能够去做这样的操作。这种方法,将来要积极运用。