- 介绍了Spark的特点,历史
- 介绍了Spark的安装
- 介绍了Spark的局限性
- 介绍了Spark的基本工作原理
- 演示了一个Hello World例子
- 演示了一个Initiation例子
Source
Spark是大数据学习的一个常用框架,很重要。下面就对以前曾经上过的课程做一个总结回顾。
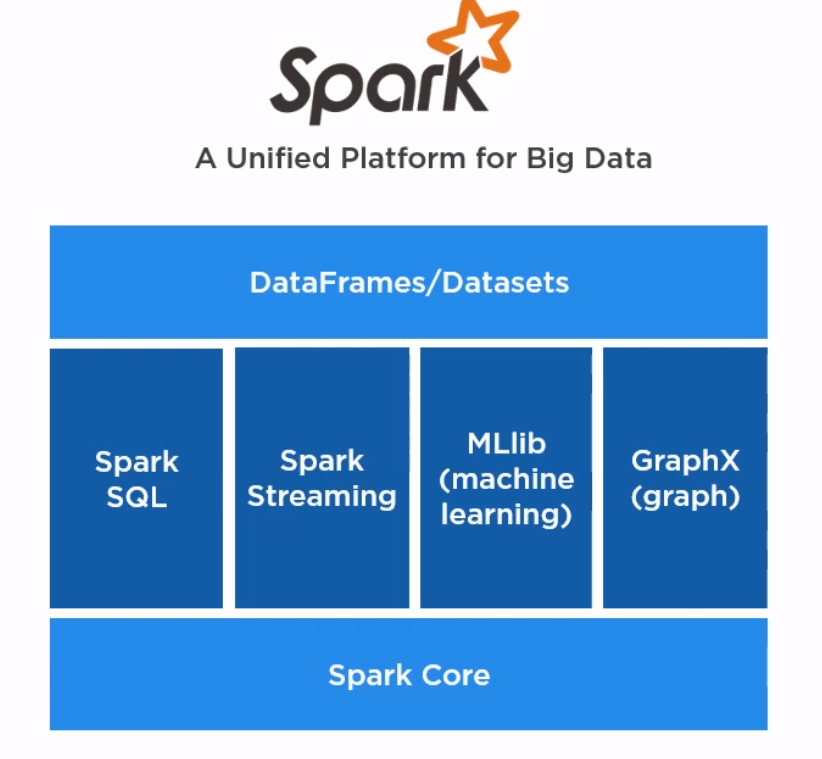
(注:本文源自Pluralsight上的课程Apache Spark Fundamentals,By Justin Pihony。)
Why Spark?
Features
- Fast
- Easy to code
Hadoop v.s. Spark
- Hadoop usually works with other framework together like Stream, Hive, HBase, etc to perform complex tasks.
- Spark includes everything in one bucket, is a unified platform for Big Data.
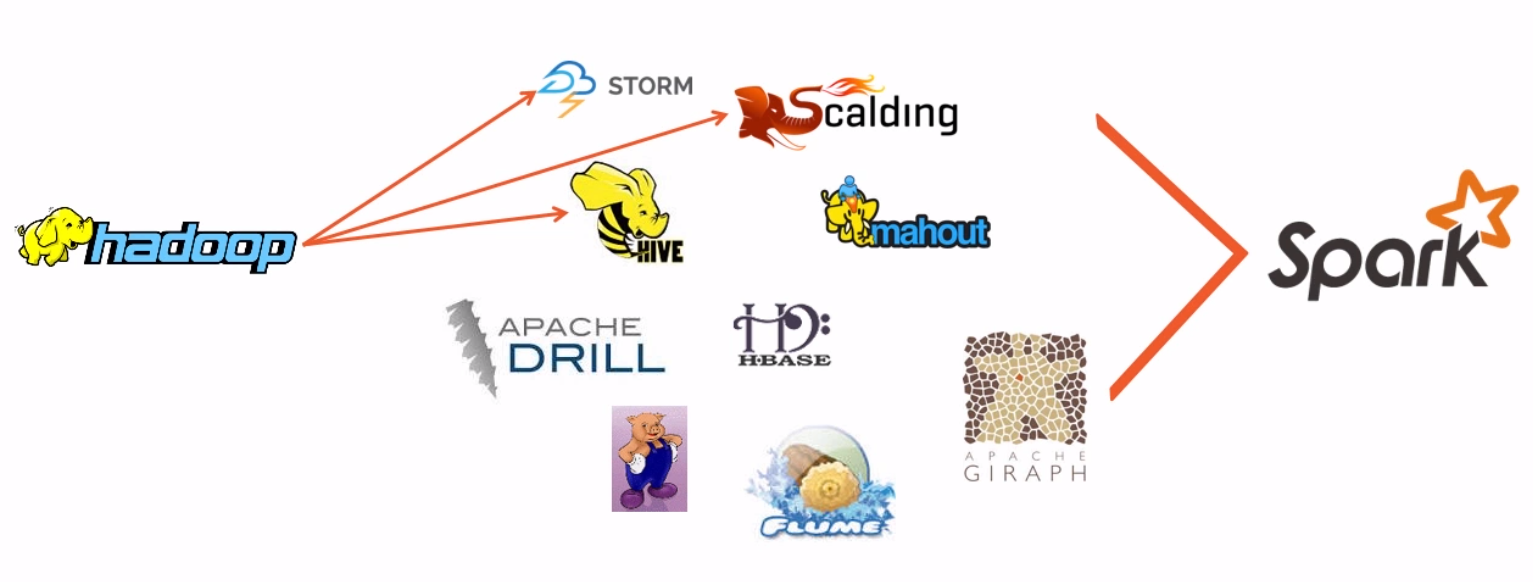
History of Spark
- 2004 -> MapReduce
- 2006 -> Hadoop
- 2009 -> Spark
- 2016 -> Spark 2.x
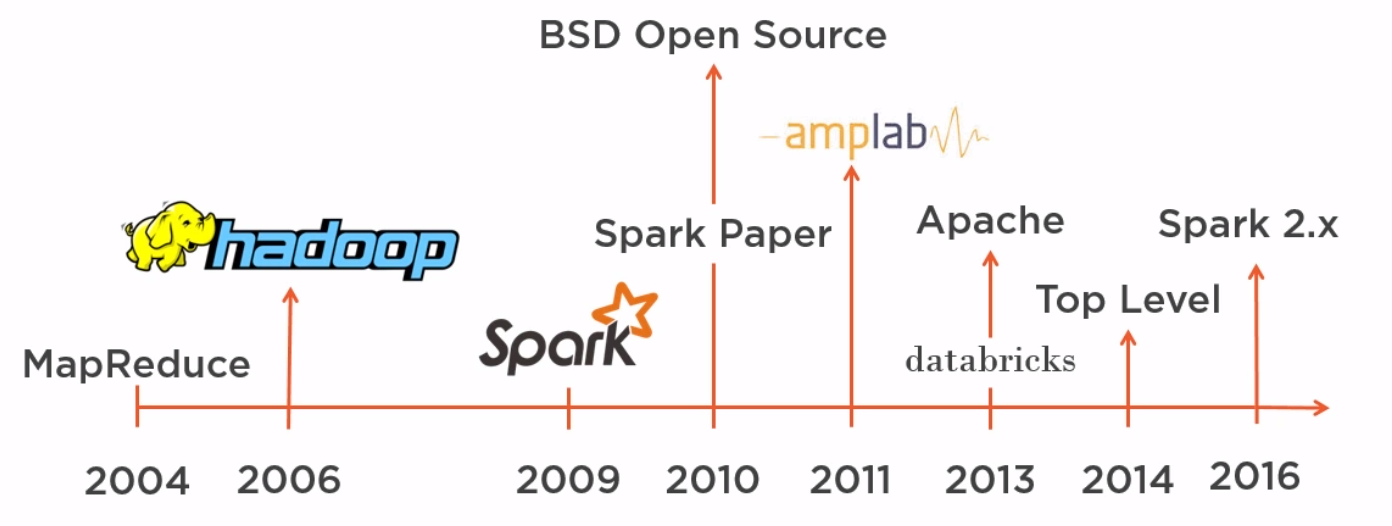
总结:技术/软件的发展是螺旋上升式的。04年MapReduce概念被提出,06年出现Hadoop,之后又出了各种支持性的package,这时候可以说是大数据的野蛮生长时期。
之后Spark出来,把常用的功能整合一下集成在自家平台,就像当年Spring干的事一样。因为好用,性能也不错,于是大家都喜欢用。
Why not Spark?
大数据是现在新兴的一个热点,但是实际上并不是所有场景都适用大数据的解决方案(Hadoop,Spark)。在使用之前,我们需要好好想一想:Do We Really Need Big Data Solution?
下面介绍三篇文章,列举了Spark/Big Data的局限性。
For Big Data, Moore’s Law Means Better Decisions这篇文章说,数据量的增长已经超过了摩尔定律,光光是使用big data processing工具并不行,我们还得更加聪明得对于有效数据进行选择,使用抽样等方式缩小需要处理得数据量。
Don't use Hadoop - your data isn't that big这篇文章说,Hadoop虽然很强大,但是局限性也很多,用起来没有SQL或者Python Script顺手,如果数据量在5TB一下,没有必要使用Hadoop。
Command-line Tools can be 235x Faster than your Hadoop Cluster这篇文章从实战的角度出发,用cmd line tool和hadoop进行对比,分析一个3G左右的文件,最终的结果是cmd完胜。
Spark Language
Spark supports a variety of programming languages.
- Scala
- Java
- Python
- R
Get Started - Install
总的来说,还是蛮简单的,下载,解压,然后配置一些路径。
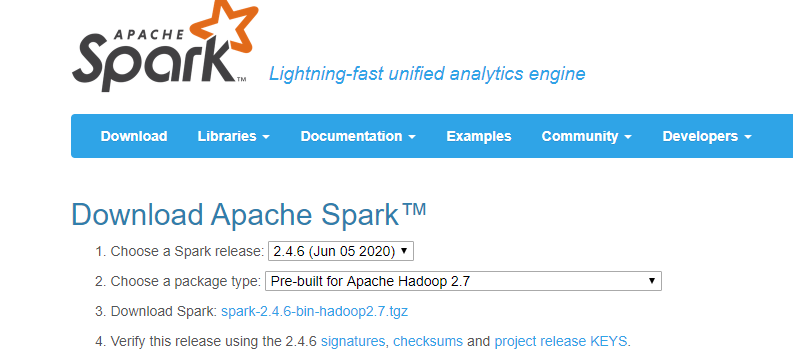
Windows Steps
- unzip
- set up
- set up Spark bin to PATH
- set up SPARK_HOME
- set up log level (optional)
- fix a bug of SPARK-2356
- download winutils.exe and put under bin folder
- set up HADOOP_HOME
- open
spark-shell
in cmd
Spark Mechanics
Spark也是主从模式,一个Driver,多个Worker
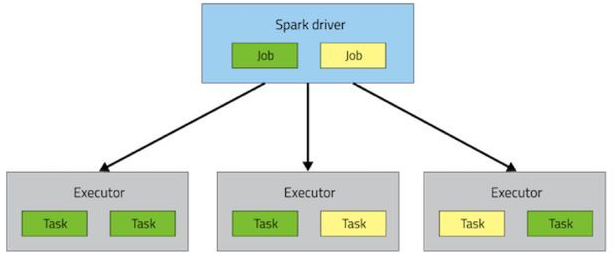
Spark Context在Driver中扮演了非常重要的角色,它负责创建并分发task,当然,也负责load balance的工作。
- Task creator
- Scheduler
- Data locality
- Fault tolerance
Get Started - Hello World Example
Read a text file and output the first line
val textFile = sc.textFile("file:///spark-3.0.0-bin-hadoop2.7/README.md")
textFile.first
->
Count the word appearance in the file
val tokenizedFileData = textFile.flatMap(line=>line.split(" "))
val countPrep = tokenizedFileData.map(word=>(word, 1))
val counts = countPrep.reduceByKey((accumValue, newValue)=>accumValue + newValue)
val sortedCounts = counts.sortBy(kvPair=>kvPair._2, false)
sortedCounts.saveAsTextFile("file:///ReadMeWordCount")
->
Or directly call the API
tokenizedFileData.countByValue
->
Get Started - Initiation Example
在上述Hello World例子中,第一步是sc.textFile
,这个sc
是什么,从哪里来的呢?
其实,sc
代表了SparkContext
。(和Spring的Application Context类似)在Spark Shell中,系统自动帮我们创建了一个SparkContext
,所以我们不需要重复创建。如果是在console之外自己写一个app,那么第一件事就是创建SparkContext
,如下:
import org.apache.spark.SparkContext
import org.apache.spark.SparkConf
val conf = new SparkConf().setAppName(appName).setMaster(master)
new SparkContext(conf)