一、代码
import numpy as np
import matplotlib as mpl
import matplotlib.pyplot as plt
import pandas as pd
from sklearn.model_selection import train_test_split
from sklearn.linear_model import Lasso, Ridge
from sklearn.model_selection import GridSearchCV
if __name__ == "__main__":
# pandas读入
data = pd.read_csv('D:/data_set/Advertising.csv') # TV、Radio、Newspaper、Sales
# print(type(data)) # <class 'pandas.core.frame.DataFrame'>
print('data=
',data)
x = data[['TV', 'Radio', 'Newspaper']]
# x = data[['TV', 'Radio']]
y = data['Sales']
print('X=
',x)
print('Y=
',y)
x_train, x_test, y_train, y_test = train_test_split(x, y, random_state=1, train_size=0.8)
# model = Lasso()
model = Ridge()
alpha_can = np.logspace(-3, 2, 10)
print(alpha_can)
np.set_printoptions(suppress=True) # 科学计数法(默认)
print('alpha_can =
', alpha_can)
lasso_model = GridSearchCV(model, param_grid={'alpha': alpha_can}, cv=5) # 五折交叉验证
lasso_model.fit(x_train, y_train)
print('超参数:
', lasso_model.best_params_)
order = y_test.argsort(axis=0)
print('order:',order)
y_test = y_test.values[order]
print('y_test_now =
',y_test)
x_test = x_test.values[order, :]
print('x_test_now =
',x_test)
y_hat = lasso_model.predict(x_test) # y_hat是预测值
print('lasso_model.score(x_test,y_test):',lasso_model.score(x_test, y_test))
mse = np.average((y_hat - np.array(y_test)) ** 2) # Mean Squared Error
rmse = np.sqrt(mse) # Root Mean Squared Err or
print('mse = ',mse,'rmse = ',rmse)
t = np.arange(len(x_test))
mpl.rcParams['font.sans-serif'] = ['simHei']
mpl.rcParams['axes.unicode_minus'] = False
plt.figure(facecolor='w')
plt.plot(t, y_test, 'r-', linewidth=2, label='真实数据')
plt.plot(t, y_hat, 'g-', linewidth=2, label='预测数据')
plt.title('线性回归预测销量', fontsize=18)
plt.legend(loc='upper left')
plt.grid(b=True, ls=':')
plt.show()
二、运行结果
data=
Unnamed: 0 TV Radio Newspaper Sales
0 1 230.1 37.8 69.2 22.1
1 2 44.5 39.3 45.1 10.4
2 3 17.2 45.9 69.3 9.3
3 4 151.5 41.3 58.5 18.5
4 5 180.8 10.8 58.4 12.9
5 6 8.7 48.9 75.0 7.2
6 7 57.5 32.8 23.5 11.8
7 8 120.2 19.6 11.6 13.2
8 9 8.6 2.1 1.0 4.8
9 10 199.8 2.6 21.2 10.6
10 11 66.1 5.8 24.2 8.6
11 12 214.7 24.0 4.0 17.4
12 13 23.8 35.1 65.9 9.2
13 14 97.5 7.6 7.2 9.7
14 15 204.1 32.9 46.0 19.0
15 16 195.4 47.7 52.9 22.4
16 17 67.8 36.6 114.0 12.5
17 18 281.4 39.6 55.8 24.4
18 19 69.2 20.5 18.3 11.3
19 20 147.3 23.9 19.1 14.6
20 21 218.4 27.7 53.4 18.0
21 22 237.4 5.1 23.5 12.5
22 23 13.2 15.9 49.6 5.6
23 24 228.3 16.9 26.2 15.5
24 25 62.3 12.6 18.3 9.7
25 26 262.9 3.5 19.5 12.0
26 27 142.9 29.3 12.6 15.0
27 28 240.1 16.7 22.9 15.9
28 29 248.8 27.1 22.9 18.9
29 30 70.6 16.0 40.8 10.5
.. ... ... ... ... ...
170 171 50.0 11.6 18.4 8.4
171 172 164.5 20.9 47.4 14.5
172 173 19.6 20.1 17.0 7.6
173 174 168.4 7.1 12.8 11.7
174 175 222.4 3.4 13.1 11.5
175 176 276.9 48.9 41.8 27.0
176 177 248.4 30.2 20.3 20.2
177 178 170.2 7.8 35.2 11.7
178 179 276.7 2.3 23.7 11.8
179 180 165.6 10.0 17.6 12.6
180 181 156.6 2.6 8.3 10.5
181 182 218.5 5.4 27.4 12.2
182 183 56.2 5.7 29.7 8.7
183 184 287.6 43.0 71.8 26.2
184 185 253.8 21.3 30.0 17.6
185 186 205.0 45.1 19.6 22.6
186 187 139.5 2.1 26.6 10.3
187 188 191.1 28.7 18.2 17.3
188 189 286.0 13.9 3.7 15.9
189 190 18.7 12.1 23.4 6.7
190 191 39.5 41.1 5.8 10.8
191 192 75.5 10.8 6.0 9.9
192 193 17.2 4.1 31.6 5.9
193 194 166.8 42.0 3.6 19.6
194 195 149.7 35.6 6.0 17.3
195 196 38.2 3.7 13.8 7.6
196 197 94.2 4.9 8.1 9.7
197 198 177.0 9.3 6.4 12.8
198 199 283.6 42.0 66.2 25.5
199 200 232.1 8.6 8.7 13.4
[200 rows x 5 columns]
X=
TV Radio Newspaper
0 230.1 37.8 69.2
1 44.5 39.3 45.1
2 17.2 45.9 69.3
3 151.5 41.3 58.5
4 180.8 10.8 58.4
5 8.7 48.9 75.0
6 57.5 32.8 23.5
7 120.2 19.6 11.6
8 8.6 2.1 1.0
9 199.8 2.6 21.2
10 66.1 5.8 24.2
11 214.7 24.0 4.0
12 23.8 35.1 65.9
13 97.5 7.6 7.2
14 204.1 32.9 46.0
15 195.4 47.7 52.9
16 67.8 36.6 114.0
17 281.4 39.6 55.8
18 69.2 20.5 18.3
19 147.3 23.9 19.1
20 218.4 27.7 53.4
21 237.4 5.1 23.5
22 13.2 15.9 49.6
23 228.3 16.9 26.2
24 62.3 12.6 18.3
25 262.9 3.5 19.5
26 142.9 29.3 12.6
27 240.1 16.7 22.9
28 248.8 27.1 22.9
29 70.6 16.0 40.8
.. ... ... ...
170 50.0 11.6 18.4
171 164.5 20.9 47.4
172 19.6 20.1 17.0
173 168.4 7.1 12.8
174 222.4 3.4 13.1
175 276.9 48.9 41.8
176 248.4 30.2 20.3
177 170.2 7.8 35.2
178 276.7 2.3 23.7
179 165.6 10.0 17.6
180 156.6 2.6 8.3
181 218.5 5.4 27.4
182 56.2 5.7 29.7
183 287.6 43.0 71.8
184 253.8 21.3 30.0
185 205.0 45.1 19.6
186 139.5 2.1 26.6
187 191.1 28.7 18.2
188 286.0 13.9 3.7
189 18.7 12.1 23.4
190 39.5 41.1 5.8
191 75.5 10.8 6.0
192 17.2 4.1 31.6
193 166.8 42.0 3.6
194 149.7 35.6 6.0
195 38.2 3.7 13.8
196 94.2 4.9 8.1
197 177.0 9.3 6.4
198 283.6 42.0 66.2
199 232.1 8.6 8.7
[200 rows x 3 columns]
Y=
0 22.1
1 10.4
2 9.3
3 18.5
4 12.9
5 7.2
6 11.8
7 13.2
8 4.8
9 10.6
10 8.6
11 17.4
12 9.2
13 9.7
14 19.0
15 22.4
16 12.5
17 24.4
18 11.3
19 14.6
20 18.0
21 12.5
22 5.6
23 15.5
24 9.7
25 12.0
26 15.0
27 15.9
28 18.9
29 10.5
...
170 8.4
171 14.5
172 7.6
173 11.7
174 11.5
175 27.0
176 20.2
177 11.7
178 11.8
179 12.6
180 10.5
181 12.2
182 8.7
183 26.2
184 17.6
185 22.6
186 10.3
187 17.3
188 15.9
189 6.7
190 10.8
191 9.9
192 5.9
193 19.6
194 17.3
195 7.6
196 9.7
197 12.8
198 25.5
199 13.4
Name: Sales, Length: 200, dtype: float64
C:Users87823.condaenvs ensorflowlibsite-packagessklearnmodel_selection\_split.py:2010: FutureWarning: From version 0.21, test_size will always complement train_size unless both are specified.
FutureWarning)
[ 1.00000000e-03 3.59381366e-03 1.29154967e-02 4.64158883e-02
1.66810054e-01 5.99484250e-01 2.15443469e+00 7.74263683e+00
2.78255940e+01 1.00000000e+02]
alpha_can =
[ 0.001 0.00359381 0.0129155 0.04641589 0.16681005
0.59948425 2.15443469 7.74263683 27.82559402 100. ]
超参数:
{'alpha': 7.7426368268112773}
order: 58 39
40 22
34 2
102 18
184 26
198 8
95 20
4 37
29 11
168 23
171 36
18 33
11 24
89 31
110 21
118 17
159 7
35 16
136 14
59 10
51 3
16 25
44 35
94 29
31 15
162 1
38 13
28 6
193 9
27 32
47 12
165 4
194 19
177 27
176 28
97 34
174 38
73 30
69 0
172 5
Name: Sales, dtype: int64
y_test_now =
[ 7.6 8.5 9.5 9.5 10.1 10.5 10.7 11. 11.3 11.5 11.5 11.7
11.9 11.9 12.5 12.8 12.9 12.9 13.4 14.5 14.8 14.9 15.5 15.9
15.9 16.6 16.7 16.9 17.1 17.3 17.4 17.6 18.4 18.9 19.6 20.2
22.3 23.2 23.8 25.5]
x_test_now =
[[ 19.6 20.1 17. ]
[ 25.1 25.7 43.3]
[ 95.7 1.4 7.4]
[ 25.6 39. 9.3]
[ 43.1 26.7 35.1]
[ 70.6 16. 40.8]
[ 100.4 9.6 3.6]
[ 129.4 5.7 31.3]
[ 69.2 20.5 18.3]
[ 107.4 14. 10.9]
[ 222.4 3.4 13.1]
[ 170.2 7.8 35.2]
[ 112.9 17.4 38.6]
[ 234.5 3.4 84.8]
[ 67.8 36.6 114. ]
[ 290.7 4.1 8.5]
[ 180.8 10.8 58.4]
[ 131.7 18.4 34.6]
[ 225.8 8.2 56.5]
[ 164.5 20.9 47.4]
[ 280.2 10.1 21.4]
[ 188.4 18.1 25.6]
[ 184.9 21. 22. ]
[ 240.1 16.7 22.9]
[ 125.7 36.9 79.2]
[ 202.5 22.3 31.6]
[ 109.8 47.8 51.4]
[ 163.3 31.6 52.9]
[ 215.4 23.6 57.6]
[ 149.7 35.6 6. ]
[ 214.7 24. 4. ]
[ 253.8 21.3 30. ]
[ 210.7 29.5 9.3]
[ 248.8 27.1 22.9]
[ 166.8 42. 3.6]
[ 248.4 30.2 20.3]
[ 216.8 43.9 27.2]
[ 239.9 41.5 18.5]
[ 210.8 49.6 37.7]
[ 283.6 42. 66.2]]
lasso_model.score(x_test,y_test): 0.892714279041
mse = 1.99274576769 rmse = 1.41164647405
三、图片
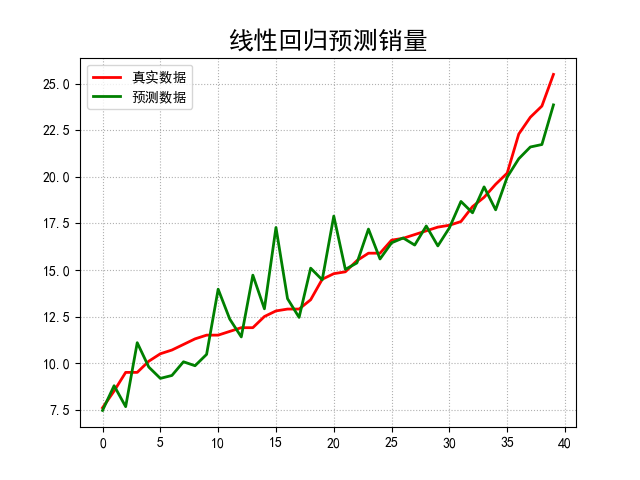