东南亚“美团” Grab 的搜索索引优化之法 https://mp.weixin.qq.com/s/O58PrtsG-qVsySxmPvrWrg
东南亚“美团” Grab 的搜索索引优化之法
Grab 是一家总部位于新加坡的东南亚网约车和送餐平台公司,业务遍及东南亚大部分地区,为 8 个国家的 350 多座城市的 1.87 亿多用户提供服务。Grab 当前提供包括网约车、送餐、酒店预订、网上银行、移动支付和保险服务。是东南亚的“美团”。Grab Engineering 分享了他们对搜索索引进行优化的方法与心得,InfoQ 中文站翻译并分享。
当今的应用程序通常使用各种数据库引擎,每个引擎服务于特定的需求。对于 Grab Deliveries,MySQL 数据库是用来存储典型数据格式的,而 Elasticsearch 则提供高级搜索功能。MySQL 是原始数据的主要数据存储,而 Elasticsearch 是派生存储。
搜索数据流
对于 MySQL 和 Elasticsearch 之间的数据同步进行了很多工作。本文介绍了如何优化增量搜索数据索引的一系列技术。
背 景从主数据存储到派生数据存储的数据同步是由数据同步平台(Data Synchronisation Platform,DSP)Food-Puxian 处理的。就搜索服务而言,它是 MySQL 和 Elasticsearch 之间的数据同步。
当 MySQL 的每一次实时数据更新时触发数据同步过程,它将向 Kafka 传递更新的数据。数据同步平台使用 Kafka 流列表,并在 Elasticsearch 中增量更新相应的搜索索引。此过程也称为增量同步。
Kafka 到数据同步平台利用 Kafka 流,数据同步平台实现增量同步。“流”是一种没有边界的、持续更新的数据集,它是有序的、可重放的和容错的。
利用 Kafaka 的数据同步过程
上图描述了使用 Kafka 进行数据同步的过程。数据生产器为 MySQL 上的每一个操作创建一个 Kafka 流,并实时将其发送到 Kafka。数据同步平台为每个 Kafka 流创建一个流消费器,消费器从各自的 Kafka 流中读取数据更新,并将其同步到 Elasticsearch。
MySQL 到 ElasticsearchElasticsearch 中的索引与 MySQL 表对应。MySQL 的数据存储在表中,而 Elasticsearch 的数据则存储在索引中。多个 MySQL 表被连接起来,形成一个 Elasticsearch 索引。以下代码段展示了 MySQL 和 Elasticsearch 中的实体 - 关系映射。实体 A 与实体 B 有一对多的关系。实体 A 在 MySQL 中有多个相关的表,即表 A1 和 A2,它们被连接成一个 Elasticsearch 索引 A。
MySQL 和 Elasticsearch 中的 ER 映射
有时,一个搜索索引同时包含实体 A 和实体 B。对于该索引的关键字搜索查询,例如“Burger”,实体 A 和实体 B 中名称包含“Burger”的对象都会在搜索响应中返回。
原始增量同步原始 Kafaka 流在上面所示的 ER 图中,数据生产器为每个 MySQL 表都会创建一个 Kafaka 流。每当 MySQL 发生插入、更新或删除操作时,执行操作之后的数据副本会被发送到其 Kafka 流中。对于每个 Kafaka 流,数据同步平台都会创建不同的流消费器(Stream Consumer),因为它们具有不同的数据结构。
流消费器基础设施流消费器由 3 个组件组成。
-
事件调度器(Event Dispatcher):监听并从 Kafka 流中获取事件,将它们推送到事件缓冲区,并启动一个 goroutine,在事件缓冲区中为不存在 ID 的每个事件运行事件处理器。
-
事件缓冲区(Event Buffer):事件通过主键(aID、bID 等)缓存在内存中。一个事件被缓存在缓冲区中,直到它被一个 goroutine 选中,或者当一个具有相同主键的新事件被推入缓冲区时被替换。
-
事件处理器(Event Handler):从事件缓冲区中读取事件,由事件调度器启动的 goroutine 会对其进行处理。
流消费器基础设施
事件缓冲区过程事件缓冲区由许多子缓冲区组成,每个子缓冲区具有一个唯一的 ID,该 ID 是缓冲区中事件的主键。一个子缓冲区的最大尺寸为 1。这样,事件缓冲区就可以重复处理缓冲区中具有相同 ID 的事件。
下图展示了将事件推送到事件缓冲区的过程。在将新事件推送到缓冲区时,将替换共享相同 ID 的旧事件。结果,被替换的事件不会被处理。
将事件推送到事件缓冲区
事件处理器过程下面的流程图显示了由事件处理器执行的程序。其中包括公共处理器流程(白色),以及针对对象 B 事件的附加过程(绿色)。当通过从数据库中加载的数据创建一个新的 Elasticsearch 文档时,它会从 Elasticsearch 获取原始文档,比较是否有更改字段,并决定是否需要向 Elasticsearch 发送新文档。
在处理对象 B 事件时,它还根据公共处理器级联更新到 Elasticsearch 索引中的相关对象 A。我们将这种操作命名为“级联更新”(Cascade Update)。
事件处理器执行的过程
原始基础设施存在的问题Elasticsearch 索引中的数据可以来自多个 MySQL 表,如下所示。
Elasticsearch 索引中的数据
原始基础设施存在一些问题。
-
繁重的数据库负载:消费器从 Kafka 流中读取数据,将流事件视为通知,然后使用 ID 从数据库中加载数据,创建新的 Elasticsearch 文档。流事件中的数据并没有得到很好的利用。每次从数据库加载数据,然后创建新的 Elasticsearch 文档,都会导致大量的数据库流量。数据库成为一个瓶颈。
-
数据丢失:生产器在应用程序代码中向 Kafka 发送数据副本。通过 MySQL 命令行工具(command-line tool,CLT)或其他数据库管理工具进行的数据更改会丢失。
-
与 MySQL 表结构的紧密耦合:如果生产器在 MySQL 中的现有表中添加了一个新的列,并且这个列需要同步到 Elasticsearch,那么数据同步平台就无法捕捉到这个列的数据变化,直到生产器进行代码修改并将这个列添加到相关的 Kafka 流中。
-
冗余的 Elasticsearch 更新:Elasticsearch 数据是 MySQL 数据的一个子集。生产器将数据发布到 Kafka 流中,即使对与 Elasticsearch 无关的字段进行了修改。这些与 Elasticsearch 无关的流事件仍会被拾取。
-
重复的级联更新:考虑一种情况,即搜索索引同时包含对象 A 和对象 B,在很短的时间内对对象 B 产生大量的更新。所有的更新将被级联到同时包含对象 A 和 B 的索引,这会为数据库带来大量流量。
MySQL 二进制日志(Binlog)是一组日志文件,其中包含对 MySQL 服务器实例进行的数据修改信息。它包含所有更新数据的语句。二进制日志有两种类型。
-
基于语句的日志记录:事件包含产生数据更改(插入、更新、删除)的 SQL 语句。
-
基于行的日志记录:事件描述了单个行的更改。
Grab Caspian 团队(Data Tech)构建了一个基于 MySQL 基于行的二进制日志的变更数据捕获(Change Data Capture,CDC)系统。它能够捕获所有 MySQL 表的所有数据修改。
当前 Kafaka 流二进制日志流事件定义是一种普通的数据结构,包含三个主要字段:Operation、PayloadBefore 和 PayloadAfter。Operation 的枚举是创建、删除和更新。Payload 是 JSON 字符串格式的数据。所有二进制日志流都遵循相同的流事件定义。利用二进制日志事件中的 PayloadBefore 和 PayloadAfter,在数据同步平台上对增量同步进行优化成为可能。
二进制日志流事件主要字段
流消费器优化事件处理器优化优化 1请记住,上面提到过 Elasticsearch 存在冗余更新问题,Elasticsearch 数据是 MySQL 数据的一个子集。第一个优化是通过检查 PayloadBefore 和 PayloadAfter 之间的不同字段是否位于 Elasticsearch 数据子集中,从而过滤掉无关的流事件。
二进制日志事件中的 Payload 是 JSON 字符串,所以定义了一个数据结构来解析 PayloadBefore 和 PayloadAfter,其中仅包含 Elasticsearch 数据中存在的字段。对比解析后的 Payload,我们很容易知道这个更改是否与 Elasticsearch 相关。
下图显示了经过优化的事件处理器流。从蓝色流程可以看出,在处理事件时,首先对 PayloadBefore 和 PayloadAfter 进行比较。仅在 PayloadBefore 和 PayloadAfter 之间存在差异时,才处理该事件。因为无关的事件已经被过滤掉,所以没有必要从 Elasticsearch 中获取原始文件。
事件处理器优化 1
成效-
没有数据丢失。使用 MySQL CLT 或其他数据库管理工具进行的更改可以被捕获。
-
对 MySQL 表的定义没有依赖性。所有的数据都是 JSON 字符串格式。
-
不存在多余的 Elasticsearch 更新和数据库读取。
-
Elasticsearch 读取流量减少 90%。
-
不再需要从 Elasticsearch 获取原始文档与新创建的文档进行比较。
-
过滤掉 55% 的不相关流事件。
-
数据库负载降低 55%。
针对优化 1 的 Elasticsearch 事件更新
优化 2事件中的 PayloadAfter 提供了更新的数据。因此,我们开始思考是否需要一种全新的从多个 MySQL 表读取的 Elasticsearch 文档。第二个优化是利用二进制日志事件的数据差异,改为部分更新。
下图展示了部分更新的事件处理程序流程。如红色流所示,没有为每个事件创建一个新的 Elasticsearch 文档,而是首先检查该文档是否存在。加入文档存在(大部分时间都存在),则在此事件中更改数据,只要 PayloadBefore 和 PayloadAfter 之间的比较就会更新到现有的 Elasticsearch 文档。
事件处理器优化 2
成效-
将大部分 Elasticsearch 相关事件更改为部分更新:使用流事件中的数据来更新 Elasticsearch。
-
Elasticsearch 负载减少:只将 Elasticsearch 发送修改的字段。
-
数据库负载减少:基于优化 1,数据库负载减少 80%。
在把新事件推送到事件缓冲区的时候,我们不会替换旧事件,而会把新事件和旧事件合并。
事件缓冲区中每个子缓冲区的尺寸为 1。在这种优化中,流事件不再被视为通知。我们使用事件中的 Payload 来执行部分更新。替换旧事件的旧过程已经不再适用于二进制日志流。
当事件调度器将一个新的事件推送到事件缓冲区的一个非空的子缓冲区时,它会将把子缓冲区中的事件 A 和新的事件 B 合并成一个新的二进制日志事件 C,其 PayloadBefore 来自事件 A,而 PayloadAfter 来自事件 B。
合并事件缓冲区优化的操作
级联更新优化优化我们使用一个新的流来处理级联更新事件。当生产器发送数据到 Kafka 流时,共享相同 ID 的数据将被存储在同一个分区上。每一个数据同步平台服务实例只有一个流消费器。在消费器消费 Kafaka 流时,一个分区仅由一个消费器消费。因此,共享相同 ID 的级联更新事件将由同一个 EC2 实例上的一个流消费器所消费。有了这种特殊的机制,内存中的事件缓冲区能够重复使用大部分共享相同 ID 的级联更新事件。
以下流程图展示了优化后的事件处理程序。绿色显示的是原始流,而紫色显示的是当前流,带有级联更新事件。在处理对象 B 的事件时,事件处理器不会直接级联更新相关对象 A,而是发送一个级联更新事件到新的流。这个新流的消费器将处理级联更新事件,并将对象 A 的数据同步到 Elasticsearch 中。
带有级联更新的事件处理器
成效-
级联更新事件消除了 80% 的重复数据。
-
级联更新引入的数据库负载减少。
级联更新事件
总 结本文介绍了四种不同的数据同步平台优化方法。在改用 Coban 团队提供的 MySQL 二进制日志流并对流消费器进行优化后,数据同步平台节省了约 91% 的数据库读取和 90% 的 Elasticsearch 读取,流消费器处理的流流量的平均查询次数(Queries Per Second,QPS)从 200 次增加到 800 次。高峰时段的平均查询次数最大可达到 1000 次以上。随着平均查询次数的提高,处理数据的时间和从 MySQL 到 Elasticsearch 的数据同步的延迟都有所减少。经过优化,数据同步平台的数据同步能力得到显著的提高。
原文链接:
https://engineering.grab.com/search-indexing-optimisation
Search indexing optimisation https://engineering.grab.com/search-indexing-optimisation
Modern applications commonly utilise various database engines, with each serving a specific need. At Grab Deliveries, MySQL database (DB) is utilised to store canonical forms of data, and Elasticsearch to provide advanced search capabilities. MySQL serves as the primary data storage for raw data, and Elasticsearch as the derived storage.
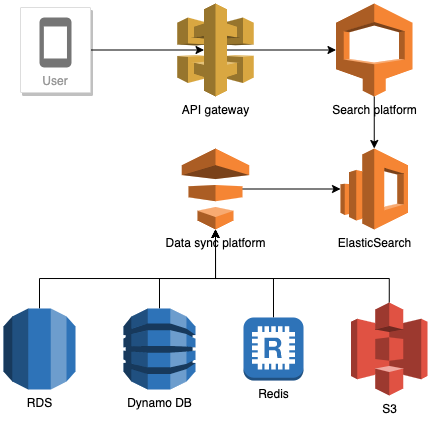
Efforts have been made to synchronise data between MySQL and Elasticsearch. In this post, a series of techniques will be introduced on how to optimise incremental search data indexing.
Background
The synchronisation of data from the primary data storage to the derived data storage is handled by Food-Puxian, a Data Synchronisation Platform (DSP). In a search service context, it is the synchronisation of data between MySQL and Elasticsearch.
The data synchronisation process is triggered on every real-time data update to MySQL, which will streamline the updated data to Kafka. DSP consumes the list of Kafka streams and incrementally updates the respective search indexes in Elasticsearch. This process is also known as Incremental Sync.
Kafka to DSP
DSP uses Kafka streams to implement Incremental Sync. A stream represents an unbounded, continuously updating data set, which is ordered, replayable and fault-tolerant.
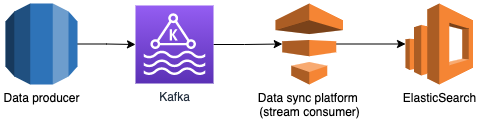
The above diagram depicts the process of data synchronisation using Kafka. The Data Producer creates a Kafka stream for every operation done on MySQL and sends it to Kafka in real-time. DSP creates a stream consumer for each Kafka stream and the consumer reads data updates from respective Kafka streams and synchronises them to Elasticsearch.
MySQL to Elasticsearch
Indexes in Elasticsearch correspond to tables in MySQL. MySQL data is stored in tables, while Elasticsearch data is stored in indexes. Multiple MySQL tables are joined to form an Elasticsearch index. The below snippet shows the Entity-Relationship mapping in MySQL and Elasticsearch. Entity A has a one-to-many relationship with entity B. Entity A has multiple associated tables in MySQL, table A1 and A2, and they are joined into a single Elasticsearch index A.
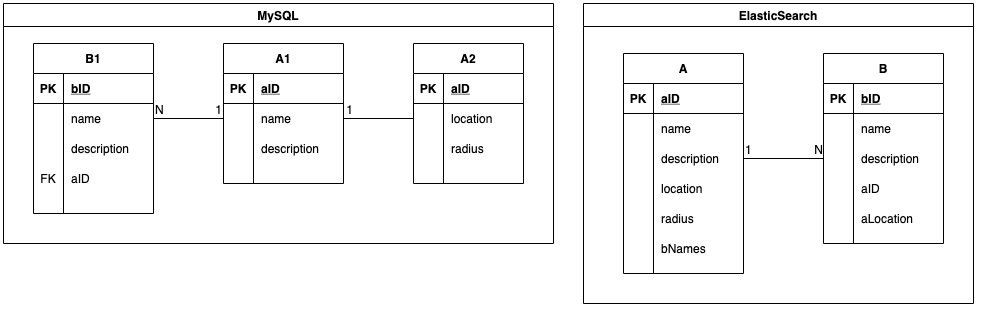
Sometimes a search index contains both entity A and entity B. In a keyword search query on this index, e.g. “Burger”, objects from both entity A and entity B whose name contains “Burger” are returned in the search response.
Original Incremental Sync
Original Kafka streams
The Data Producers create a Kafka stream for every MySQL table in the ER diagram above. Every time there is an insert, update, or delete operation on the MySQL tables, a copy of the data after the operation executes is sent to its Kafka stream. DSP creates different stream consumers for every Kafka stream since their data structures are different.
Stream Consumer infrastructure
Stream Consumer consists of 3 components.
- Event Dispatcher: Listens and fetches events from the Kafka stream, pushes them to the Event Buffer and starts a goroutine to run Event Handler for every event whose ID does not exist in the Event Buffer.
- Event Buffer: Caches events in memory by the primary key (aID, bID, etc). An event is cached in the Buffer until it is picked by a goroutine or replaced when a new event with the same primary key is pushed into the Buffer.
- Event Handler: Reads an event from the Event Buffer and the goroutine started by the Event Dispatcher handles it.
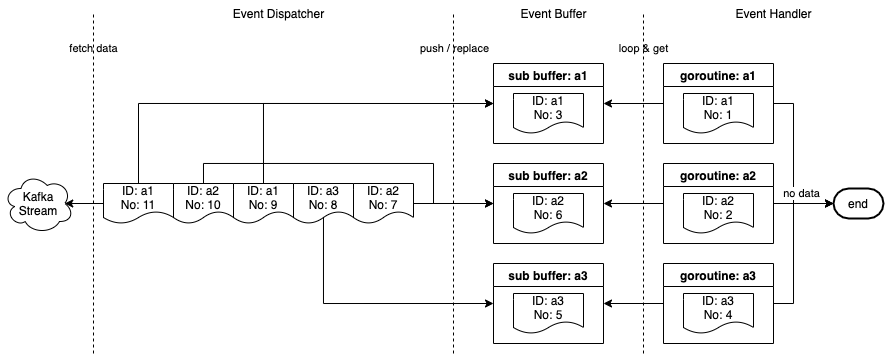
Event Buffer procedure
Event Buffer consists of many sub buffers, each with a unique ID which is the primary key of the event cached in it. The maximum size of a sub buffer is 1. This allows the Event Buffer to deduplicate events having the same ID in the buffer.
The below diagram shows the procedure of pushing an event to the Event Buffer. When a new event is pushed to the buffer, the old event sharing the same ID will be replaced. The replaced event is therefore not handled.
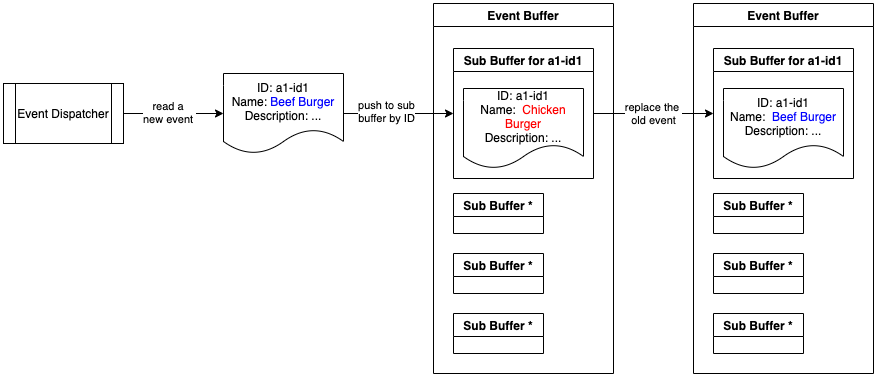
Event Handler procedure
The below flowchart shows the procedures executed by the Event Handler. It consists of the common handler flow (in white), and additional procedures for object B events (in green). After creating a new Elasticsearch document by data loaded from the database, it will get the original document from Elasticsearch to compare if any field is changed and decide whether it is necessary to send the new document to Elasticsearch.
When object B event is being handled, on top of the common handler flow, it also cascades the update to the related object A in the Elasticsearch index. We name this kind of operation Cascade Update.
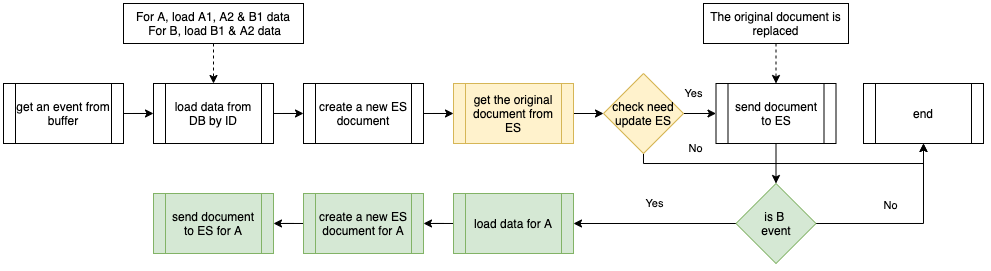
Issues in the original infrastructure
Data in an Elasticsearch index can come from multiple MySQL tables as shown below.
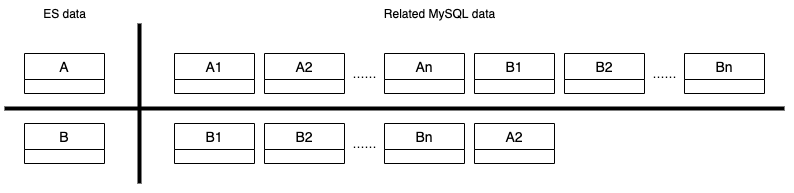
The original infrastructure came with a few issues.
- Heavy DB load: Consumers read from Kafka streams, treat stream events as notifications then use IDs to load data from the DB to create a new Elasticsearch document. Data in the stream events are not well utilised. Loading data from the DB every time to create a new Elasticsearch document results in heavy traffic to the DB. The DB becomes a bottleneck.
- Data loss: Producers send data copies to Kafka in application code. Data changes made via MySQL command-line tool (CLT) or other DB management tools are lost.
- Tight coupling with MySQL table structure: If producers add a new column to an existing table in MySQL and this column needs to be synchronised to Elasticsearch, DSP is not able to capture the data changes of this column until the producers make the code change and add the column to the related Kafka Stream.
- Redundant Elasticsearch updates: Elasticsearch data is a subset of MySQL data. Producers publish data to Kafka streams even if changes are made on fields that are not relevant to Elasticsearch. These stream events that are irrelevant to Elasticsearch would still be picked up.
- Duplicate cascade updates: Consider a case where the search index contains both object A and object B. A large number of updates to object B are created within a short span of time. All the updates will be cascaded to the index containing both objects A and B. This will bring heavy traffic to the DB.
Optimised Incremental Sync
MySQL Binlog
MySQL binary log (Binlog) is a set of log files that contain information about data modifications made to a MySQL server instance. It contains all statements that update data. There are two types of binary logging:
- Statement-based logging: Events contain SQL statements that produce data changes (inserts, updates, deletes).
- Row-based logging: Events describe changes to individual rows.
The Grab Caspian team (Data Tech) has built a Change Data Capture (CDC) system based on MySQL row-based Binlog. It captures all the data modifications made to MySQL tables.
Current Kafka streams
The Binlog stream event definition is a common data structure with three main fields: Operation, PayloadBefore and PayloadAfter. The Operation enums are Create, Delete, and Update. Payloads are the data in JSON string format. All Binlog streams follow the same stream event definition. Leveraging PayloadBefore and PayloadAfter in the Binlog event, optimisations of incremental sync on DSP becomes possible.
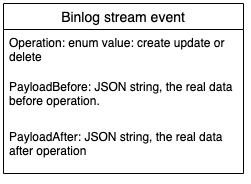
Stream Consumer optimisations
Event Handler optimisations
Optimisation 1
Remember that there was a redundant Elasticsearch updates issue mentioned above where the Elasticsearch data is a subset of the MySQL data. The first optimisation is to filter out irrelevant stream events by checking if the fields that are different between PayloadBefore and PayloadAfter are in the Elasticsearch data subset.
Since the payloads in the Binlog event are JSON strings, a data structure only with fields that are present in Elasticsearch data is defined to parse PayloadBefore and PayloadAfter. By comparing the parsed payloads, it is easy to know whether the change is relevant to Elasticsearch.
The below diagram shows the optimised Event Handler flows. As shown in the blue flow, when an event is handled, PayloadBefore and PayloadAfter are compared first. An event will be processed only if there is a difference between PayloadBefore and PayloadAfter. Since the irrelevant events are filtered, it is unnecessary to get the original document from Elasticsearch.
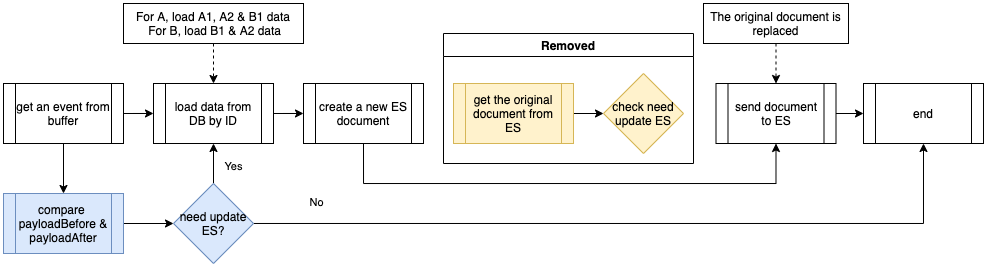
Achievements
- No data loss. Changes made via MySQL CLT or other DB manage tools can be captured.
- No dependency on MySQL table definition. All the data is in JSON string format.
- No redundant Elasticsearch updates and DB reads.
- Elasticsearch reads traffic reduced by 90%: Not a need to get the original document from Elasticsearch to compare with the newly created document anymore.
- 55% of irrelevant stream events are filtered out.
- The DB load is reduced by 55%
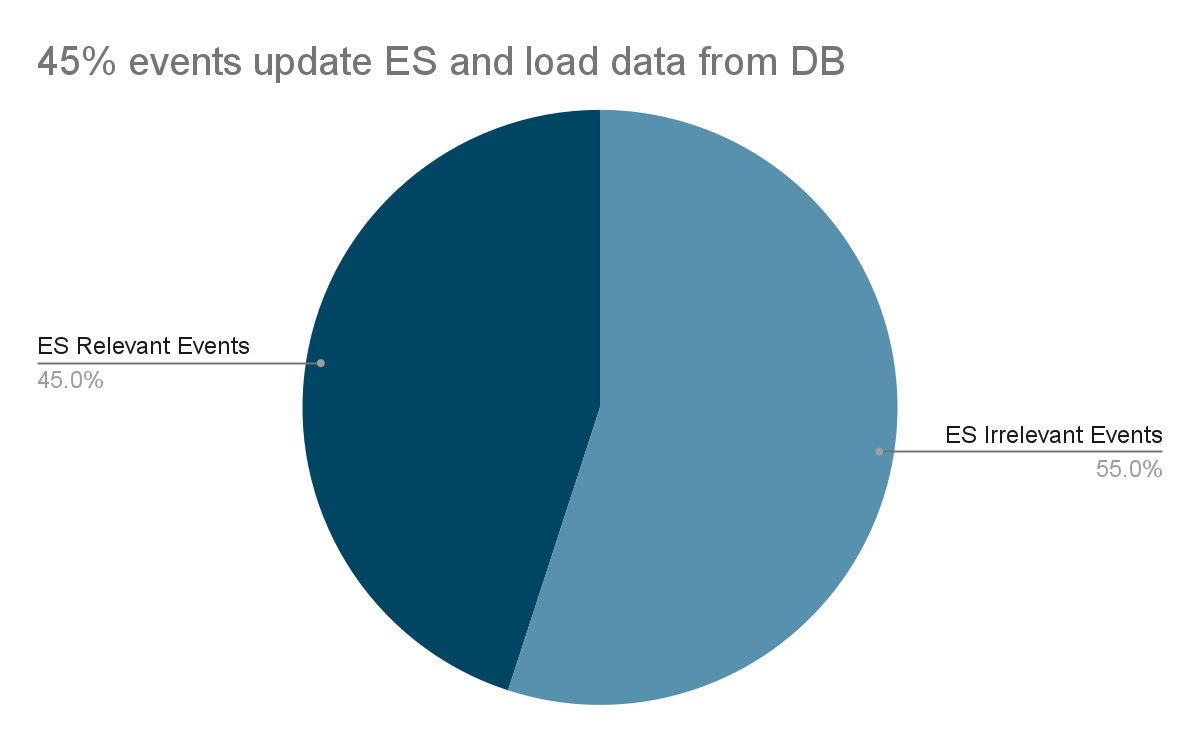
Optimisation 2
The PayloadAfter in the event provides updated data. This makes us think about whether a completely new Elasticsearch document is needed each time, with its data read from several MySQL tables. The second optimisation is to change to a partial update using data differences from the Binlog event.
The below diagram shows the Event Handler procedure flow with a partial update. As shown in the red flow, instead of creating a new Elasticsearch document for each event, a check on whether the document exists will be performed first. If the document exists, which happens for the majority of the time, the data is changed in this event, provided the comparison between PayloadBefore and PayloadAfter is updated to the existing Elasticsearch document.
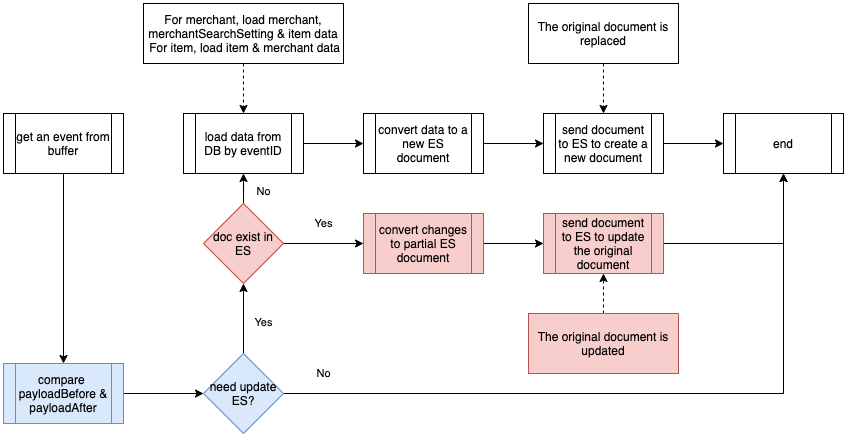
Achievements
- Change most Elasticsearch relevant events to partial update: Use data in stream events to update Elasticsearch.
- Elasticsearch load reduced: Only fields that have been changed will be sent to Elasticsearch.
- DB load reduced: DB load reduced by 80% based on Optimisation 1.
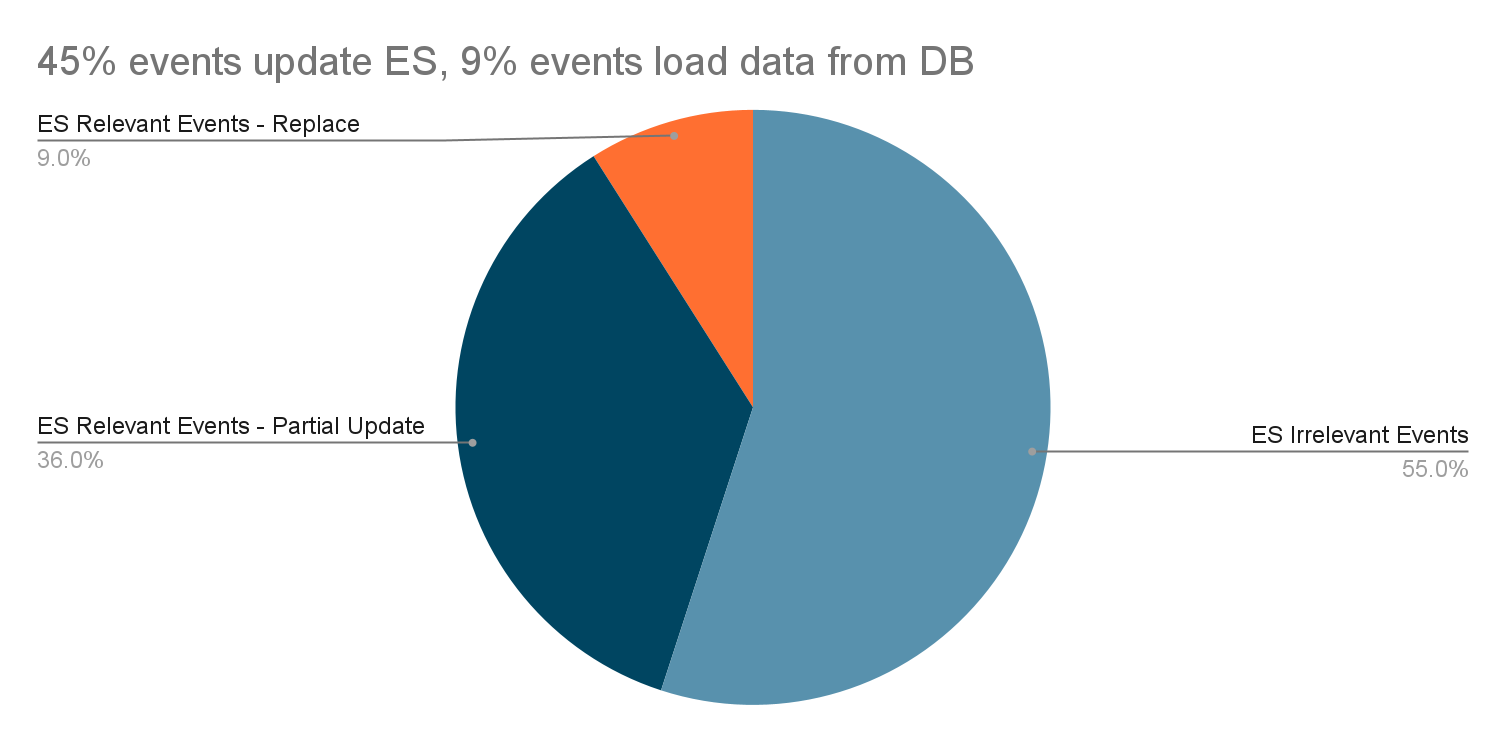
Event Buffer optimisation
Instead of replacing the old event, we merge the new event with the old event when the new event is pushed to the Event Buffer.
The size of each sub buffer in Event Buffer is 1. In this optimisation, the stream event is not treated as a notification anymore. We use the Payloads in the event to perform Partial Updates. The old procedure of replacing old events is no longer suitable for the Binlog stream.
When the Event Dispatcher pushes a new event to a non-empty sub buffer in the Event Buffer, it will merge event A in the sub buffer and the new event B into a new Binlog event C, whose PayloadBefore is from Event A and PayloadAfter is from Event B.
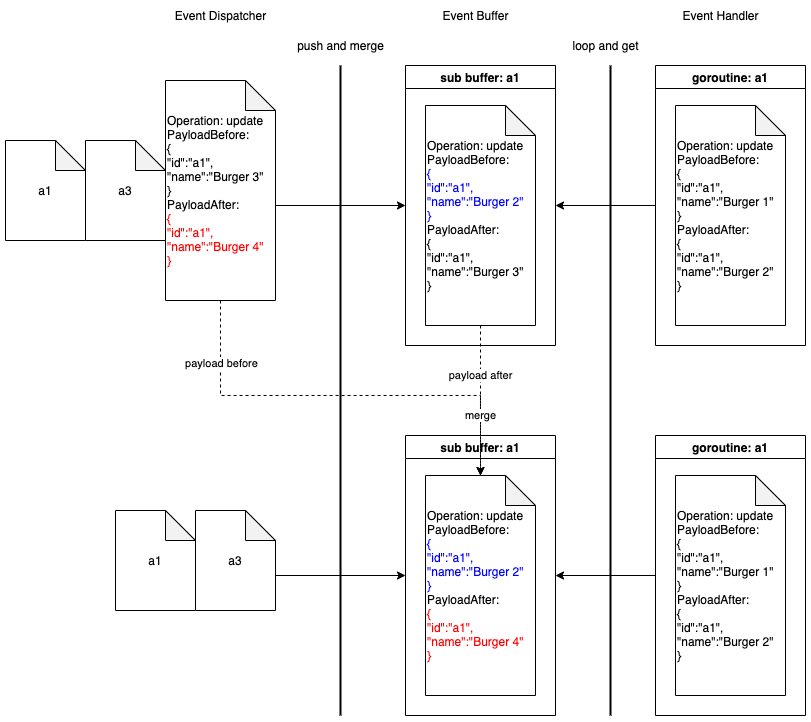
Cascade Update optimisation
Optimisation
We used a new stream to handle cascade update events. When the producer sends data to the Kafka stream, data sharing the same ID will be stored at the same partition. Every DSP service instance has only one stream consumer. When Kafka streams are consumed by consumers, one partition will be consumed by only one consumer. So the Cascade Update events sharing the same ID will be consumed by one stream consumer on the same EC2 instance. With this special mechanism, the in-memory Event Buffer is able to deduplicate most of the Cascade Update events sharing the same ID.
The flowchart below shows the optimised Event Handler procedure. Highlighted in green is the original flow while purple highlights the current flow with Cascade Update events. When handling an object B event, instead of cascading update the related object A directly, the Event Handler will send a Cascade Update event to the new stream. The consumer of the new stream will handle the Cascade Update event and synchronise the data of object A to the Elasticsearch.
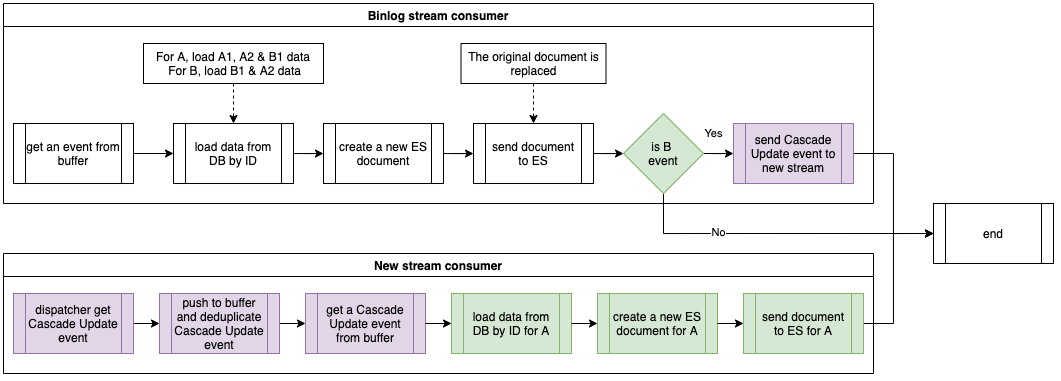
Achievements
- Cascade Update events deduplicated by 80%.
- DB load introduced by cascade update is reduced.
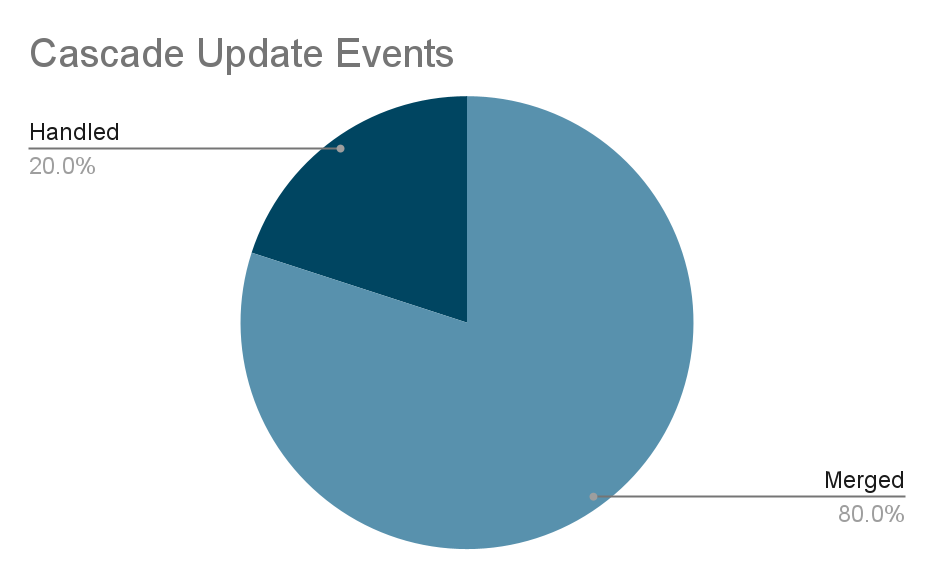
Summary
In this article four different DSP optimisations are explained. After switching to MySQL Binlog streams provided by the Coban team and optimising Stream Consumer, DSP has saved about 91% DB reads and 90% Elasticsearch reads, and the average queries per second (QPS) of stream traffic processed by Stream Consumer increased from 200 to 800. The max QPS at peak hours could go up to 1000+. With a higher QPS, the duration of processing data and the latency of synchronising data from MySQL to Elasticsearch was reduced. The data synchronisation ability of DSP has greatly improved after optimisation.
Special thanks to Jun Ying Lim and Amira Khazali for proofreading this article.