https://blog.csdn.net/lovelyaiq/article/details/79929393
https://blog.csdn.net/qq_29462849/article/details/85272575
Opencv调用深度学习模型
OpenCv 从V3.3版本开始支持调用深度学习模型,例如Caffe, Tensorflow, darknet等.详细见下图,具体的使用方法,可以参考官网:
https://docs.opencv.org/3.4.1/d6/d0f/group__dnn.html
目前Opencv可以支持的网络有GoogLeNet, ResNet-50,MobileNet-SSD from Caffe等,具体的可以参考:https://github.com/opencv/opencv/wiki/ChangeLog,里面有对dnn模块的详细介绍.
在github上,Opencv也有关于dnn模块的使用例子:https://github.com/opencv/opencv/tree/3.4.1/samples/dnn
这里只使用Python接口的Opencv 对Yolo V2(目前Opencv还不支持Yolo V3, 期待下一个版本支持)和Tensorflow训练出来的ssd_inception_v2_coco模型进行说明.
Yolo V2模型:
import cv2
import numpy as np
cap = cv2.VideoCapture('solidYellowLeft.mp4')
def read_cfg_model():
model_path = '/home/scyang/TiRan/WorkSpace/others/darknet/cfg/yolov2.weights'
cfg_path = '/home/scyang/TiRan/WorkSpace/others/darknet/cfg/yolov2.cfg'
yolo_net = cv2.dnn.readNet(model_path, cfg_path, 'darknet')
while True:
flag, img = cap.read()
if flag:
yolo_net.setInput(cv2.dnn.blobFromImage(img, 1.0/127.5, (416, 416), (127.5, 127.5, 127.5), False, False))
cvOut = yolo_net.forward()
for detection in cvOut:
confidence = np.max(detection[5:])
if confidence > 0:
classIndex = np.argwhere(detection == confidence)[0][0] - 5
x_center = detection[0] * cols
y_center = detection[1] * rows
width = detection[2] * cols
height = detection[3] * rows
start = (int(x_center - width/2), int(y_center - height/2))
end = (int(x_center + width/2), int(y_center + height/2))
cv2.rectangle(img,start, end , (23, 230, 210), thickness=2)
else:
break
cv2.imshow('show', img)
cv2.waitKey(10)
- 1
- 2
- 3
- 4
- 5
- 6
- 7
- 8
- 9
- 10
- 11
- 12
- 13
- 14
- 15
- 16
- 17
- 18
- 19
- 20
- 21
- 22
- 23
- 24
- 25
- 26
- 27
- 28
这里需要对cvOut的结果说明一下:cvOut的前4个表示检测到的矩形框信息,第5位表示背景,从第6位开始代表检测到的目标置信度及目标属于那个类。
因此,下面两处的作用是,从5位开始获取结果中目标的置信度及目标属于那个类。
confidence = np.max(detection[5:])
classIndex = np.argwhere(detection == confidence)[0][0] - 5
- 1
- 2
结果的截图如下:
Tensorflow模型
cvNet = cv2.dnn.readNetFromTensorflow('model/ssd_inception_v2_coco_2017_11_17.pb','model/ssd_inception_v2_coco_2017_11_17.pbtxt')
while True:
flag, img = cap.read()
if flag:
rows = img.shape[0]
cols = img.shape[1]
width = height = 300
image = cv2.resize(img, ((int(cols * height / rows), width)))
img = image[0:height, image.shape[1] - image.shape[1]]
cvNet.setInput(cv2.dnn.blobFromImage(img, 1.0/127.5, (300, 300), (127.5, 127.5, 127.5), swapRB=True, crop=False))
cvOut = cvNet.forward()
# Network produces output blob with a shape 1x1xNx7 where N is a number of
# detections and an every detection is a vector of values
# [batchId, classId, confidence, left, top, right, bottom]
for detection in cvOut[0,0,:,:]:
score = float(detection[2])
if score > 0.3:
rows = cols = 300
# print(detection)
left = detection[3] * cols
top = detection[4] * rows
right = detection[5] * cols
bottom = detection[6] * rows
cv2.rectangle(img, (int(left), int(top)), (int(right), int(bottom)), (23, 230, 210), thickness=2)
cv2.imshow('img', img)
cv2.waitKey(10)
else:
break
- 1
- 2
- 3
- 4
- 5
- 6
- 7
- 8
- 9
- 10
- 11
- 12
- 13
- 14
- 15
- 16
- 17
- 18
- 19
- 20
- 21
- 22
- 23
- 24
- 25
- 26
- 27
- 28
- 29
- 30
- 31
效果如下:
使用方法和Yolo的类似,从最终的效果可以看出,ssd_inception_v2模型要比V2好。
注:blobFromImage的详细介绍及使用方法,可以参考某大神的博客:https://www.pyimagesearch.com/2017/11/06/deep-learning-opencvs-blobfromimage-works/。这里就不在多述了,要学会站在巨人的肩膀上
OpenCV4.0 Mask RCNN 实例分割示例 C+/Python实现
点击我爱计算机视觉标星,更快获取cvmL新技术
前几天OpenCV4.0-Alpha发布,其中新增实例分割Mask RCNN模型是这次发布的亮点之一。
图像实例分割即将图像中目标检测出来并进行像素级分割。
昨天learnopencv.com博主Satya Mallick发表博文,详述了使用新版OpenCV加载TensorFlow Object Detection Model Zone中的Mask RCNN模型实现目标检测与实例分割的应用。使用C++/Python实现的代码示例,都开源了。
先来看看作者发布的结果视频:
从视频可以看出,2.5GHZ i7 处理器每帧推断时间大约几百到2000毫秒。
TensorFlow Object Detection Model Zone中现在有四个使用不同骨干网(InceptionV2, ResNet50, ResNet101 和 Inception-ResnetV2)的Mask RCNN模型,这些模型都是在MSCOCO 数据库上训练出来的,其中使用Inception的模型是这四个中最快的。Satya Mallick博文中正是使用了该模型。
Mask RCNN网络架构

OpenCV使用Mask RCNN目标检测与实例分割流程:
1)下载模型。
地址:
http://download.tensorflow.org/models/object_detection/
现有的四个模型:

2)参数初始化。
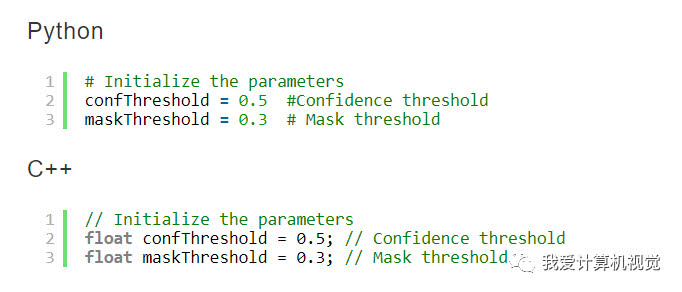
设置目标检测的置信度阈值和Mask二值化分割阈值。
3)加载Mask RCNN模型、类名称与可视化颜色值。
mscoco_labels.names包含MSCOCO所有标注对象的类名称。
colors.txt是在图像上标出某实例时其所属类显示的颜色值。
frozen_inference_graph.pb模型权重。
mask_rcnn_inception_v2_coco_2018_01_28.pbtxt文本图文件,告诉OpenCV如何加载模型权重。
OpenCV已经给定工具可以从给定模型权重提取出文本图文件。详见:
https://github.com/opencv/opencv/wiki/TensorFlow-Object-Detection-API

OpenCV支持CPU和OpenCL推断,但OpenCL只支持Intel自家GPU,Satya设置了CPU推断模式(cv.dnn.DNN_TARGET_CPU)。
4)读取图像、视频或者摄像头数据。
5)对每一帧数据计算处理。
主要步骤如图:

6)提取目标包围框和Mask,并绘制结果。
C++/Python代码下载:
https://github.com/spmallick/learnopencv/tree/master/Mask-RCNN
原博文地址:
https://www.learnopencv.com/deep-learning-based-object-detection-and-instance-segmentation-using-mask-r-cnn-in-opencv-python-c/
【点赞与转发】就是一种鼓励
C++调用mask rcnn进行实时检测--opencv4.0
介绍
Opencv在前面的几个版本中已经支持caffe、tensorflow、pytorch训练的几种模型,包括分类和物体检测模型(SSD、Yolo),针对tensorflow,opencv与tensorflow object detection api对接,可以通过该api训练模型,然后通过opencv调用,这样就可以把python下的环境移植到C++中。
关于tensorflow object detection api,后面博文会详细介绍
数据准备与环境配置
基于mask_rcnn_inception_v2_coco_2018_01_28的frozen_inference_graph.pb,这个模型在tensorflow object detection api中可以找到,然后需要对应的mask_rcnn_inception_v2_coco_2018_01_28.pbtxt,以及colors.txt,mscoco_labels.names。
opencv必须是刚发布的4.0版本,该版本支持mask rcnn和faster rcnn,低版本不支持哦,注意opencv4.0中在配置环境时,include下少了一个opencv文件夹,只有opencv2,这是正常的。
好了,废话不多说了,直接上源代码,该代码调用usb摄像头进行实时检测,基于单幅图像的检测修改下代码即可。
#include <fstream>
#include <sstream>
#include <iostream>
#include <string.h>
#include <opencv2/dnn.hpp>
#include <opencv2/imgproc.hpp>
#include <opencv2/highgui.hpp>
using namespace cv;
using namespace dnn;
using namespace std;
// Initialize the parameters
float confThreshold = 0.5; // Confidence threshold
float maskThreshold = 0.3; // Mask threshold
vector<string> classes;
vector<Scalar> colors;
// Draw the predicted bounding box
void drawBox(Mat& frame, int classId, float conf, Rect box, Mat& objectMask);
// Postprocess the neural network's output for each frame
void postprocess(Mat& frame, const vector<Mat>& outs);
int main()
{
// Load names of classes
string classesFile = "./mask_rcnn_inception_v2_coco_2018_01_28/mscoco_labels.names";
ifstream ifs(classesFile.c_str());
string line;
while (getline(ifs, line)) classes.push_back(line);
// Load the colors
string colorsFile = "./mask_rcnn_inception_v2_coco_2018_01_28/colors.txt";
ifstream colorFptr(colorsFile.c_str());
while (getline(colorFptr, line))
{
char* pEnd;
double r, g, b;
r = strtod(line.c_str(), &pEnd);
g = strtod(pEnd, NULL);
b = strtod(pEnd, NULL);
Scalar color = Scalar(r, g, b, 255.0);
colors.push_back(Scalar(r, g, b, 255.0));
}
// Give the configuration and weight files for the model
String textGraph = "./mask_rcnn_inception_v2_coco_2018_01_28/mask_rcnn_inception_v2_coco_2018_01_28.pbtxt";
String modelWeights = "./mask_rcnn_inception_v2_coco_2018_01_28/frozen_inference_graph.pb";
// Load the network
Net net = readNetFromTensorflow(modelWeights, textGraph);
net.setPreferableBackend(DNN_BACKEND_OPENCV);
net.setPreferableTarget(DNN_TARGET_CPU);
// Open a video file or an image file or a camera stream.
string str, outputFile;
VideoCapture cap(0);//根据摄像头端口id不同,修改下即可
//VideoWriter video;
Mat frame, blob;
// Create a window
static const string kWinName = "Deep learning object detection in OpenCV";
namedWindow(kWinName, WINDOW_NORMAL);
// Process frames.
while (waitKey(1) < 0)
{
// get frame from the video
cap >> frame;
// Stop the program if reached end of video
if (frame.empty())
{
cout << "Done processing !!!" << endl;
cout << "Output file is stored as " << outputFile << endl;
waitKey(3000);
break;
}
// Create a 4D blob from a frame.
blobFromImage(frame, blob, 1.0, Size(frame.cols, frame.rows), Scalar(), true, false);
//blobFromImage(frame, blob);
//Sets the input to the network
net.setInput(blob);
// Runs the forward pass to get output from the output layers
std::vector<String> outNames(2);
outNames[0] = "detection_out_final";
outNames[1] = "detection_masks";
vector<Mat> outs;
net.forward(outs, outNames);
// Extract the bounding box and mask for each of the detected objects
postprocess(frame, outs);
// Put efficiency information. The function getPerfProfile returns the overall time for inference(t) and the timings for each of the layers(in layersTimes)
vector<double> layersTimes;
double freq = getTickFrequency() / 1000;
double t = net.getPerfProfile(layersTimes) / freq;
string label = format("Mask-RCNN on 2.5 GHz Intel Core i7 CPU, Inference time for a frame : %0.0f ms", t);
putText(frame, label, Point(0, 15), FONT_HERSHEY_SIMPLEX, 0.5, Scalar(0, 0, 0));
// Write the frame with the detection boxes
Mat detectedFrame;
frame.convertTo(detectedFrame, CV_8U);
imshow(kWinName, frame);
}
cap.release();
return 0;
}
// For each frame, extract the bounding box and mask for each detected object
void postprocess(Mat& frame, const vector<Mat>& outs)
{
Mat outDetections = outs[0];
Mat outMasks = outs[1];
// Output size of masks is NxCxHxW where
// N - number of detected boxes
// C - number of classes (excluding background)
// HxW - segmentation shape
const int numDetections = outDetections.size[2];
const int numClasses = outMasks.size[1];
outDetections = outDetections.reshape(1, outDetections.total() / 7);
for (int i = 0; i < numDetections; ++i)
{
float score = outDetections.at<float>(i, 2);
if (score > confThreshold)
{
// Extract the bounding box
int classId = static_cast<int>(outDetections.at<float>(i, 1));
int left = static_cast<int>(frame.cols * outDetections.at<float>(i, 3));
int top = static_cast<int>(frame.rows * outDetections.at<float>(i, 4));
int right = static_cast<int>(frame.cols * outDetections.at<float>(i, 5));
int bottom = static_cast<int>(frame.rows * outDetections.at<float>(i, 6));
left = max(0, min(left, frame.cols - 1));
top = max(0, min(top, frame.rows - 1));
right = max(0, min(right, frame.cols - 1));
bottom = max(0, min(bottom, frame.rows - 1));
Rect box = Rect(left, top, right - left + 1, bottom - top + 1);
// Extract the mask for the object
Mat objectMask(outMasks.size[2], outMasks.size[3], CV_32F, outMasks.ptr<float>(i, classId));
// Draw bounding box, colorize and show the mask on the image
drawBox(frame, classId, score, box, objectMask);
}
}
}
// Draw the predicted bounding box, colorize and show the mask on the image
void drawBox(Mat& frame, int classId, float conf, Rect box, Mat& objectMask)
{
//Draw a rectangle displaying the bounding box
rectangle(frame, Point(box.x, box.y), Point(box.x + box.width, box.y + box.height), Scalar(255, 178, 50), 3);
//Get the label for the class name and its confidence
string label = format("%.2f", conf);
if (!classes.empty())
{
CV_Assert(classId < (int)classes.size());
label = classes[classId] + ":" + label;
}
//Display the label at the top of the bounding box
int baseLine;
Size labelSize = getTextSize(label, FONT_HERSHEY_SIMPLEX, 0.5, 1, &baseLine);
box.y = max(box.y, labelSize.height);
rectangle(frame, Point(box.x, box.y - round(1.5*labelSize.height)), Point(box.x + round(1.5*labelSize.width), box.y + baseLine), Scalar(255, 255, 255), FILLED);
putText(frame, label, Point(box.x, box.y), FONT_HERSHEY_SIMPLEX, 0.75, Scalar(0, 0, 0), 1);
Scalar color = colors[classId%colors.size()];
// Resize the mask, threshold, color and apply it on the image
resize(objectMask, objectMask, Size(box.width, box.height));
Mat mask = (objectMask > maskThreshold);
Mat coloredRoi = (0.3 * color + 0.7 * frame(box));
coloredRoi.convertTo(coloredRoi, CV_8UC3);
// Draw the contours on the image
vector<Mat> contours;
Mat hierarchy;
mask.convertTo(mask, CV_8U);
findContours(mask, contours, hierarchy, RETR_CCOMP, CHAIN_APPROX_SIMPLE);
drawContours(coloredRoi, contours, -1, color, 5, LINE_8, hierarchy, 100);
coloredRoi.copyTo(frame(box), mask);
}
- 1
- 2
- 3
- 4
- 5
- 6
- 7
- 8
- 9
- 10
- 11
- 12
- 13
- 14
- 15
- 16
- 17
- 18
- 19
- 20
- 21
- 22
- 23
- 24
- 25
- 26
- 27
- 28
- 29
- 30
- 31
- 32
- 33
- 34
- 35
- 36
- 37
- 38
- 39
- 40
- 41
- 42
- 43
- 44
- 45
- 46
- 47
- 48
- 49
- 50
- 51
- 52
- 53
- 54
- 55
- 56
- 57
- 58
- 59
- 60
- 61
- 62
- 63
- 64
- 65
- 66
- 67
- 68
- 69
- 70
- 71
- 72
- 73
- 74
- 75
- 76
- 77
- 78
- 79
- 80
- 81
- 82
- 83
- 84
- 85
- 86
- 87
- 88
- 89
- 90
- 91
- 92
- 93
- 94
- 95
- 96
- 97
- 98
- 99
- 100
- 101
- 102
- 103
- 104
- 105
- 106
- 107
- 108
- 109
- 110
- 111
- 112
- 113
- 114
- 115
- 116
- 117
- 118
- 119
- 120
- 121
- 122
- 123
- 124
- 125
- 126
- 127
- 128
- 129
- 130
- 131
- 132
- 133
- 134
- 135
- 136
- 137
- 138
- 139
- 140
- 141
- 142
- 143
- 144
- 145
- 146
- 147
- 148
- 149
- 150
- 151
- 152
- 153
- 154
- 155
- 156
- 157
- 158
- 159
- 160
- 161
- 162
- 163
- 164
- 165
- 166
- 167
- 168
- 169
- 170
- 171
- 172
- 173
- 174
- 175
- 176
- 177
- 178
- 179
- 180
- 181
- 182
- 183
- 184
- 185
- 186
- 187
- 188
- 189
- 190
- 191
- 192
- 193
- 194
- 195
- 196
- 197
- 198
- 199
实验结果
不过检测速度很慢,I7-8700k,GTX1060下需要1s每帧,达不到实时性要求。。。
实验数据
本博文所有的数据可以从这里下载:opencv调用mask rcnn数据