

!mkdir '/content/gdrive/My Drive/movie review'
!mkdir '/content/gdrive/My Drive/movie review/good/'
!mkdir '/content/gdrive/My Drive/movie review/bad/'
from utils import *
import tensorflow as tf
import sklearn
from sklearn import datasets
from sklearn.model_selection import train_test_split
import time
import re
import numpy as np
def clearstring(string):
#只选择包含字母和数字的字符串
string = re.sub('[^A-Za-z0-9]+', ' ', string)
#把句子分割成多个单词合成的队列
string = string.split(' ')
string = filter(None, string)
#消除单词首尾空格
string = [y.strip() for y in string]
string = ' '.join(string)
return string.lower()
def seperate_dataset(trainset, ratio=0.5):
'''
把文本中语句一条条分隔开,并打上标签
'''
text = []
label = []
for i in range(int(len(trainset.data) * ratio)):
#把文本分割成多个句子
data_ = trainset.data[i].split('
')
#过滤掉空行
data_ = list(filter(None, data_))
for n in range(len(data_)):
#去掉句子中不符合规则的单词
data_[n] = clearstring(data_[n])
text += data_
for n in range(len(data_)):
#打上标签,因为目录下只有两个文件夹因此只有两种标签
label.append(trainset.target[i])
return text, label
s = ' this is 98 !@# *q'
s = clearstring(s)
print(s)
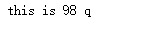
path = '/content/gdrive/My Drive/movie review'
trainset = sklearn.datasets.load_files(container_path = path, encoding = 'UTF-8')
'''
将文本中的句子抽取出来,形成两个集合,由于文件夹下只有两个子文件夹
因此它们对应两个标签
'''
trainset.data, trainset.target = seperate_dataset(trainset, 1.0)
print(trainset.target_names)
print('training data has {0} items'.format(len(trainset.data)))

#为每条记录附带两个标志位
onehot = np.zeros((len(trainset.data), len(trainset.target_names)))
#属于bad目录下的句子对应标签[1,0],属于good目录下的句子附带标签[0,1]
onehot[np.arange(len(trainset.data)), trainset.target] = 1.0
'''
将trainset.data, trianset.target, onehot三个数组以8:2的方式分成两部分
一部分用于测试
'''
train_X, test_X, train_Y, test_Y, train_onehot,test_onehot = train_test_split(trainset.data, trainset.target, onehot, test_size = 0.2)
concat = ' '.join(trainset.data).split()
vocabulary_size = len(list(set(concat)))
print(vocabulary_size)
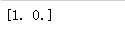
import collections
def build_dataset(words, n_words):
'''
将文本中的单词拆解成字典
'''
count = [['GO', 0], ['PAD', 1], ['EOS', 2], ['UNK', 3]]
#统计每个单词的出现次数,我们只选出现次数在前n_words范围内的单词
count.extend(collections.Counter(words).most_common(n_words - 1))
dictionary = dict()
for word, _ in count:
#给每个单词进行编号,编号会从4开始
dictionary[word] = len(dictionary)
data = list()
unk_count = 0
for word in words:
index = dictionary.get(word, 0)
#统计没有被选入范围的单词
if index == 0:
unk_count += 1
data.append(index)
count[0][1] = unk_count
#把编号和单词对应关系对换
reversed_dictionary = dict(zip(dictionary.values(), dictionary.keys()))
return data, count, dictionary, reversed_dictionary
data, count, dictionary, rev_dictionary = build_dataset(concat, vocabulary_size)
print('Most common words', count[4:10])
print('Sample data', data[:10], [rev_dictionary[i] for i in data[:10]])
'''
网络分两层,第一层128个节点,第二层也是128个节点,
'''
size_layer = 128
num_layers = 2
embedded_size = 128
dimension_output = len(trainset.target_names)
learning_rate = 1e-3
maxlen = 50
batch_size = 128
class RNN:
def __init__(self, size_layer, num_layer, embedded_size, dict_size,
dimension_output, learning_rate):
def cells(reuse = False):
'''
tensorflow封装了RNN节点,它跟我们前面描述的能在内部记录当前输入数据处理信息,
并将信息传递到下一次数据处理的R节点一样
'''
return tf.nn.rnn_cell.BasicRNNCell(size_layer, reuse=reuse)
#定义输入数据变量
self.X = tf.placeholder(tf.int32, [None, None])
#定义输出数据变量
self.Y = tf.placeholder(tf.float32, [None, dimension_output])
'''
定义embedding层,这一层与我们前面讲单词向量训练时提到过的网络第一层一样,它对应
一个二维矩阵,矩阵的每一行表示单词向量,self.X是one-hont-vector,它会将矩阵的某一行
挑选出来
'''
embeddings = tf.Variable(tf.random_uniform([dict_size, embedded_size], -1, 1))
embedded = tf.nn.embedding_lookup(embeddings, self.X)
#串联两个RNN节点增强识别能力
rnn_cells = tf.nn.rnn_cell.MultiRNNCell([cells() for _ in range(num_layers)])
#根据输入数据长度构造相应数量RNN节点,形成RNN网络层
outputs, _ = tf.nn.dynamic_rnn(rnn_cells, embedded, dtype=tf.float32)
#将RNN网络层输出的含有128个分量的向量转换为只有2个分量的向量
W = tf.get_variable('w', shape=(size_layer, dimension_output),
initializer=tf.orthogonal_initializer())
b = tf.get_variable('b', shape=(dimension_output), initializer=tf.zeros_initializer())
#将两个分量重,数值较大的那个当做当前语句所属分类
self.logits = tf.matmul(outputs[:, -1], W) + b
self.cost = tf.reduce_mean(tf.nn.softmax_cross_entropy_with_logits(logits=self.logits,
labels=self.Y))
self.optimizer = tf.train.AdamOptimizer(learning_rate=learning_rate).minimize(self.cost)
correct_pred = tf.equal(tf.argmax(self.logits, 1), tf.argmax(self.Y, 1))
self.accuracy = tf.reduce_mean(tf.cast(correct_pred, tf.float32))
'''
网络分两层,第一层128个节点,第二层也是128个节点,
'''
size_layer = 128
num_layers = 2
embedded_size = 128
dimension_output = len(trainset.target_names)
learning_rate = 1e-3
maxlen = 50
batch_size = 128
class RNN:
def __init__(self, size_layer, num_layer, embedded_size, dict_size,
dimension_output, learning_rate):
def cells(reuse = False):
'''
tensorflow封装了RNN节点,它跟我们前面描述的能在内部记录当前输入数据处理信息,
并将信息传递到下一次数据处理的R节点一样
'''
return tf.nn.rnn_cell.LSTMCell(size_layer, reuse=reuse)
#定义输入数据变量
self.X = tf.placeholder(tf.int32, [None, None])
#定义输出数据变量
self.Y = tf.placeholder(tf.float32, [None, dimension_output])
'''
定义embedding层,这一层与我们前面讲单词向量训练时提到过的网络第一层一样,它对应
一个二维矩阵,矩阵的每一行表示单词向量,self.X是one-hont-vector,它会将矩阵的某一行
挑选出来
'''
embeddings = tf.Variable(tf.random_uniform([dict_size, embedded_size], -1, 1))
embedded = tf.nn.embedding_lookup(embeddings, self.X)
#串联两个RNN节点增强识别能力
rnn_cells = tf.nn.rnn_cell.MultiRNNCell([cells() for _ in range(num_layers)])
#根据输入数据长度构造相应数量RNN节点,形成RNN网络层
outputs, _ = tf.nn.dynamic_rnn(rnn_cells, embedded, dtype=tf.float32)
#将RNN网络层输出的含有128个分量的向量转换为只有2个分量的向量
W = tf.get_variable('w', shape=(size_layer, dimension_output),
initializer=tf.orthogonal_initializer())
b = tf.get_variable('b', shape=(dimension_output), initializer=tf.zeros_initializer())
#将两个分量重,数值较大的那个当做当前语句所属分类
self.logits = tf.matmul(outputs[:, -1], W) + b
self.cost = tf.reduce_mean(tf.nn.softmax_cross_entropy_with_logits(logits=self.logits,
labels=self.Y))
self.optimizer = tf.train.AdamOptimizer(learning_rate=learning_rate).minimize(self.cost)
correct_pred = tf.equal(tf.argmax(self.logits, 1), tf.argmax(self.Y, 1))
self.accuracy = tf.reduce_mean(tf.cast(correct_pred, tf.float32))

'''
将输入语句中的单词转换成对应编号
'''
def word_to_index(corpus, dic, maxlen, UNK=3):
X = np.zeros((len(corpus), maxlen))
for i in range(len(corpus)):
'''
规定一句话单词量不能超过maxlen,超过了就截断。然后从最后一个单词开始,到第一个单词,
将每个单词转换为对应编号
'''
for no, k in enumerate(corpus[i].split()[:maxlen][::-1]):
try:
X[i, -1 - no] = dic[k]
except:
X[i, -1 - no] = UNK
return X
s = []
s.append("the rock is destined to be")
x = word_to_index(s, dictionary, maxlen)
print(x)

import time
tf.reset_default_graph()
sess = tf.InteractiveSession()
rnn = RNN(size_layer, num_layers, embedded_size, vocabulary_size+4, dimension_output,
learning_rate)
sess.run(tf.global_variables_initializer())
saver = tf.train.Saver(tf.global_variables(), max_to_keep=2)
checkpint_dir = '/content/gdrive/My Drive/dataset/checkpoints_basci_rnn'
EARLY_STOPPING, CURRENT_CHECKPOINT, CURRENT_ACC, EPOCH = 30, 0, 0, 0
while True:
lasttime = time.time()
if CURRENT_CHECKPOINT == EARLY_STOPPING:
print('break epoch: %d
' % (EPOCH))
break
train_acc, train_loss, test_acc,test_loss = 0,0,0,0
#训练网络
for i in range(0, (len(train_X) // batch_size) * batch_size, batch_size):
batch_x = word_to_index(train_X[i : i + batch_size], dictionary, maxlen)
acc, loss, _ = sess.run([rnn.accuracy, rnn.cost, rnn.optimizer],
feed_dict = {rnn.X: batch_x,
rnn.Y: train_onehot[i : i + batch_size]})
train_loss += loss
train_acc += acc
#检测训练结果
for i in range(0, (len(test_X) // batch_size) * batch_size, batch_size):
batch_x = word_to_index(test_X[i : i + batch_size], dictionary, batch_size)
acc, loss = sess.run([rnn.accuracy, rnn.cost],
feed_dict = {rnn.X : batch_x,
rnn.Y : test_onehot[i : i + batch_size]})
test_loss += loss
test_acc += acc
train_loss /= (len(train_X) // batch_size)
train_acc /= (len(train_X) // batch_size)
test_loss /= (len(test_X) // batch_size)
test_acc /= (len(test_X) // batch_size)
if test_acc > CURRENT_ACC:
print('epoch: %d, pass acc: %f, current acc %f' % (EPOCH, CURRENT_ACC, test_acc))
CURRENT_ACC = test_acc
CURRENT_CHECKPOINT = 0
else:
CURRENT_CHECKPOINT += 1
print('time taken: ', time.time() - lasttime)
print('epoch: %d, training loss: %f, training acc: %f, valid loss: %f, valid acc: %f
' % (EPOCH,
train_loss,
train_acc, test_loss,
test_acc))
path = saver.save(sess, checkpint_dir, global_step = EPOCH)
EPOCH += 1
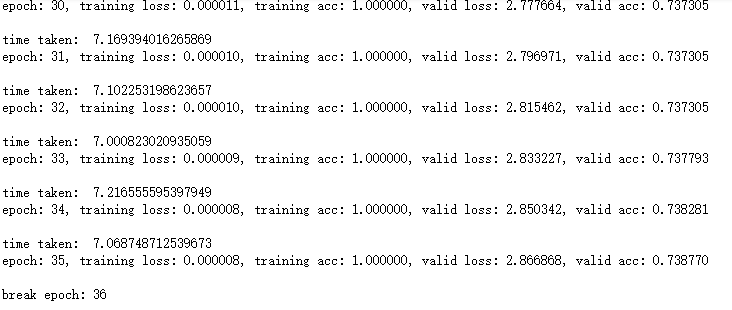