这篇博客主要是整理了PointNet提出者祁芮中台介绍PointNet、PointNet++、Frustum PointNets的PPT内容,内容包括如何将点云进行深度学习,如何设计新型的网络架构,如何将架构应用的3D场景理解。
作者主页:https://stanford.edu/~rqi/
B站视频:https://www.bilibili.com/s/video/BV1HE411g7tA
PPT下载链接:https://pan.baidu.com/s/105MRbBmCv4Tj6GYTMbS67w 提取码:z930
参考:https://www.cnblogs.com/Libo-Master/p/9759130.html
该PPT中涉及到的文献有:
PointNet: Deep Learning on Point Sets for 3D Classification and Segmentation
PointNet++: Deep Hierarchical Feature Learning on Point Sets in a Metric Space
Frustum PointNets:Frustum PointNets for 3D Object Detection from RGB-D Data
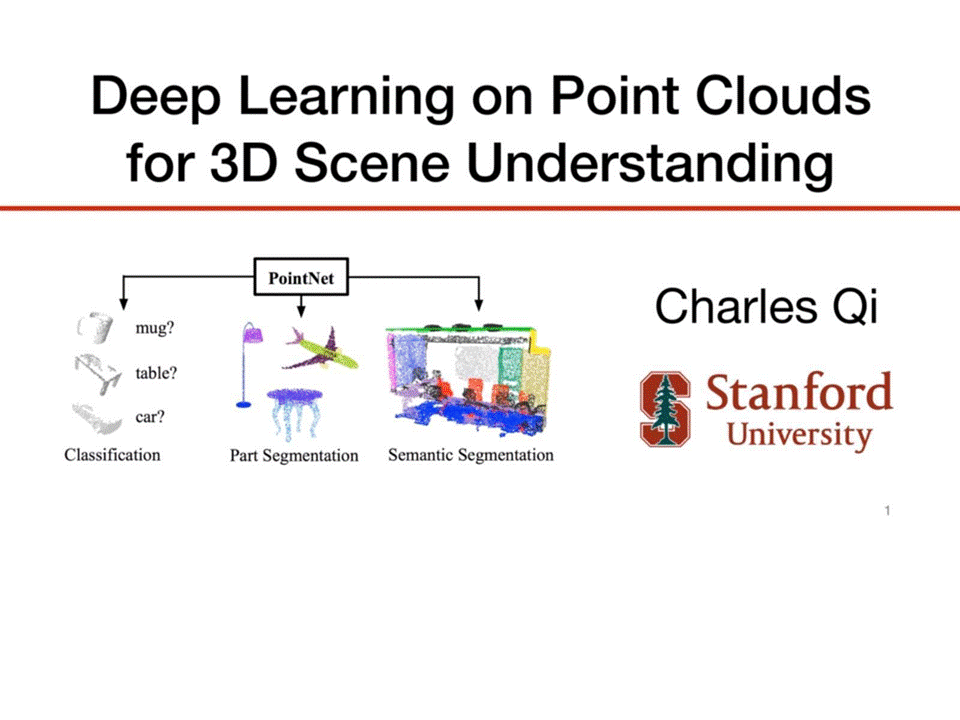
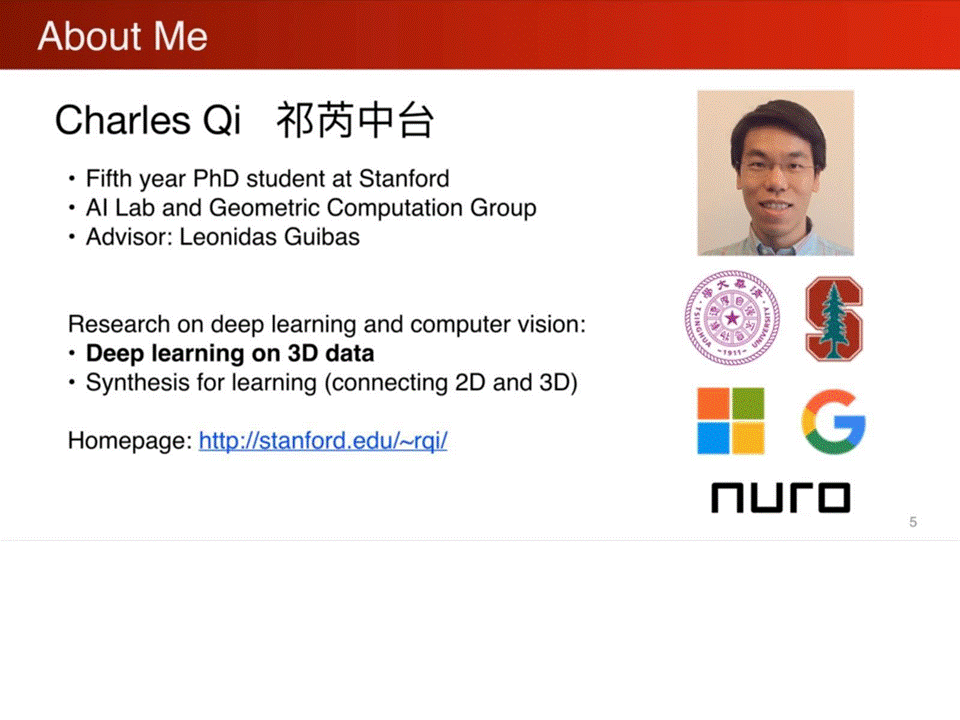
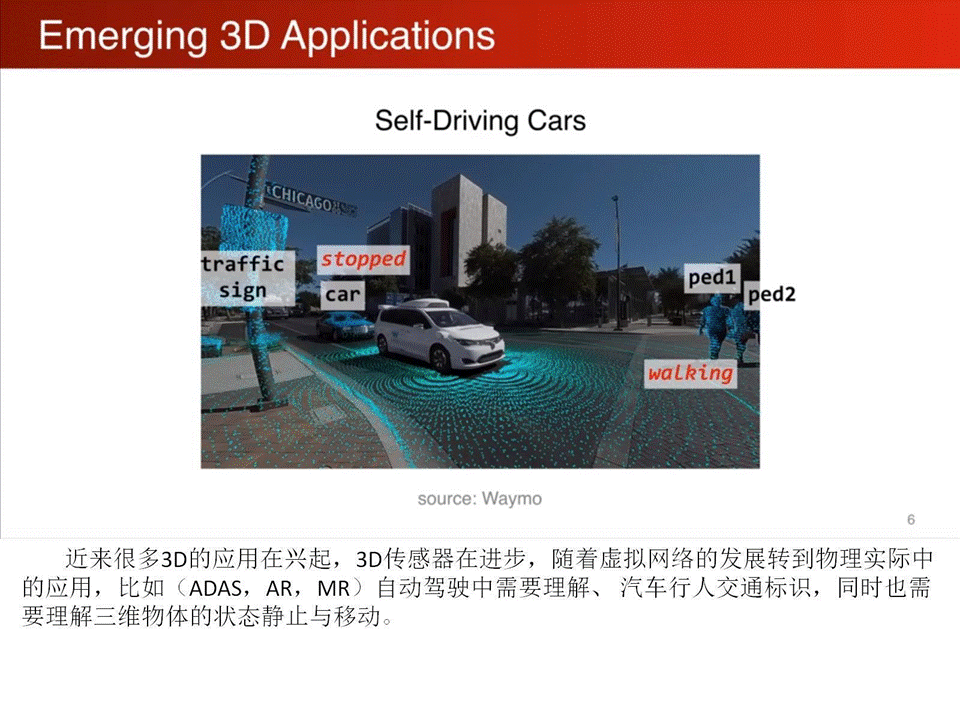
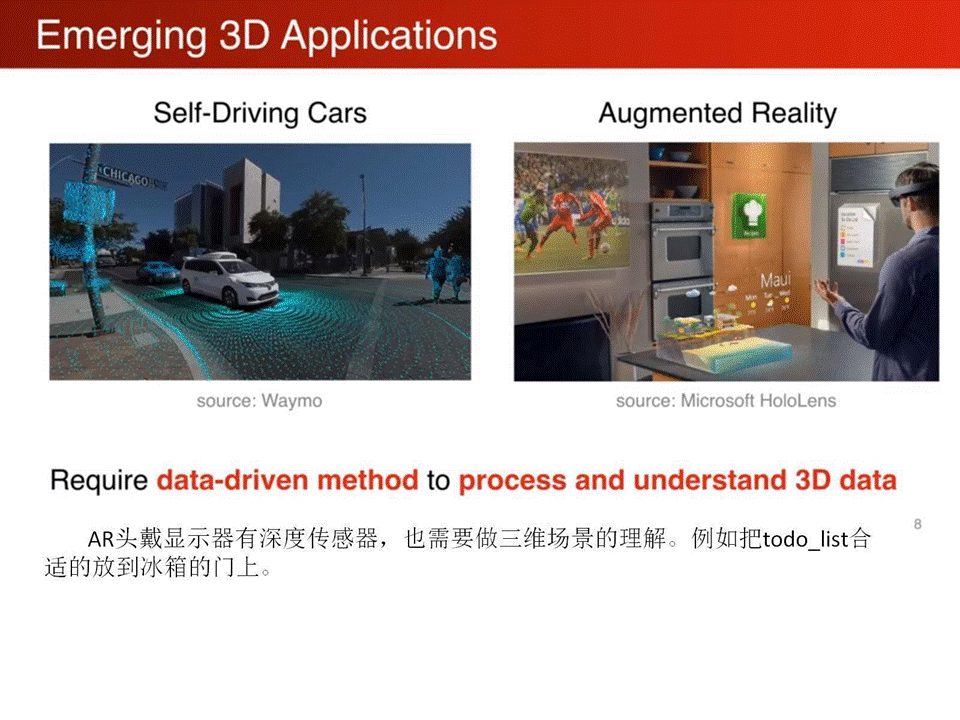
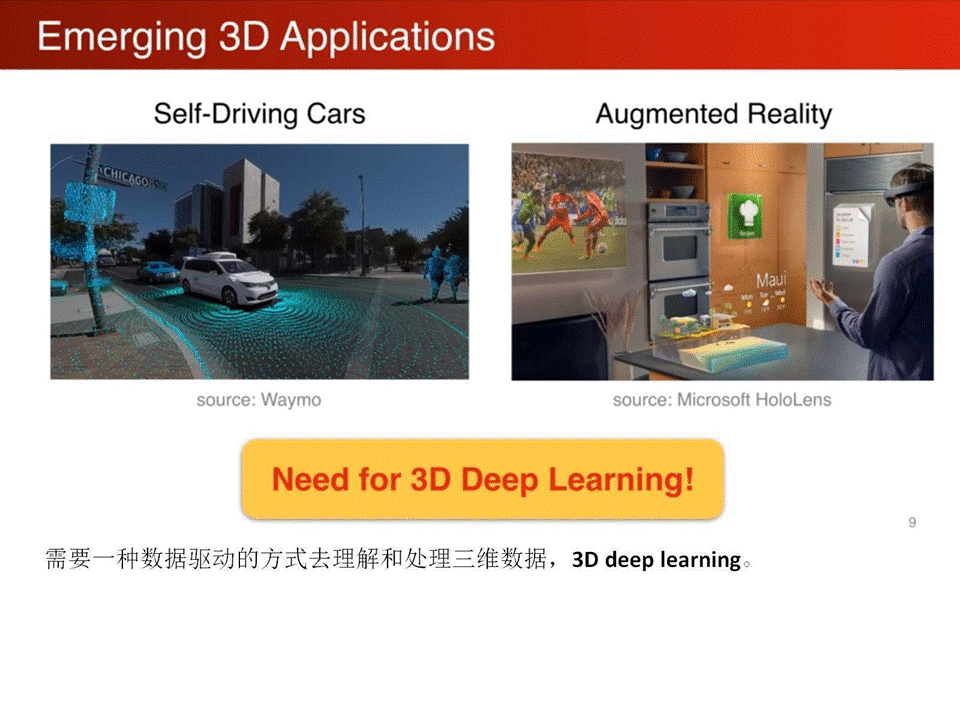
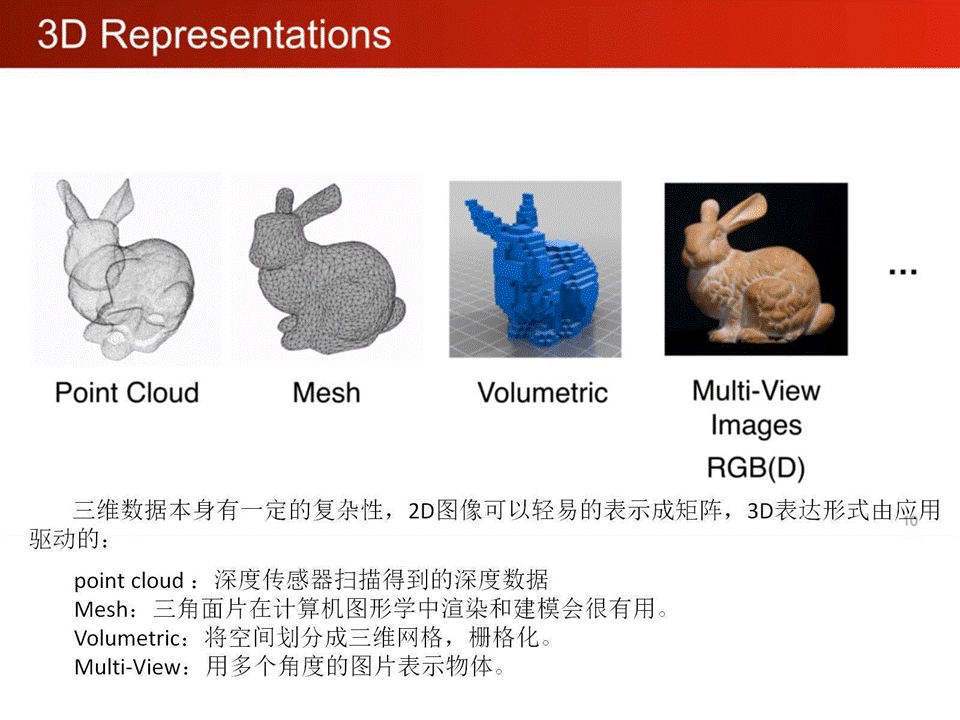
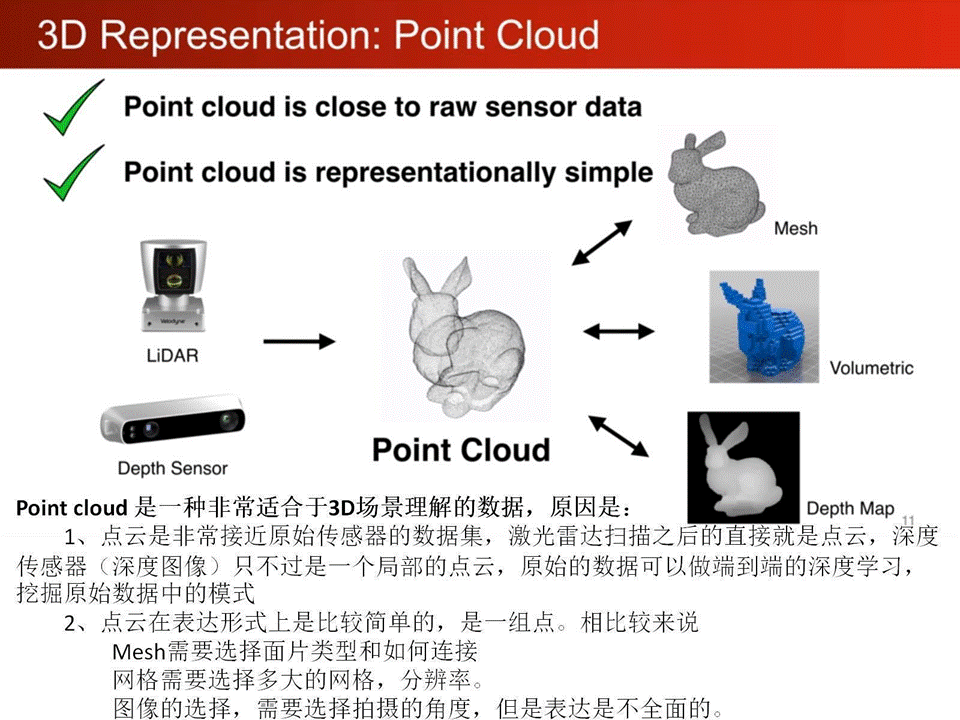
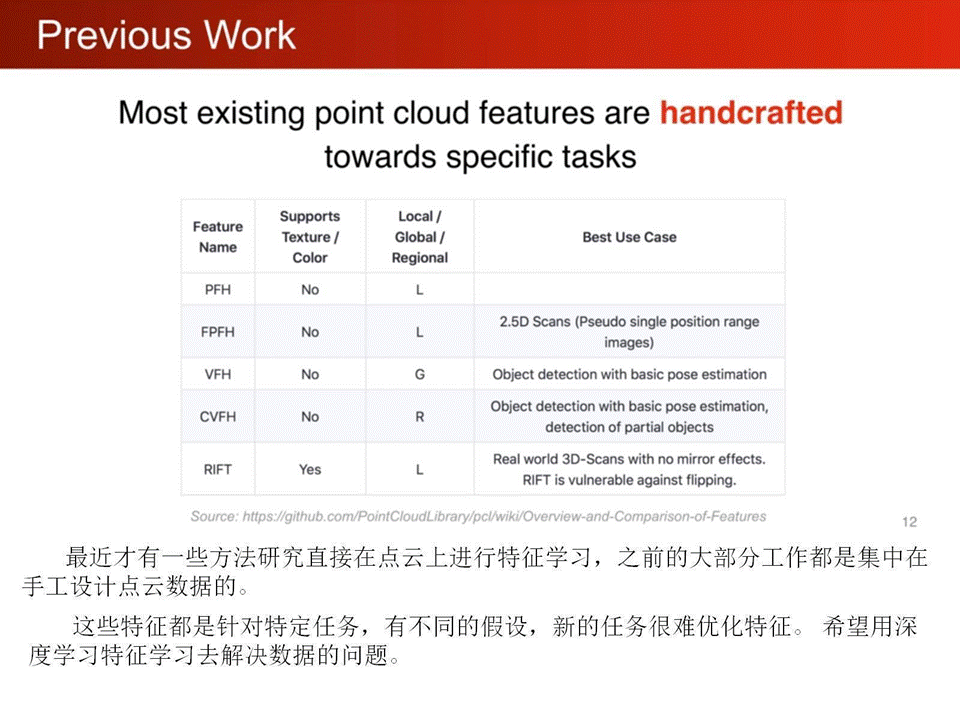
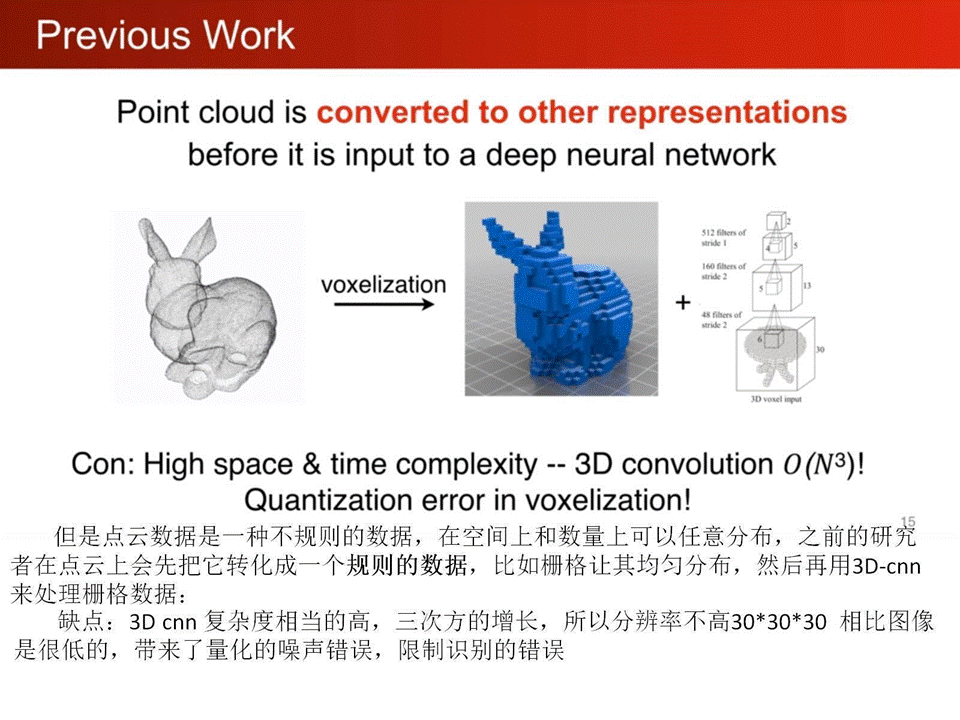
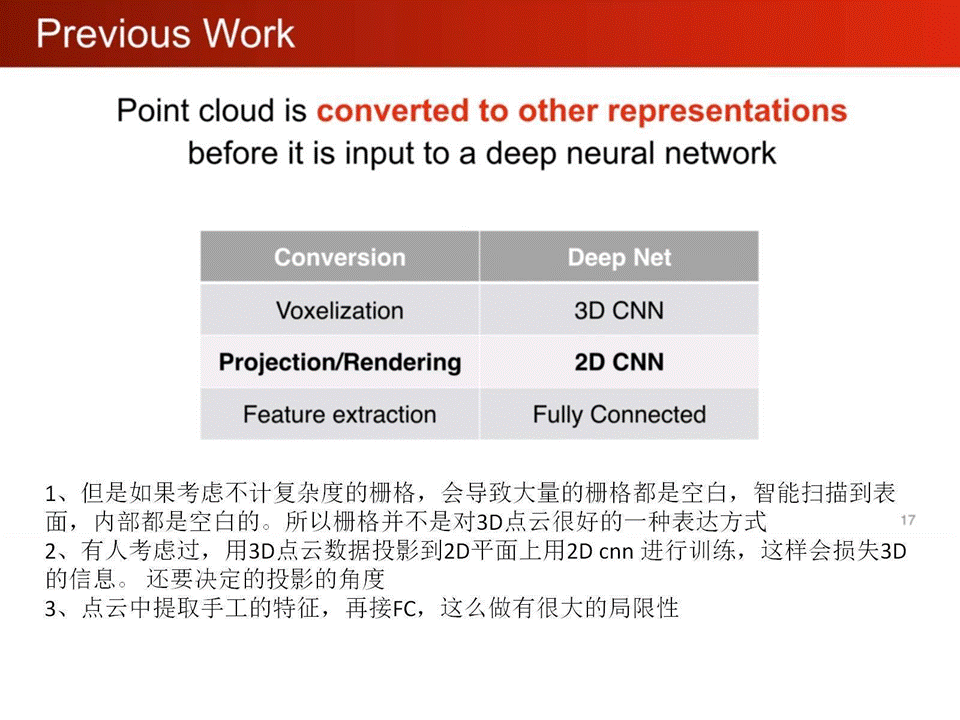
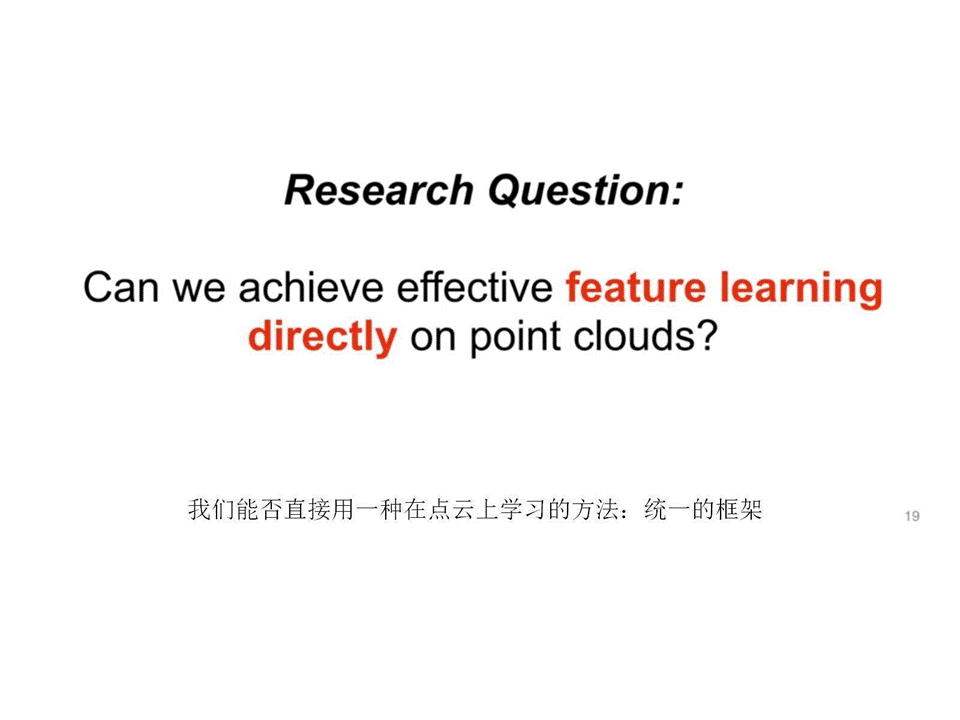
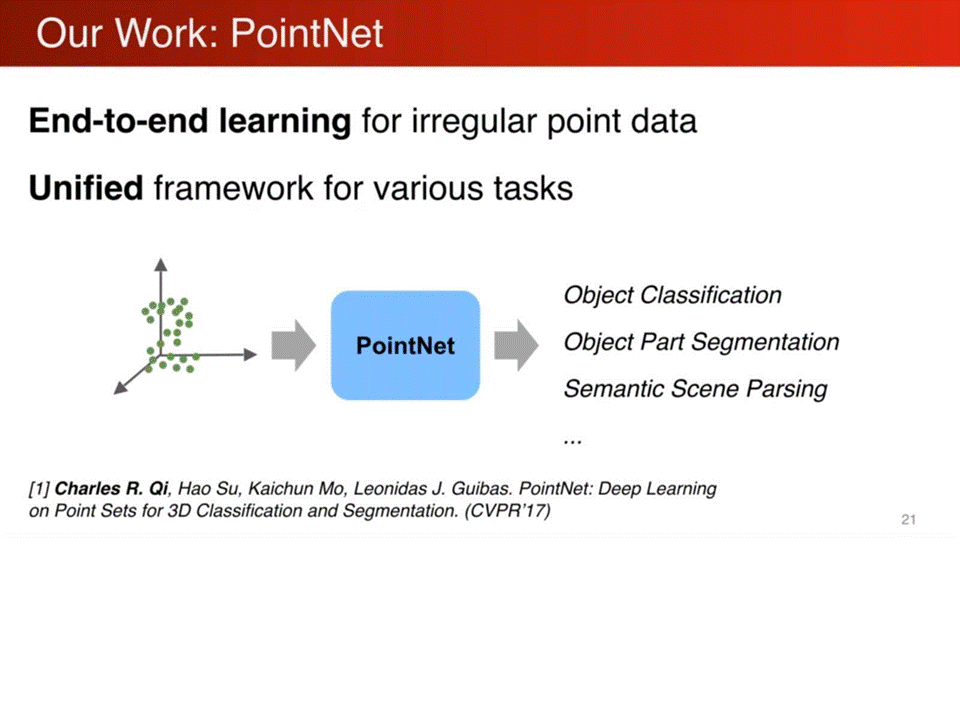
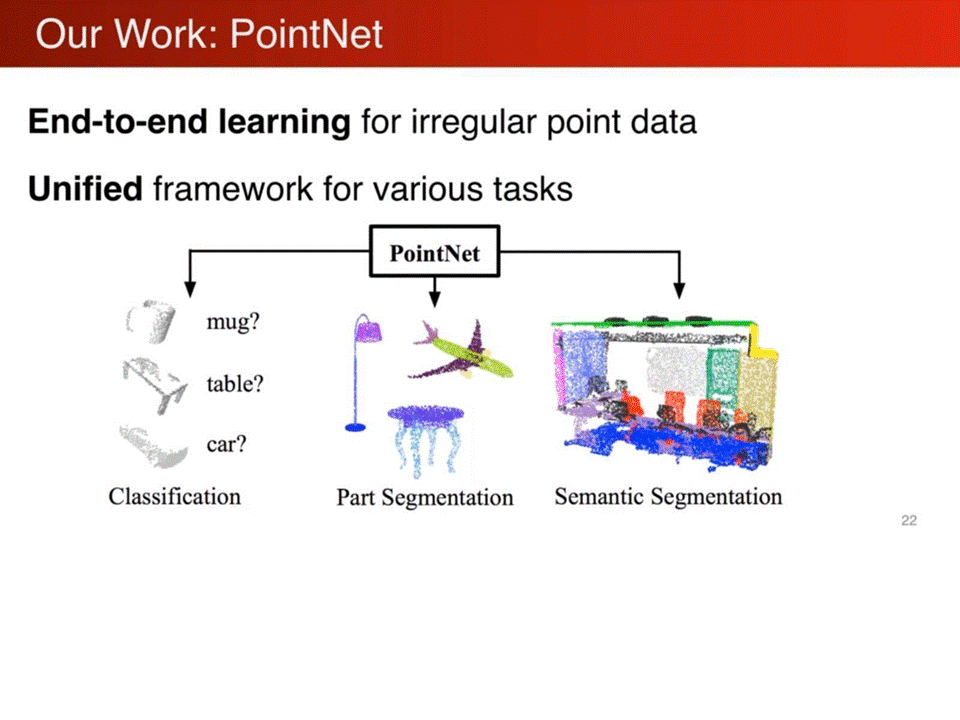
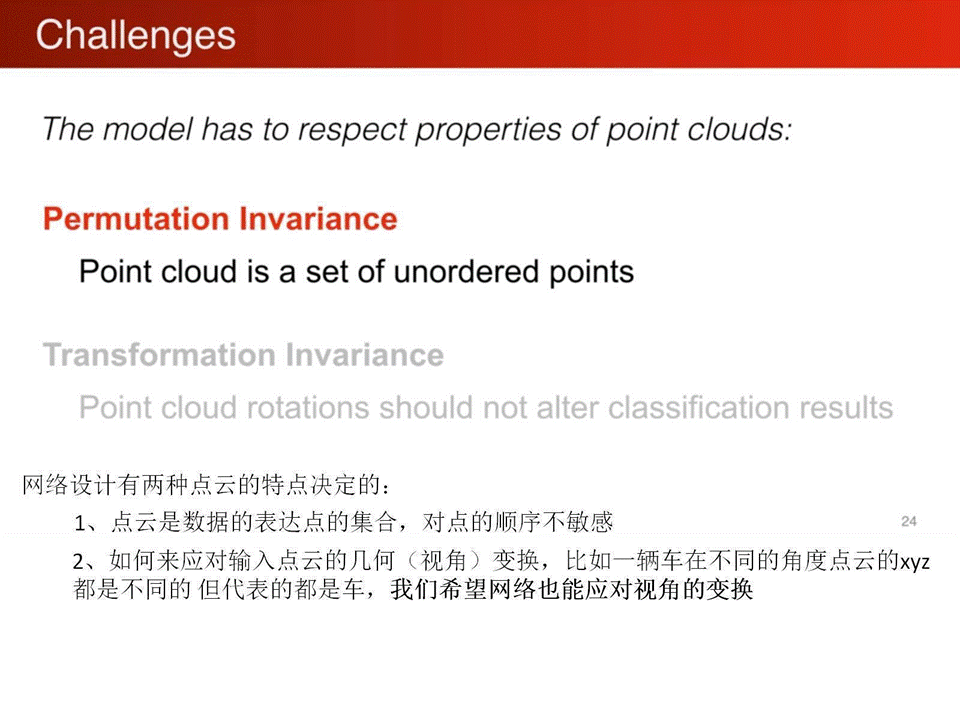
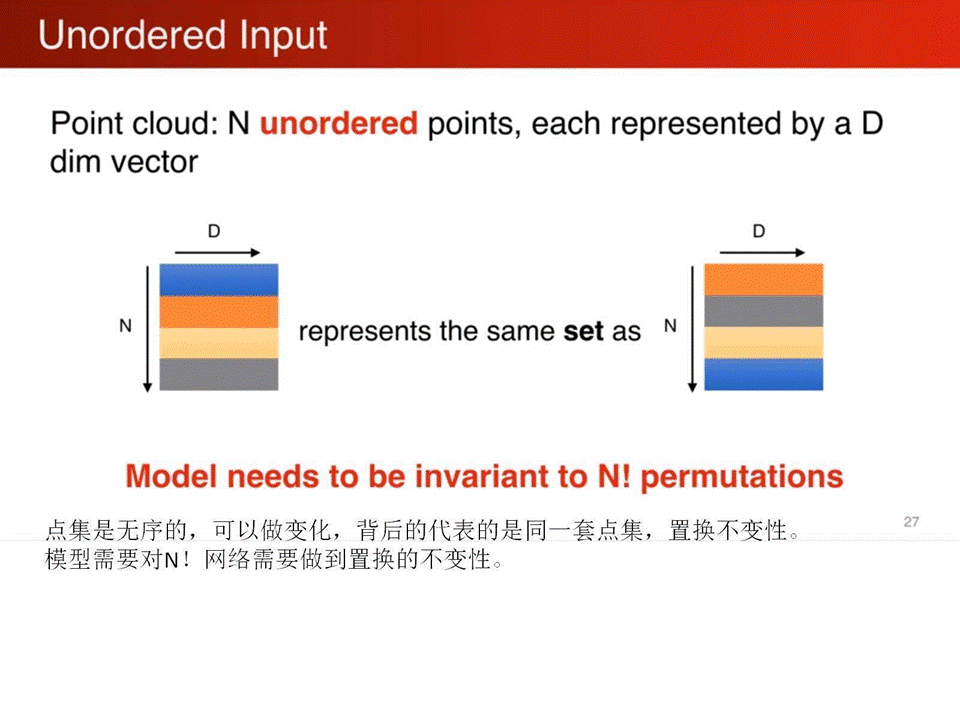
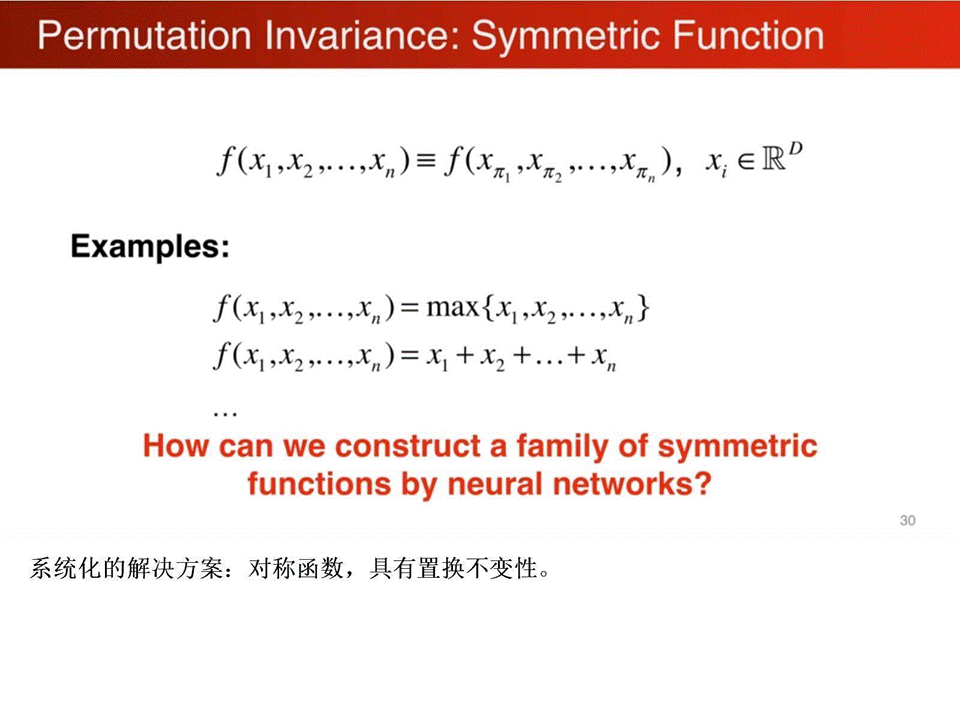
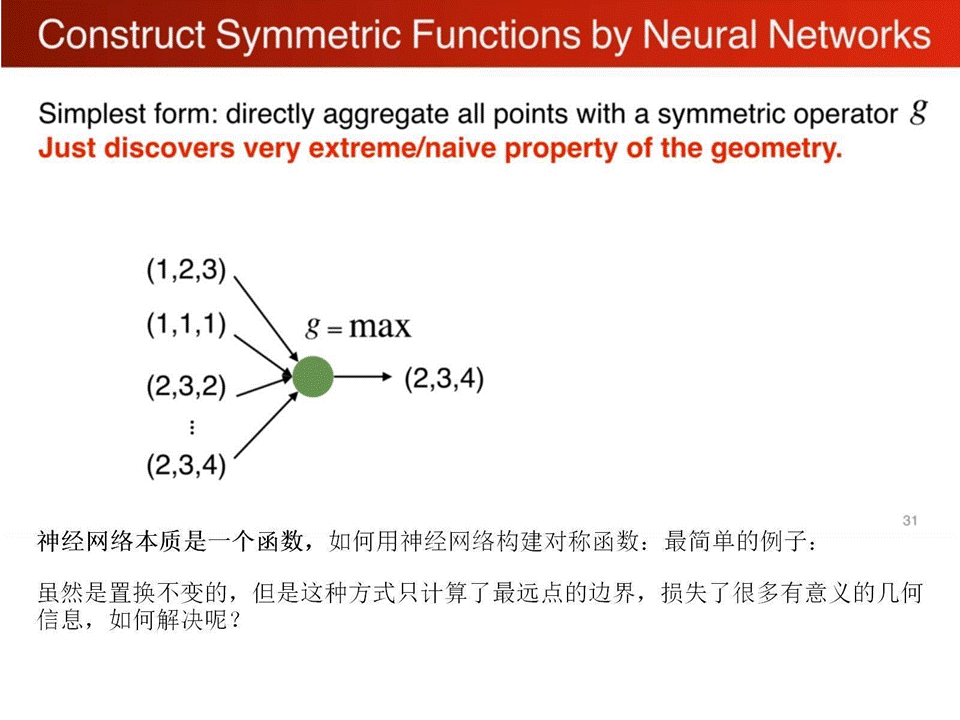
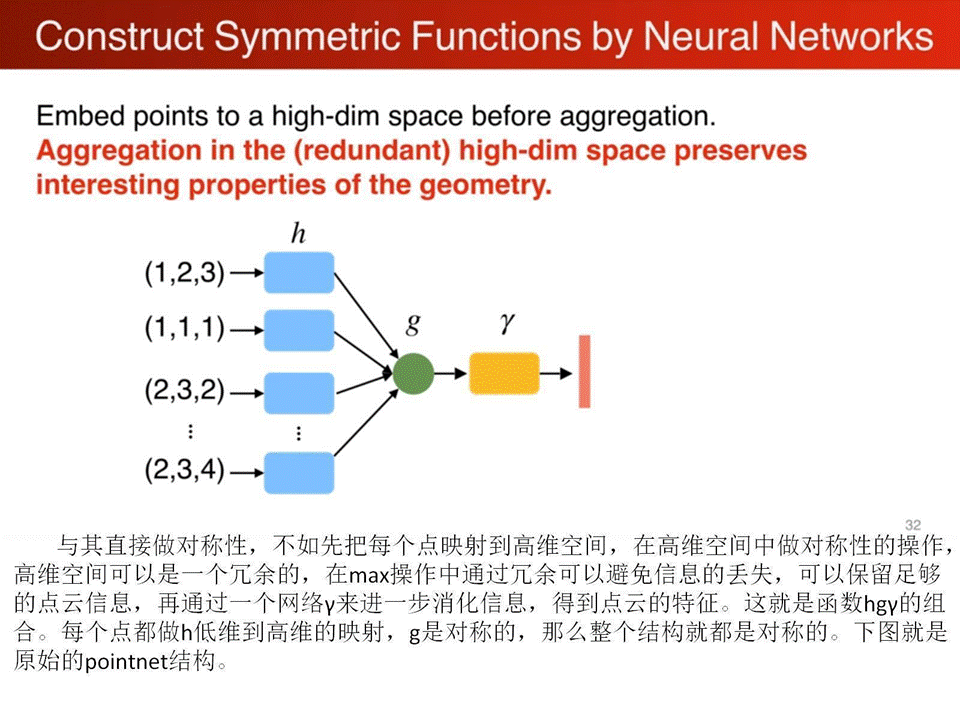
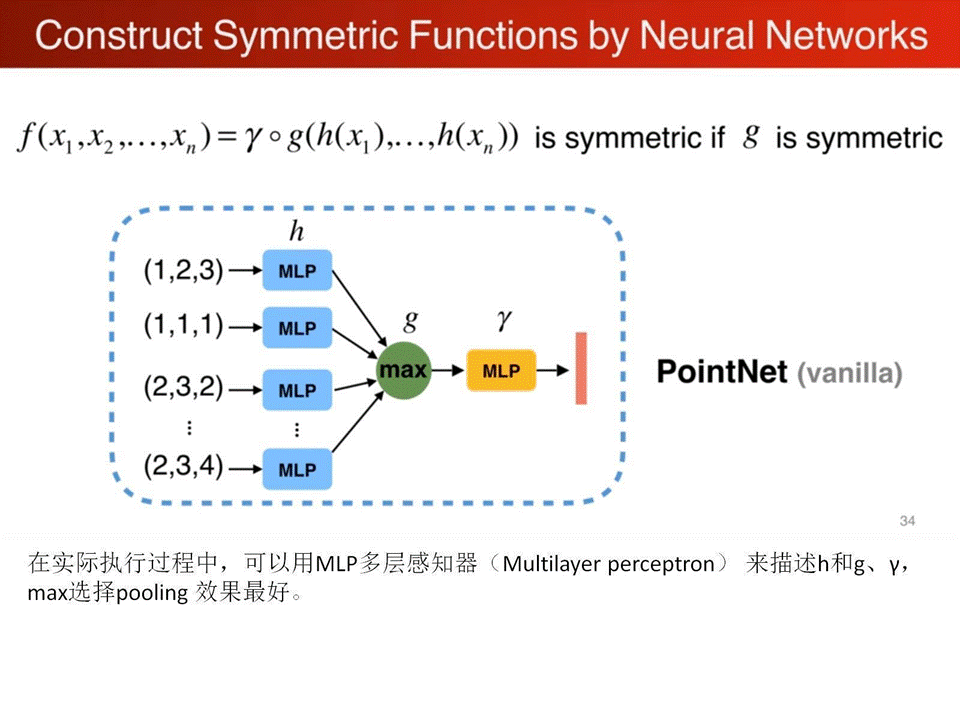
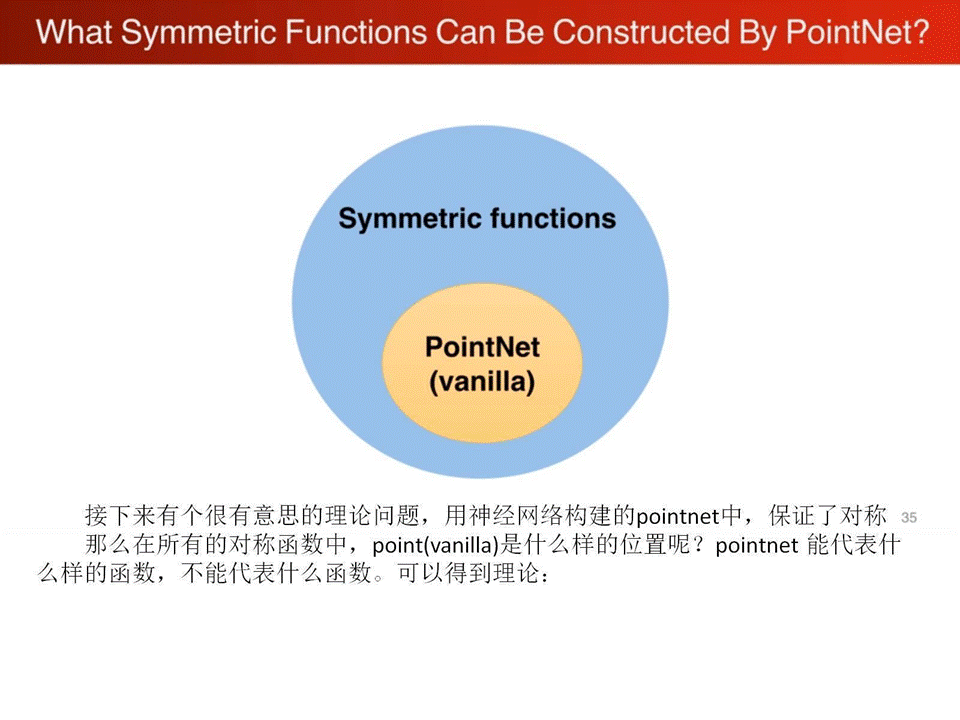
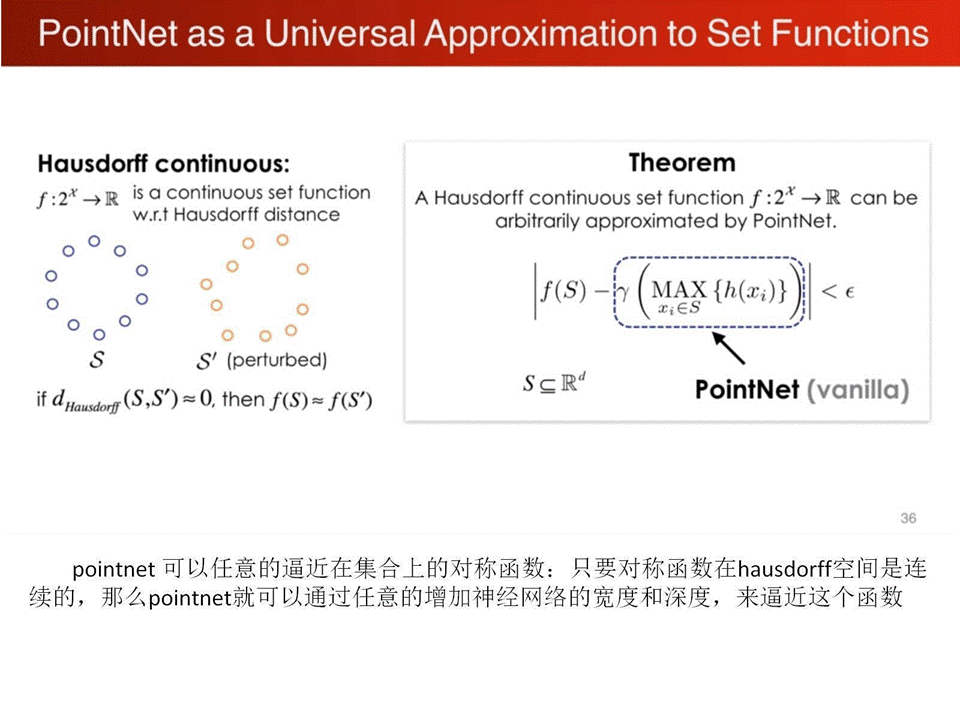
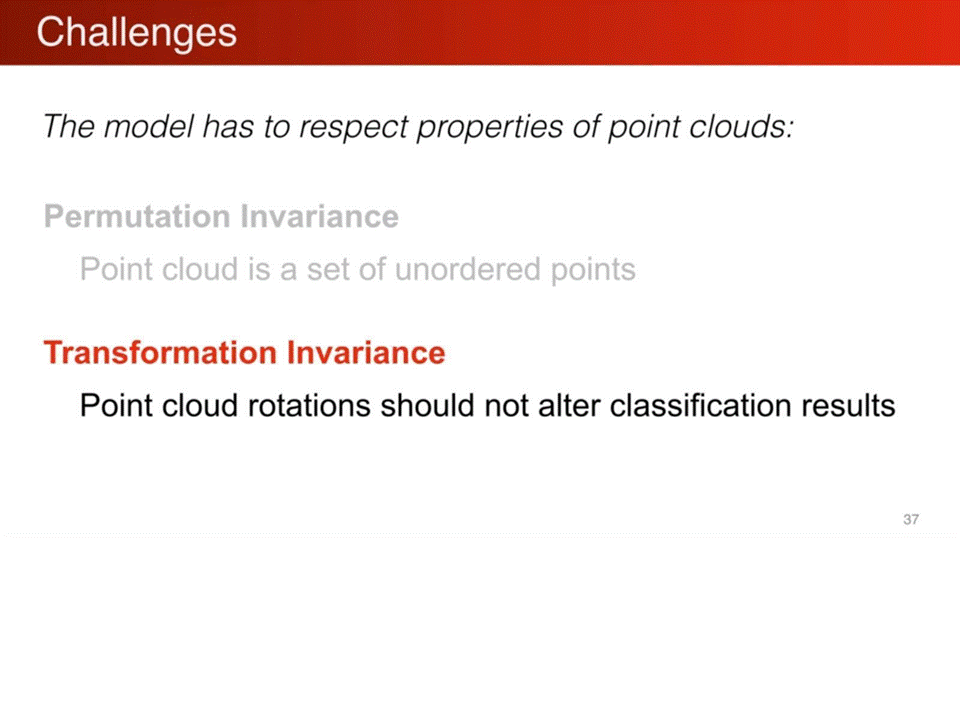
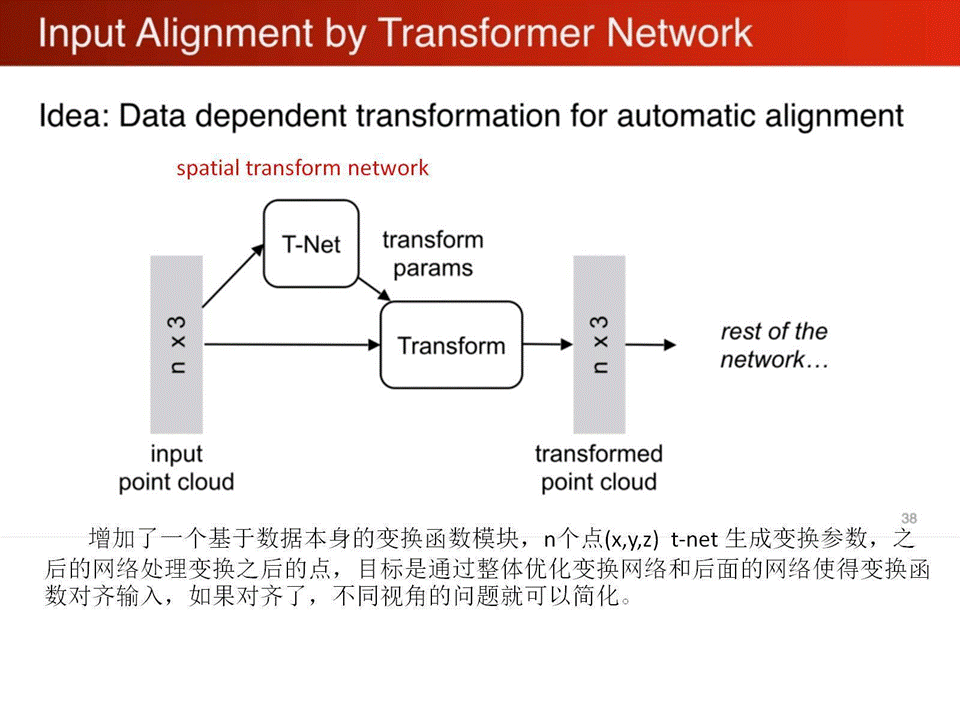
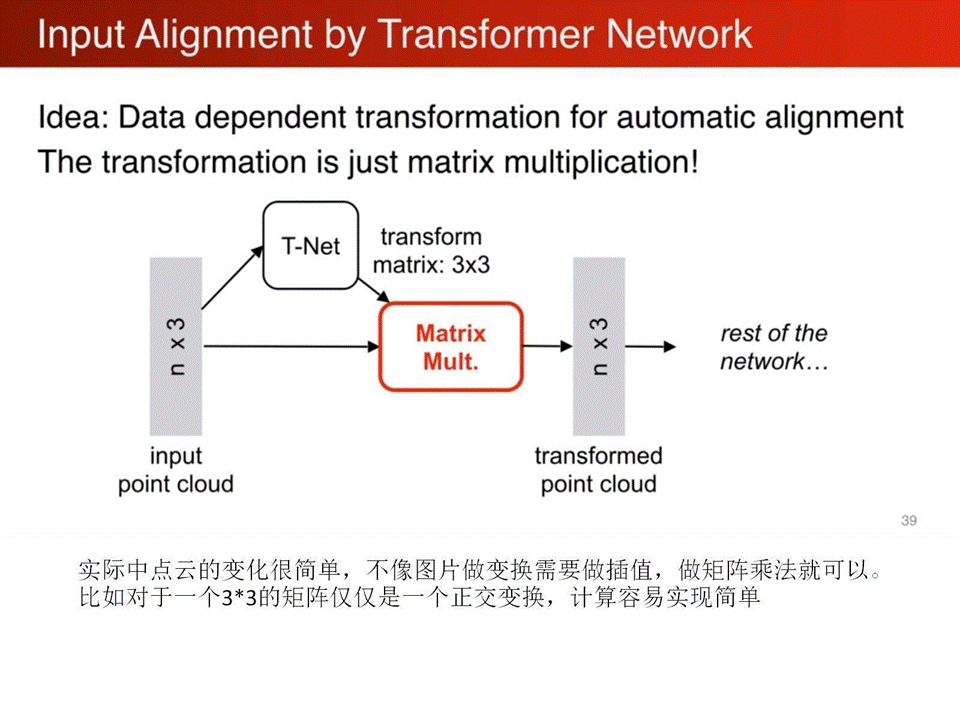
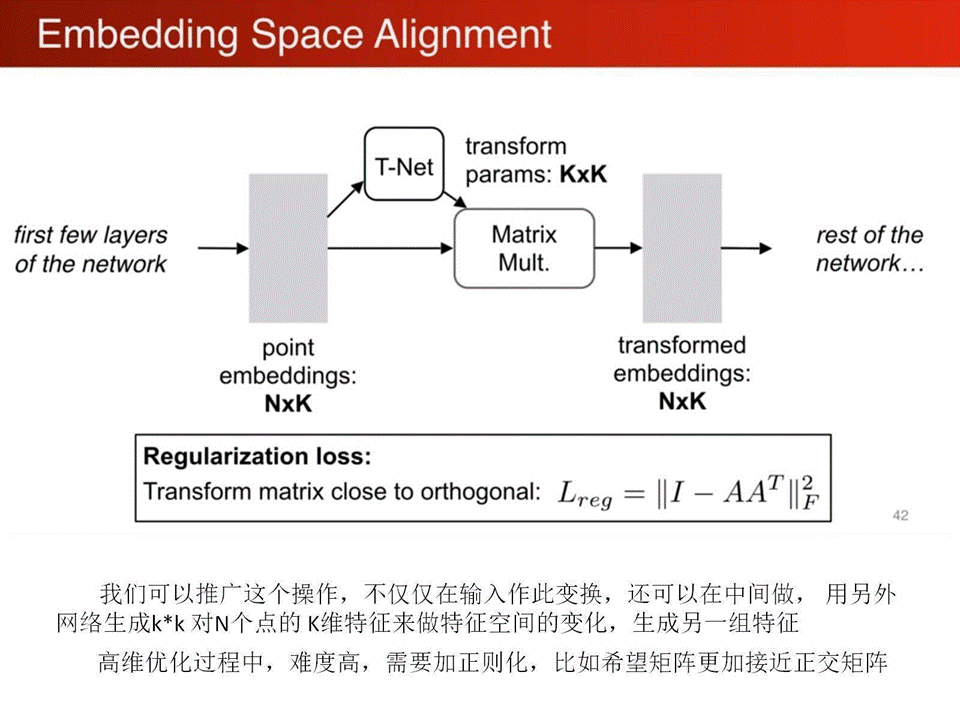
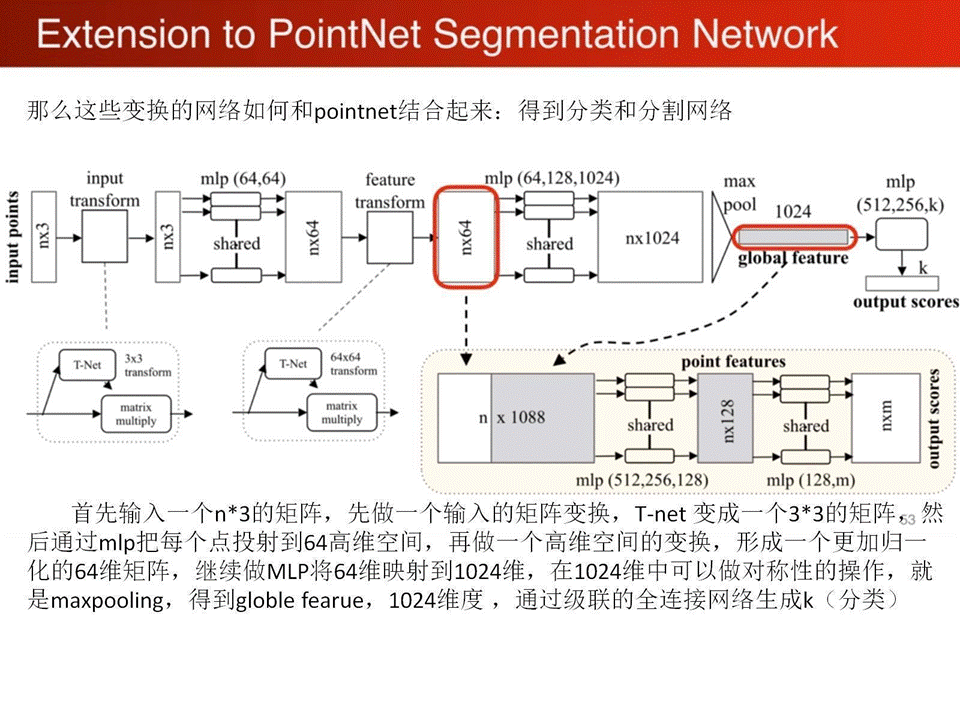
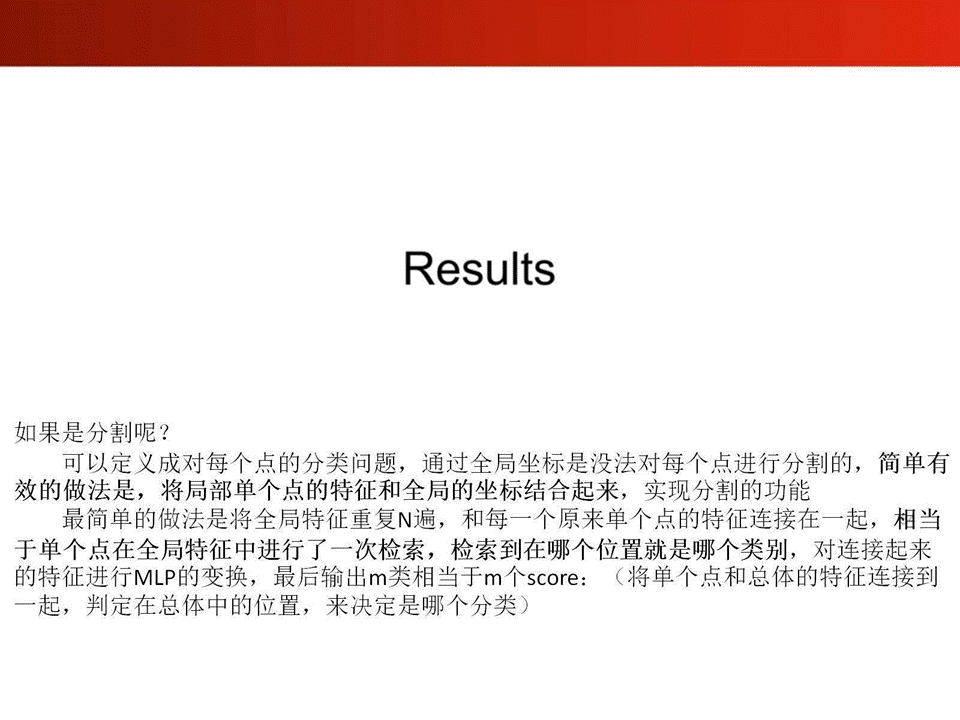
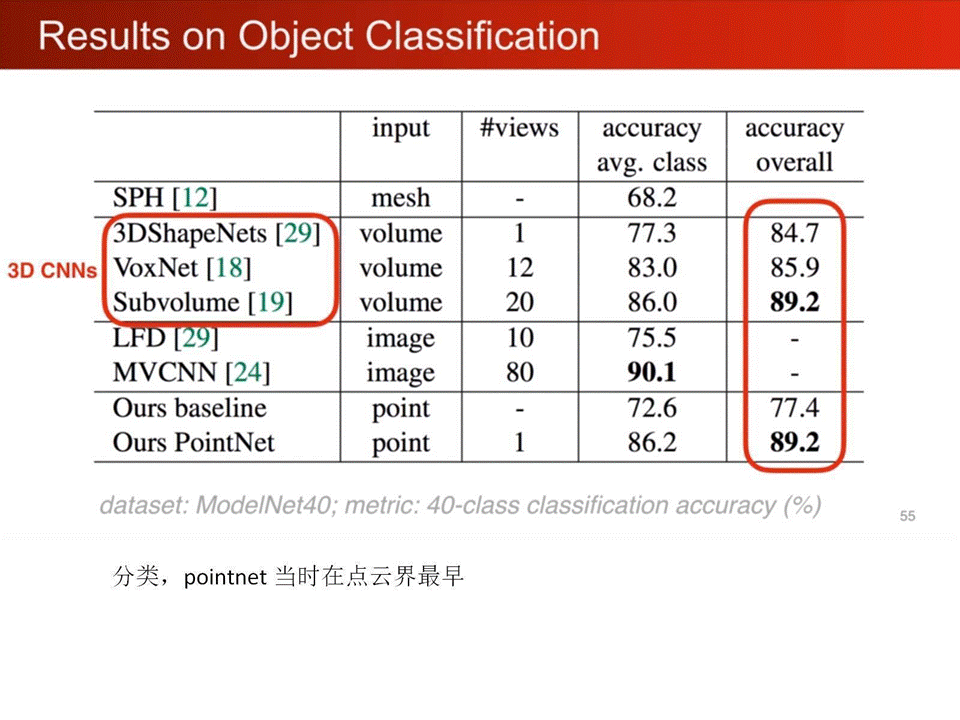
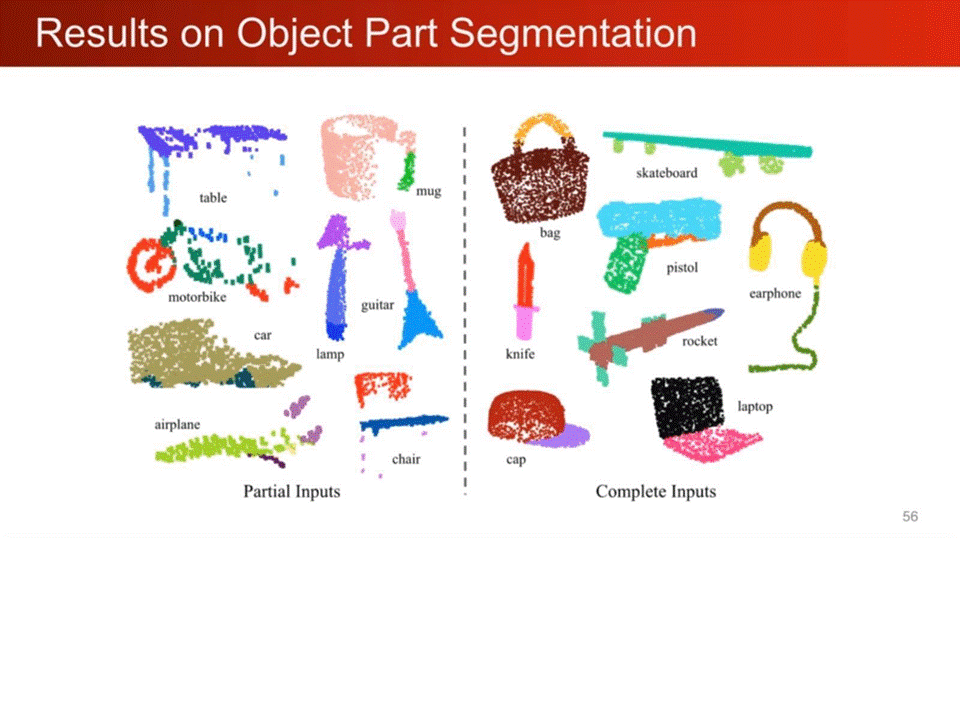
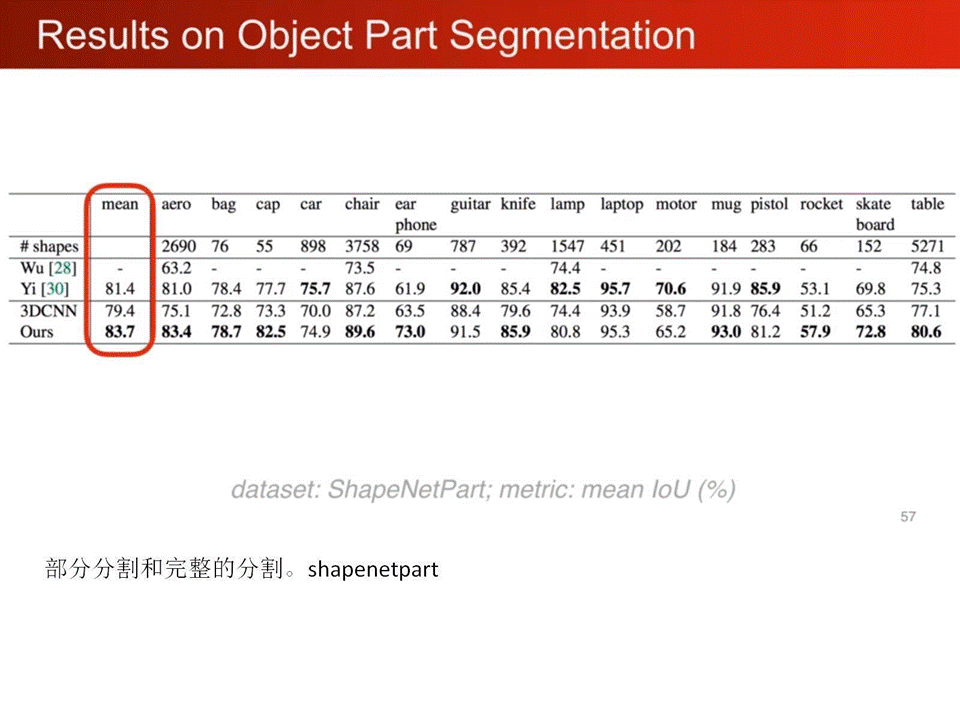
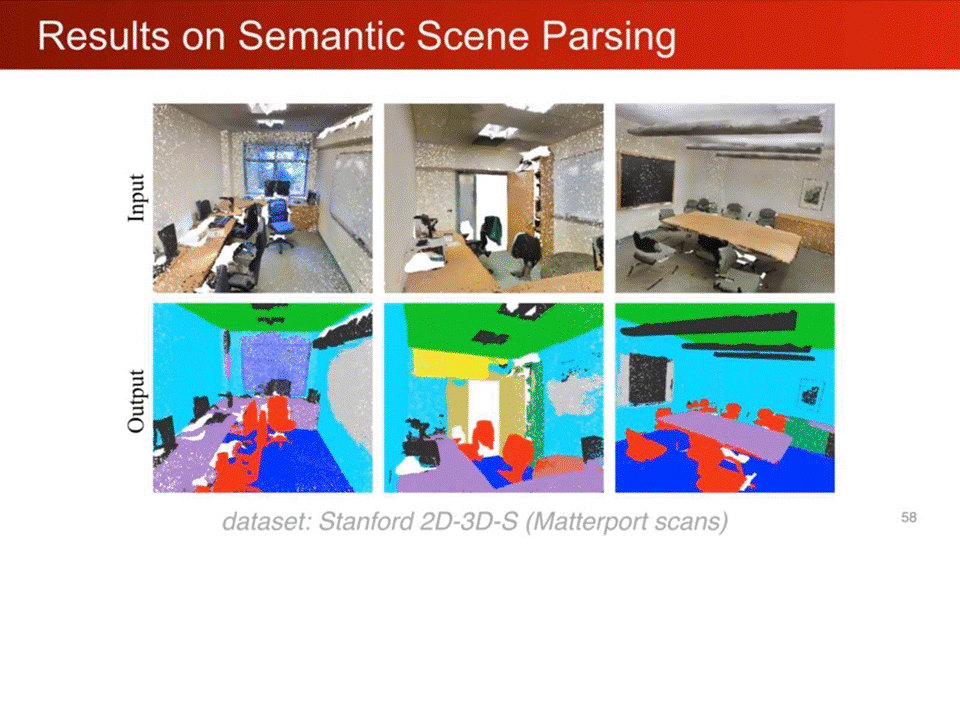
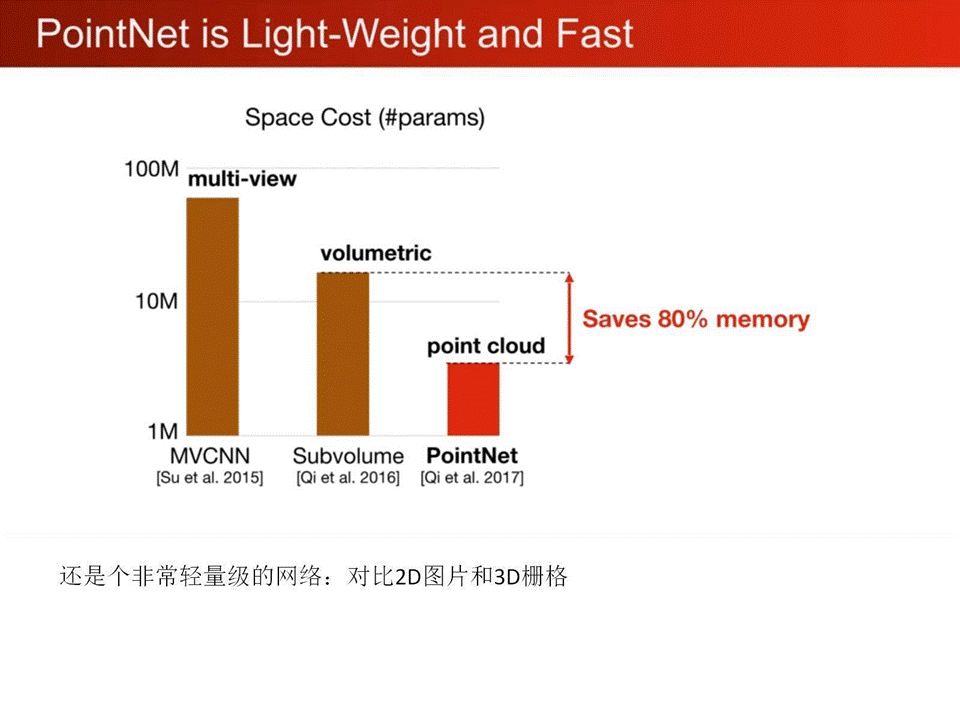
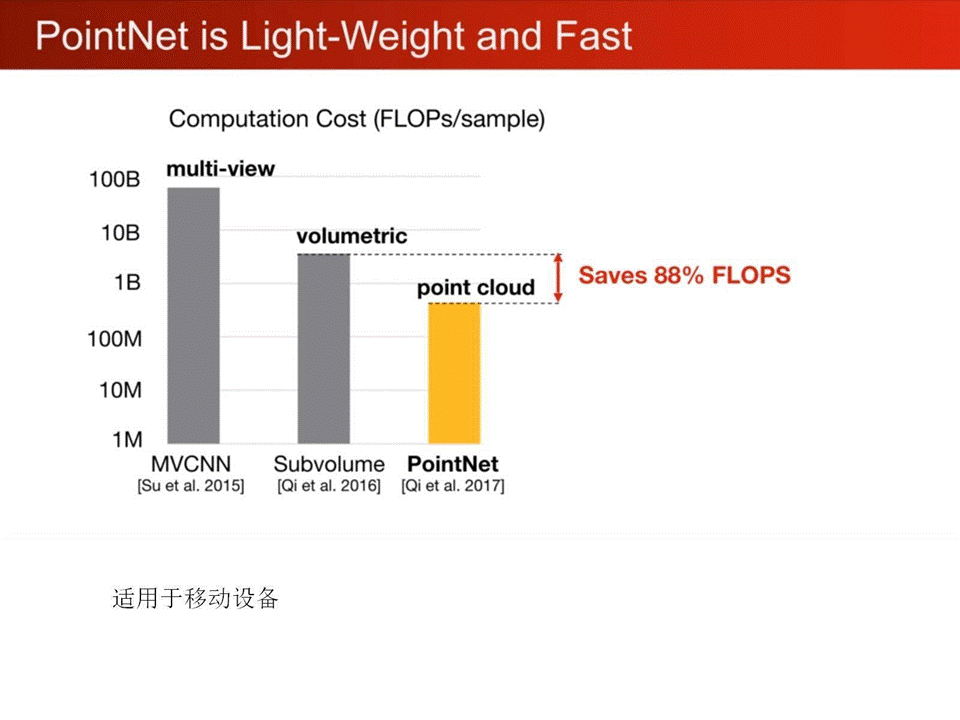
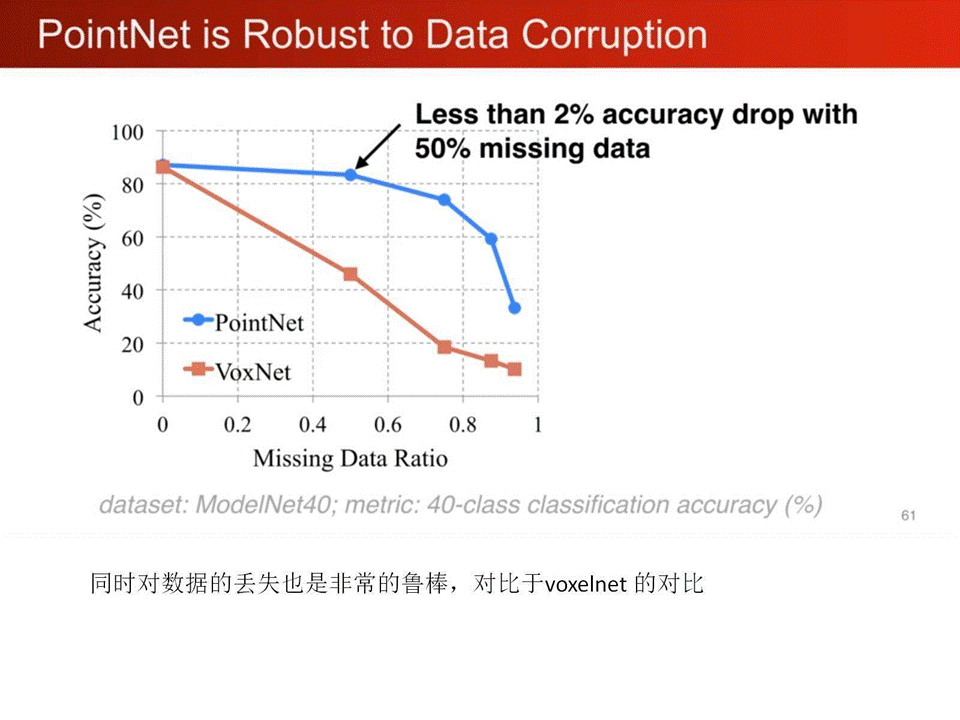
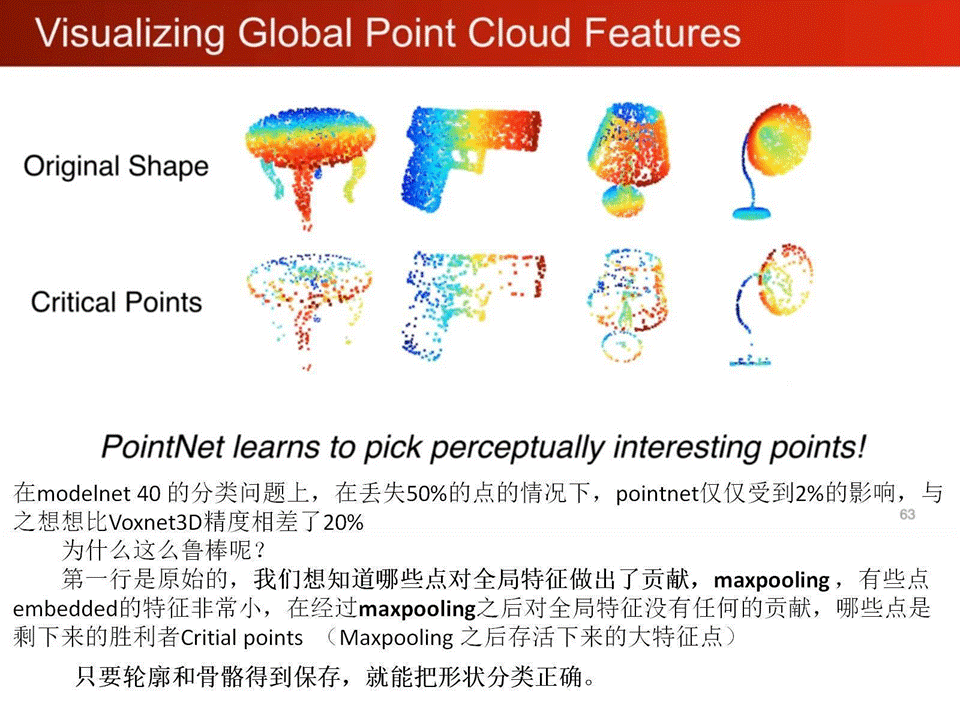
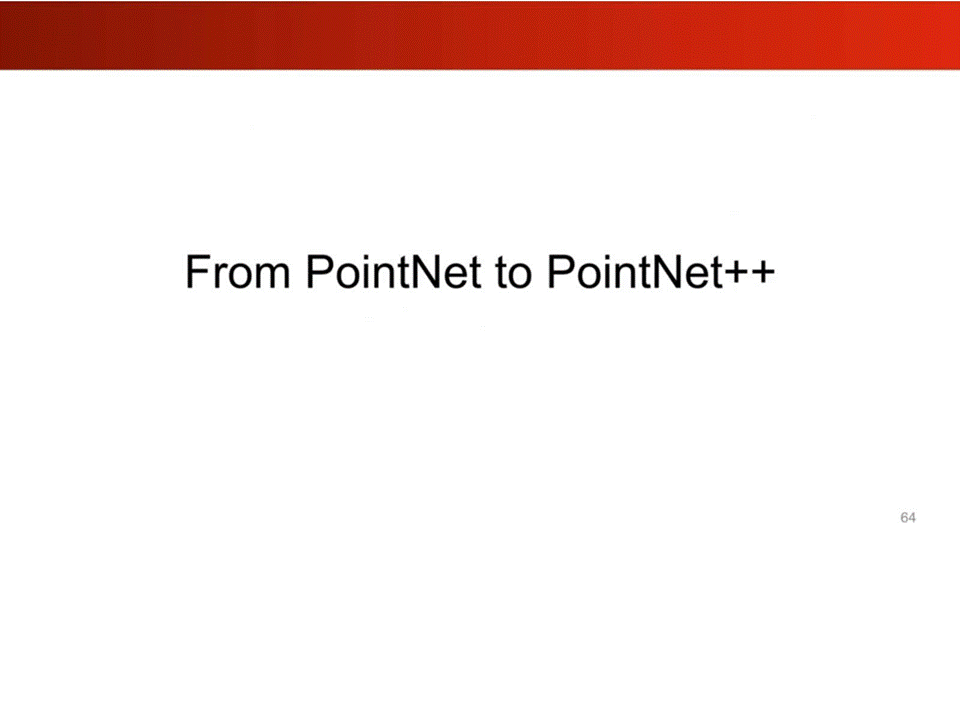
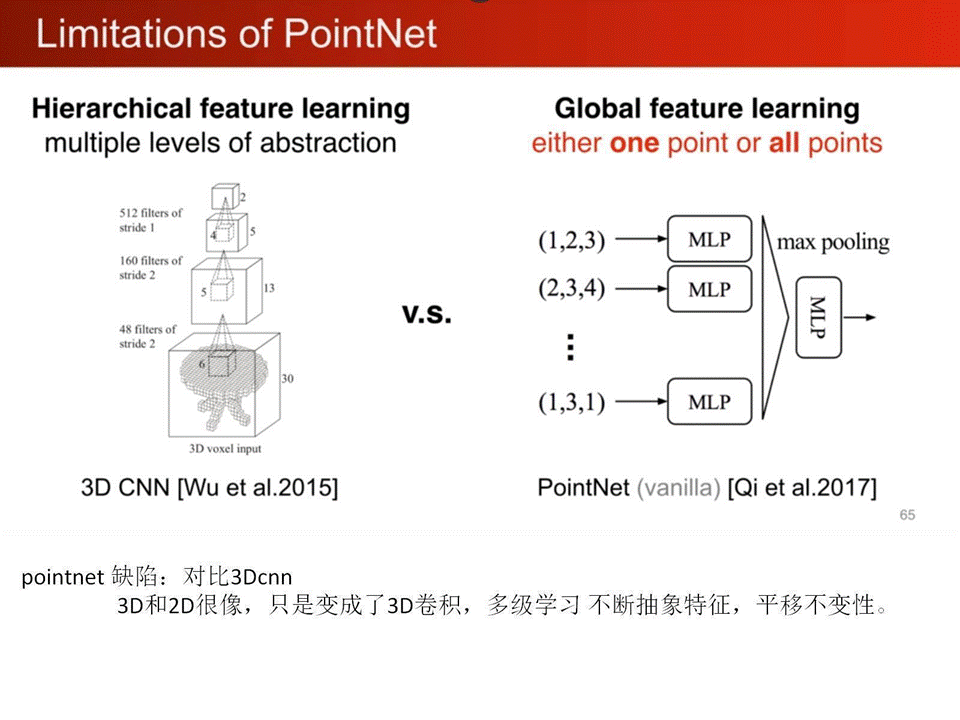
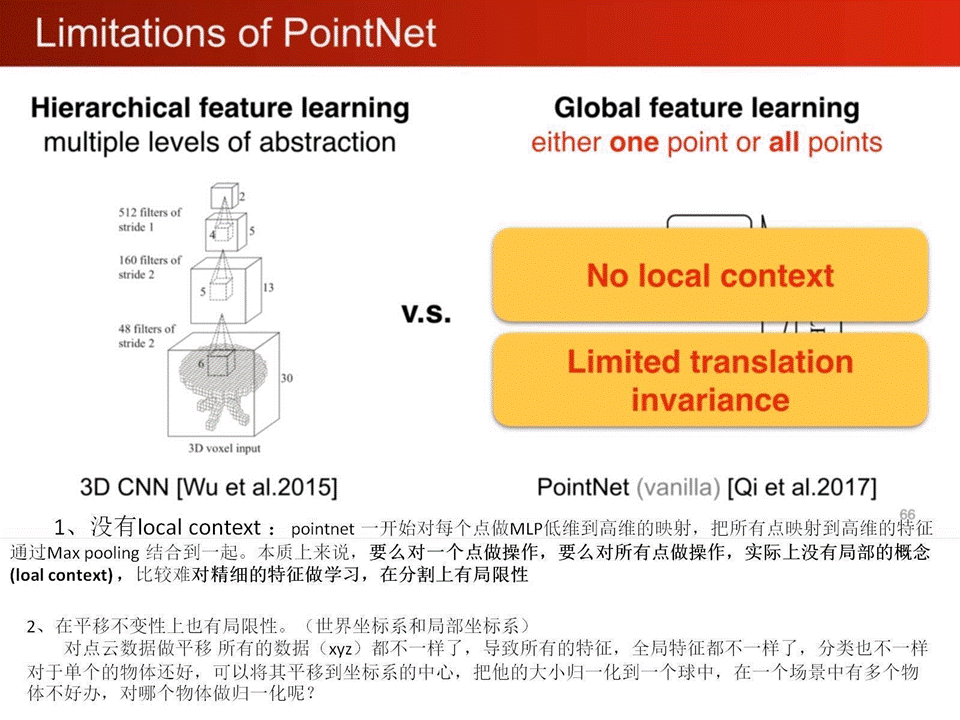
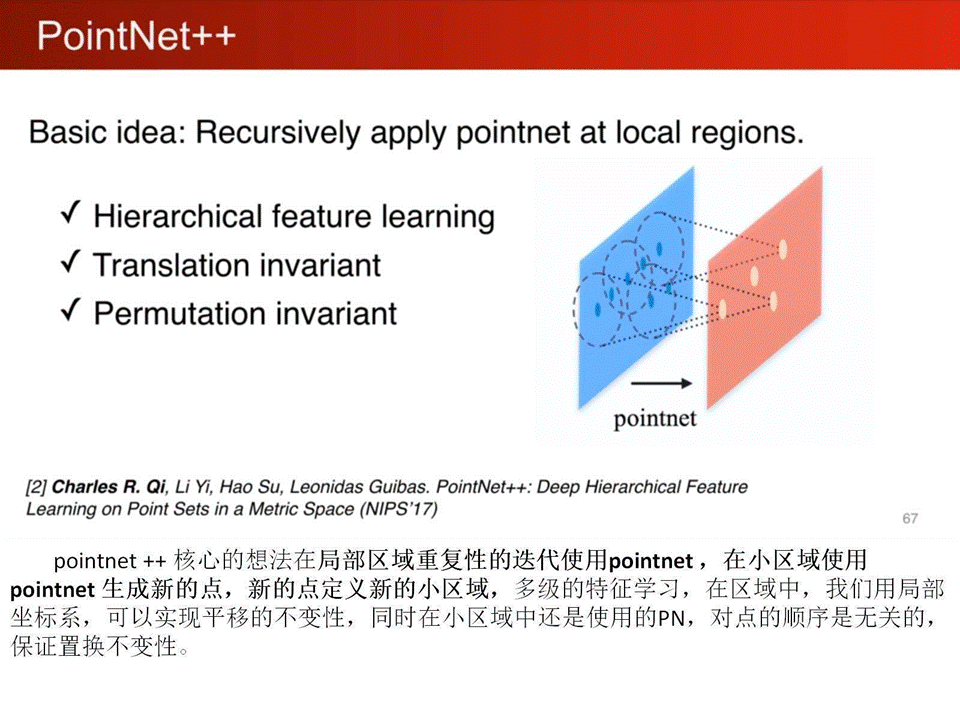
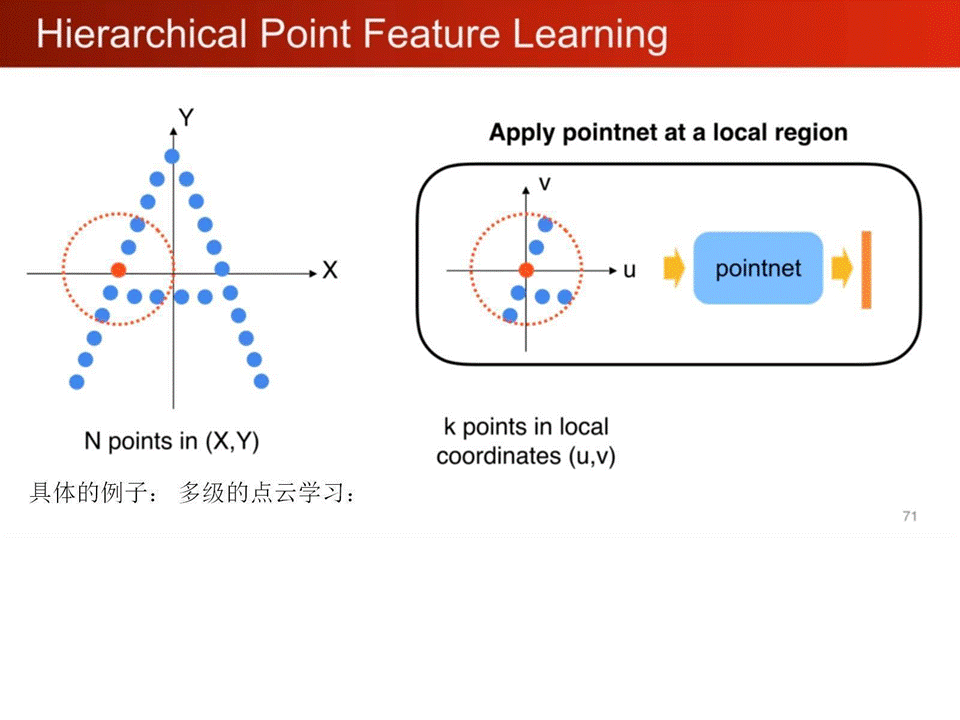
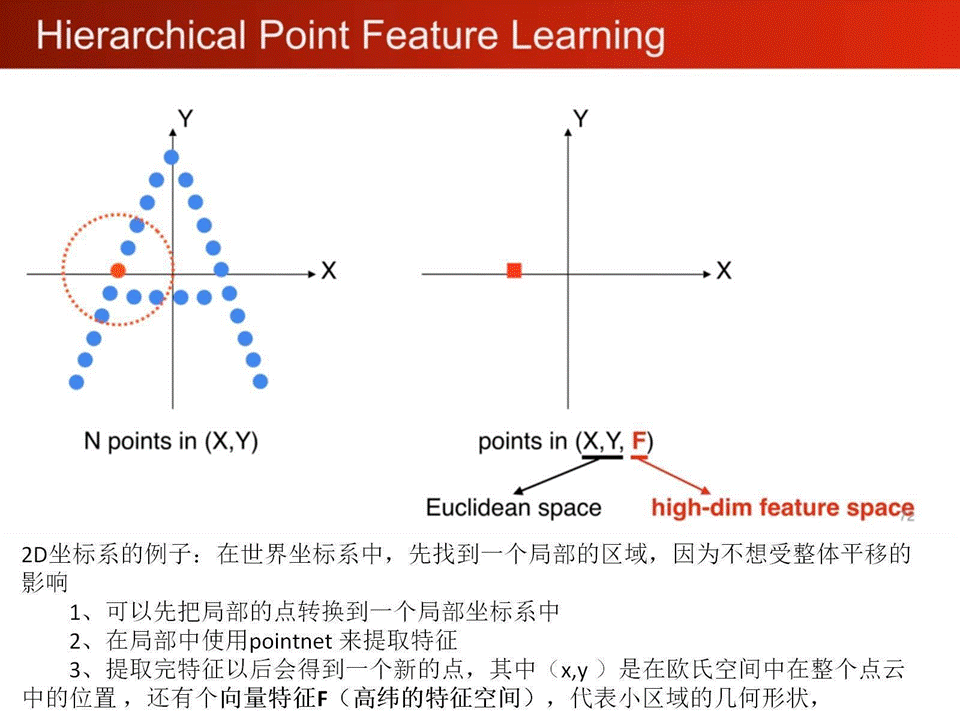
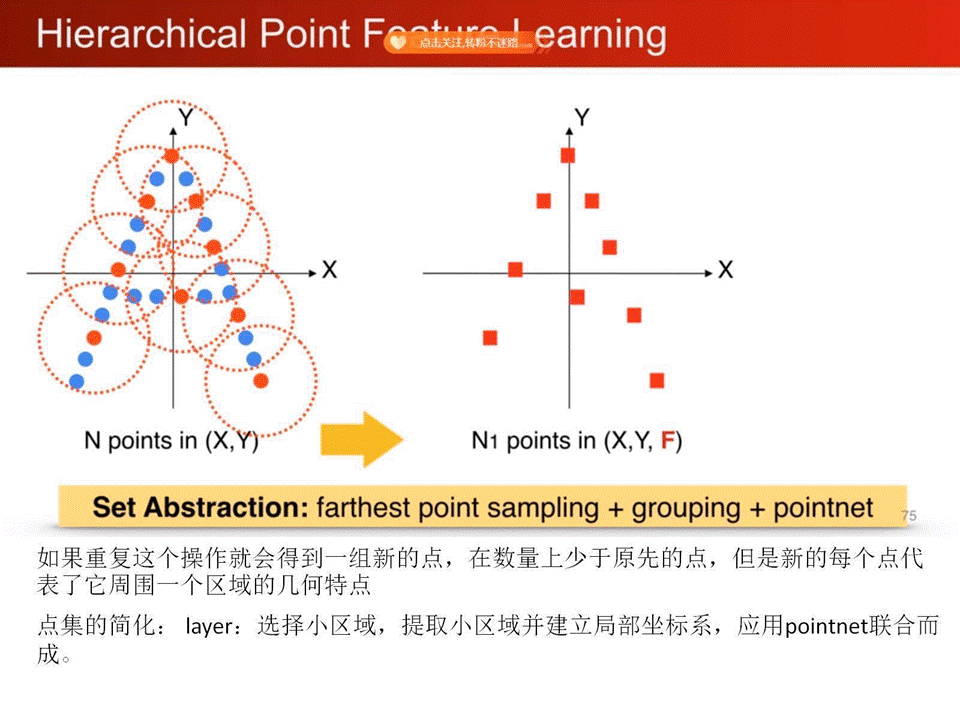
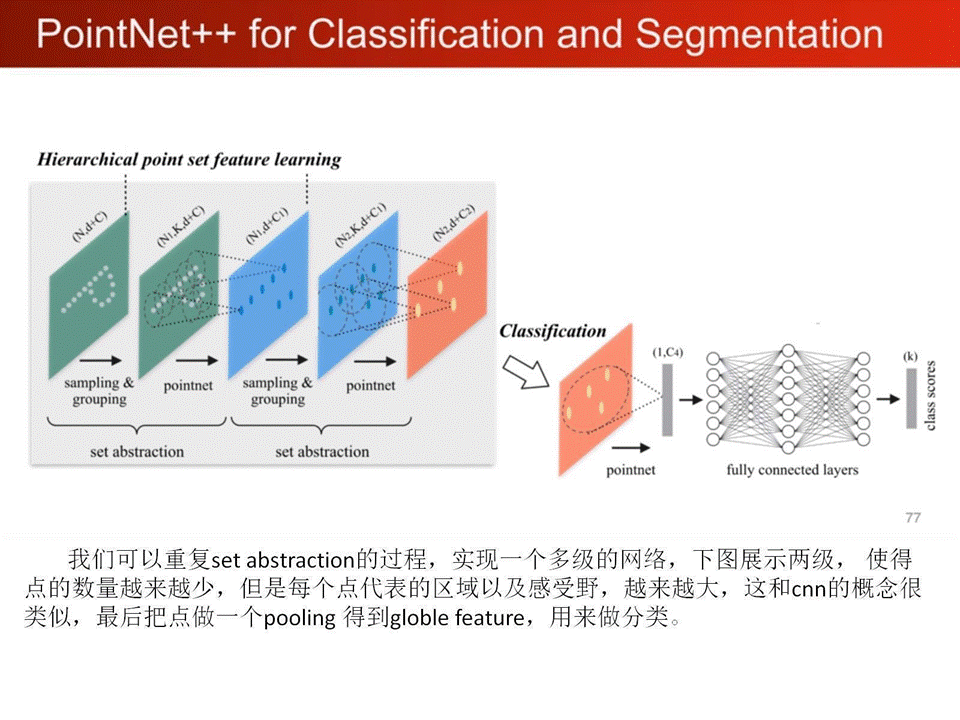
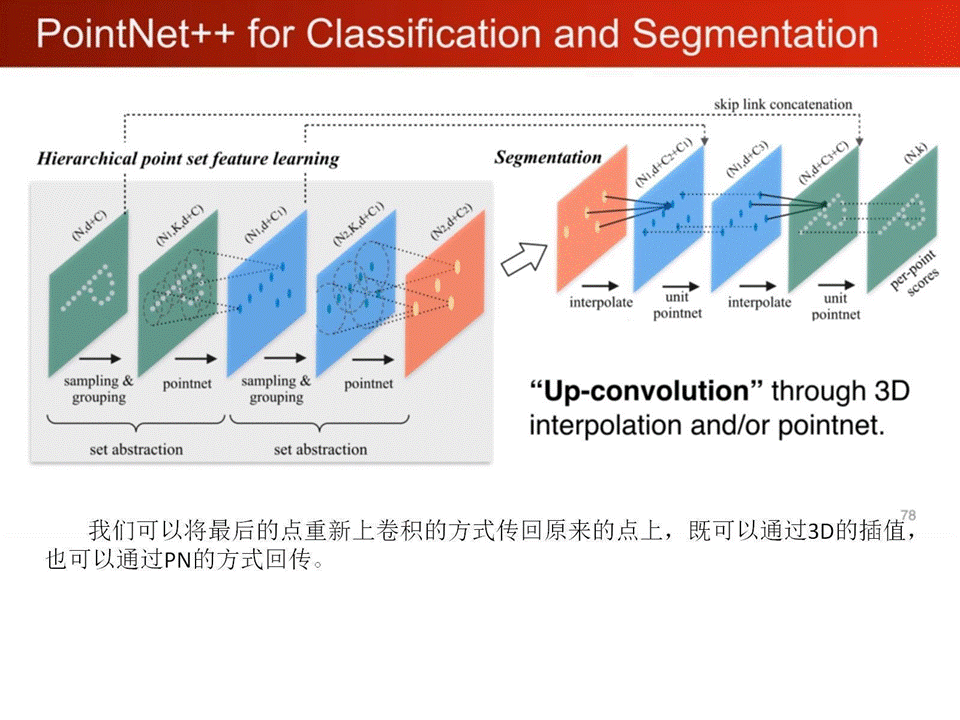
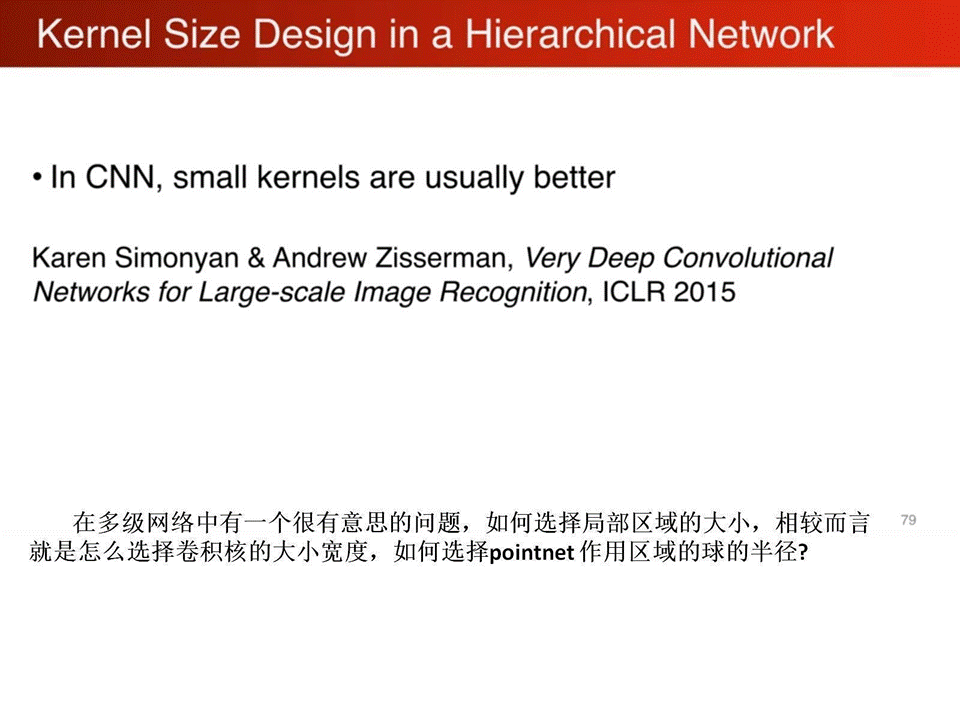
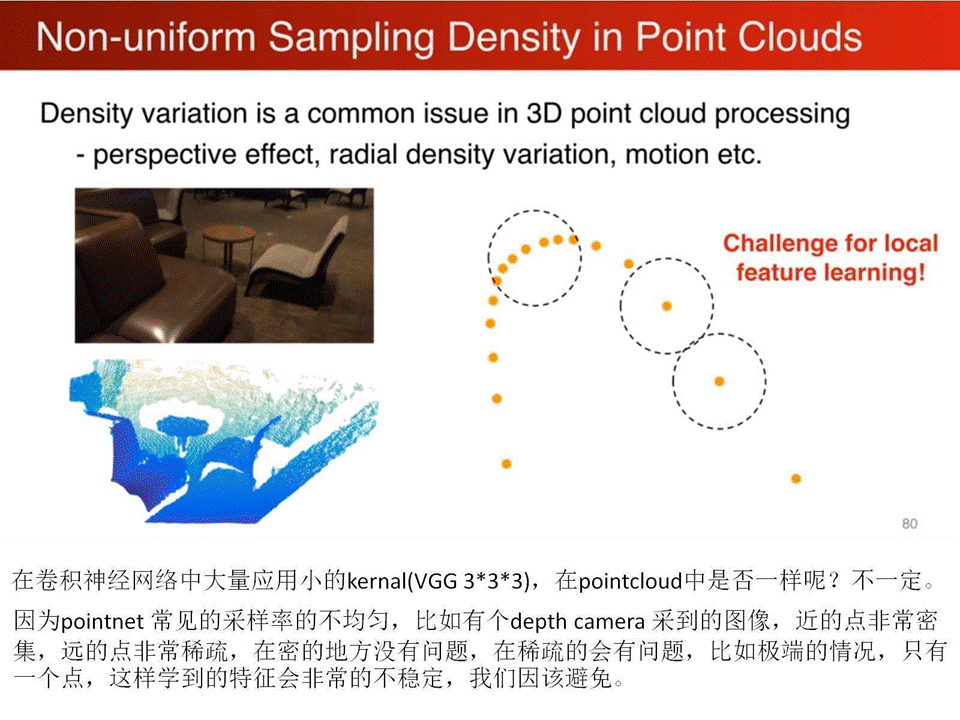
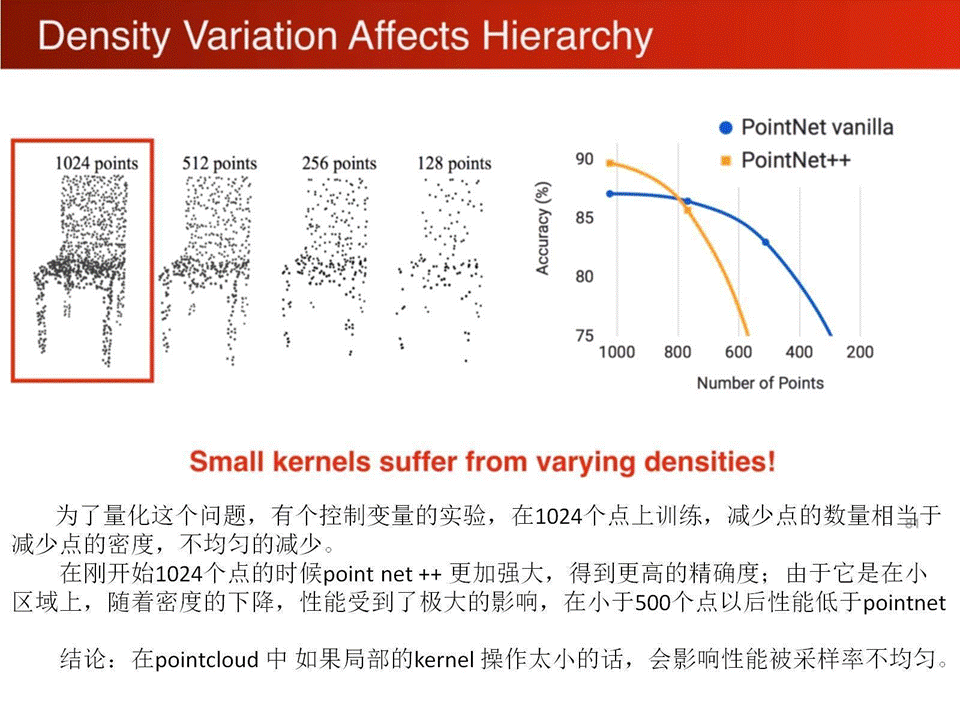
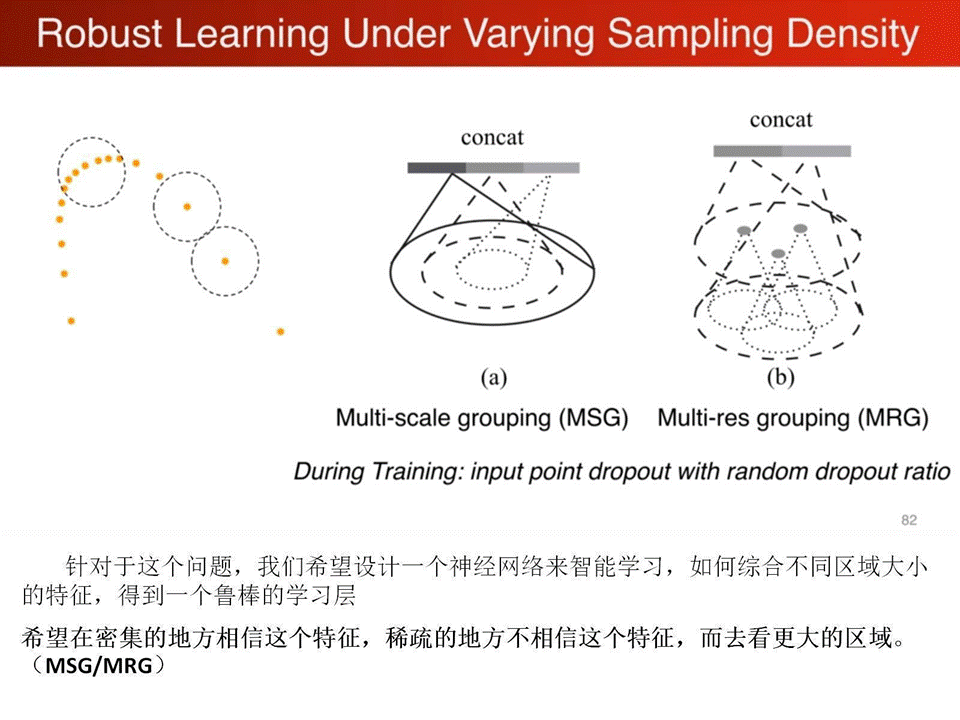
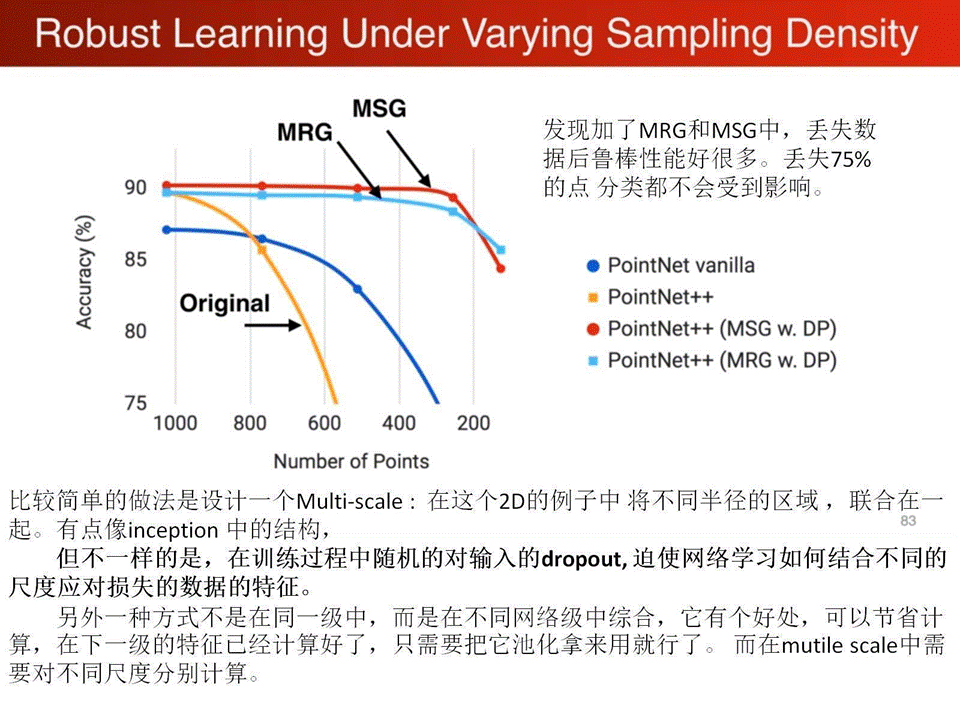
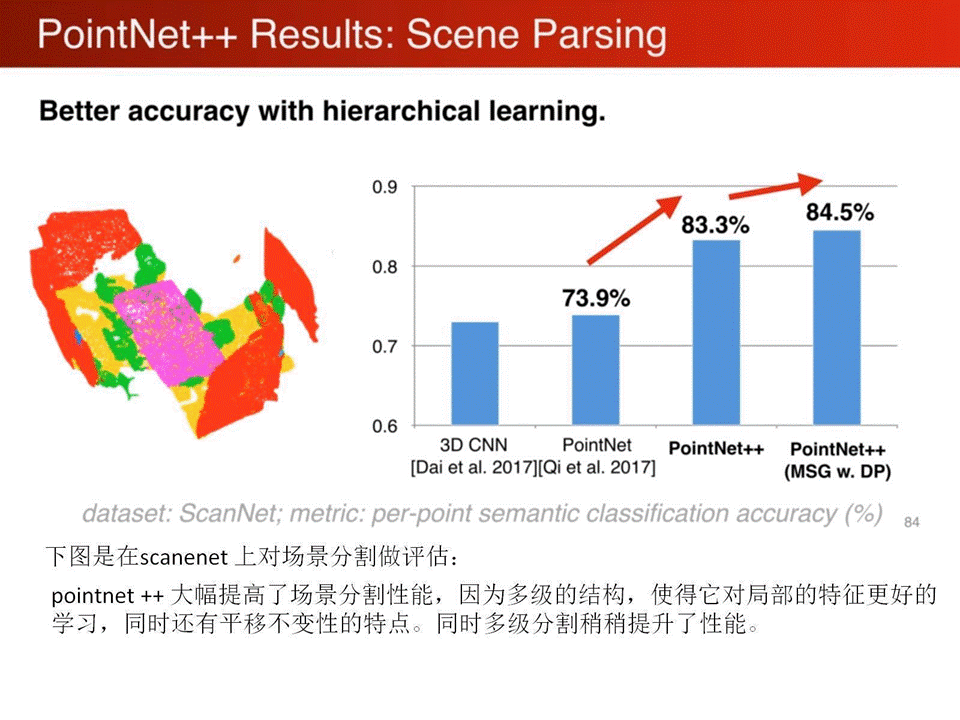
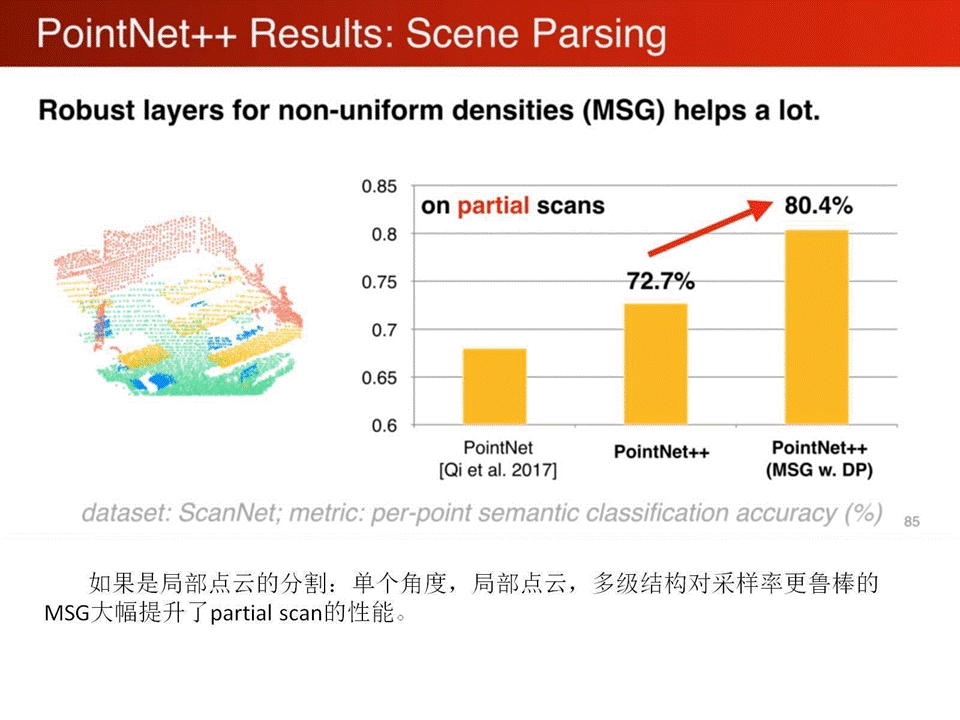
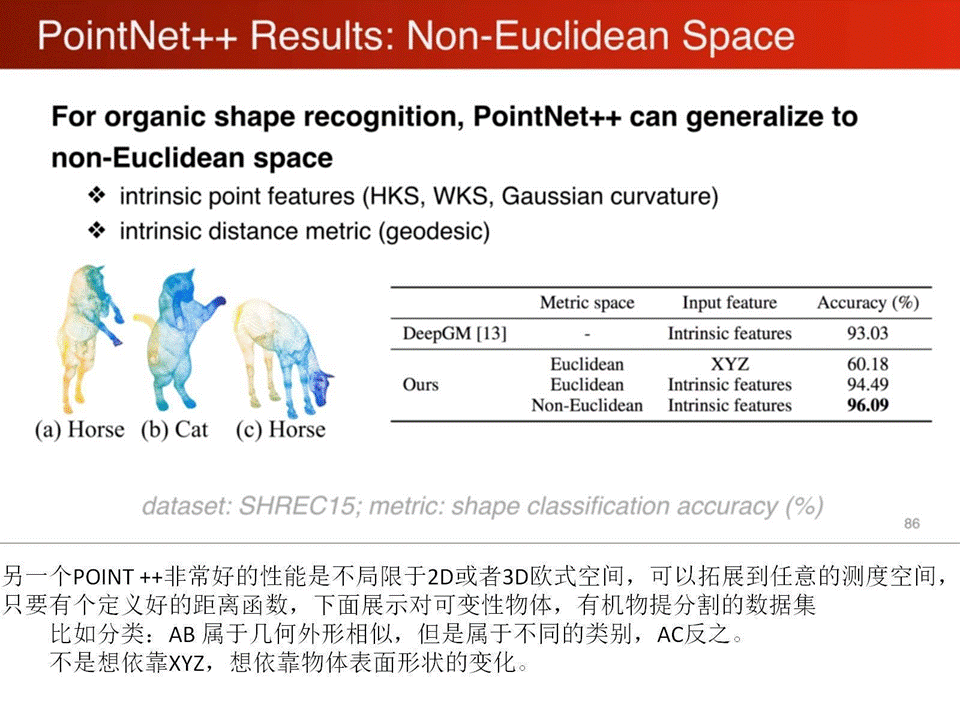
1、pointnet局部特征学习,平移和视角变换的不变性。
2、部分填充数据丢失的鲁棒性。
3、采样率变化的鲁棒性。
4、外形相似的分类鲁棒性。
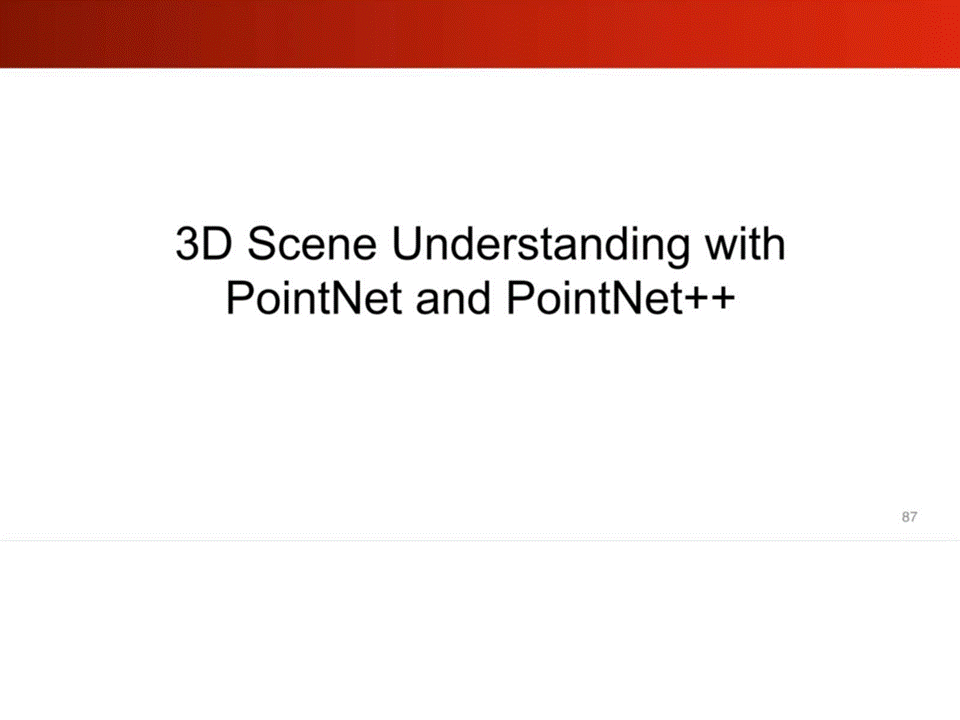
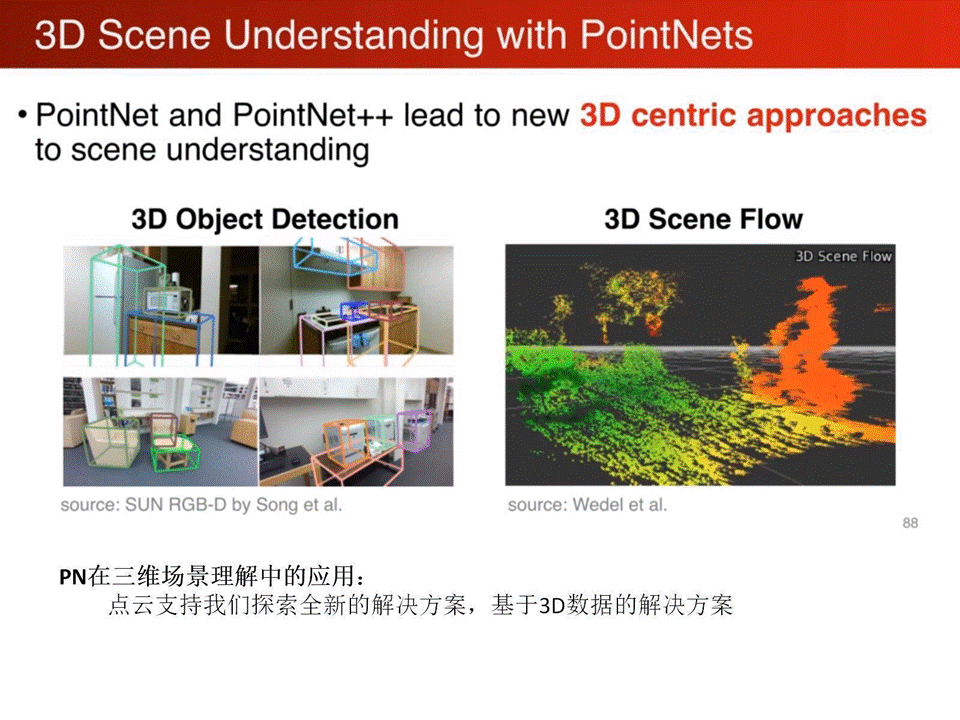
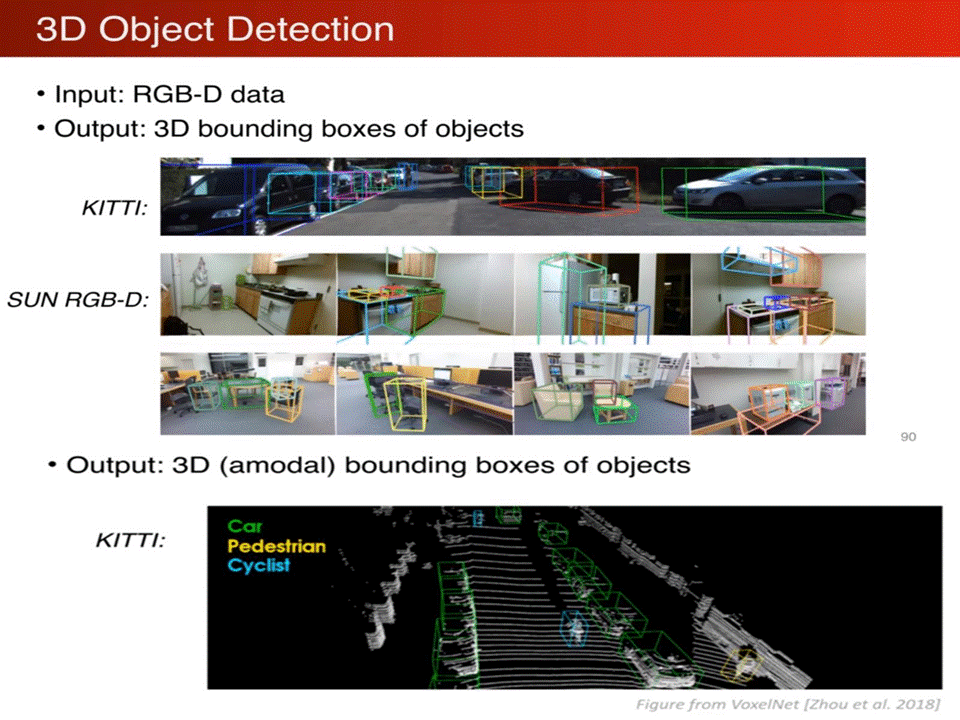
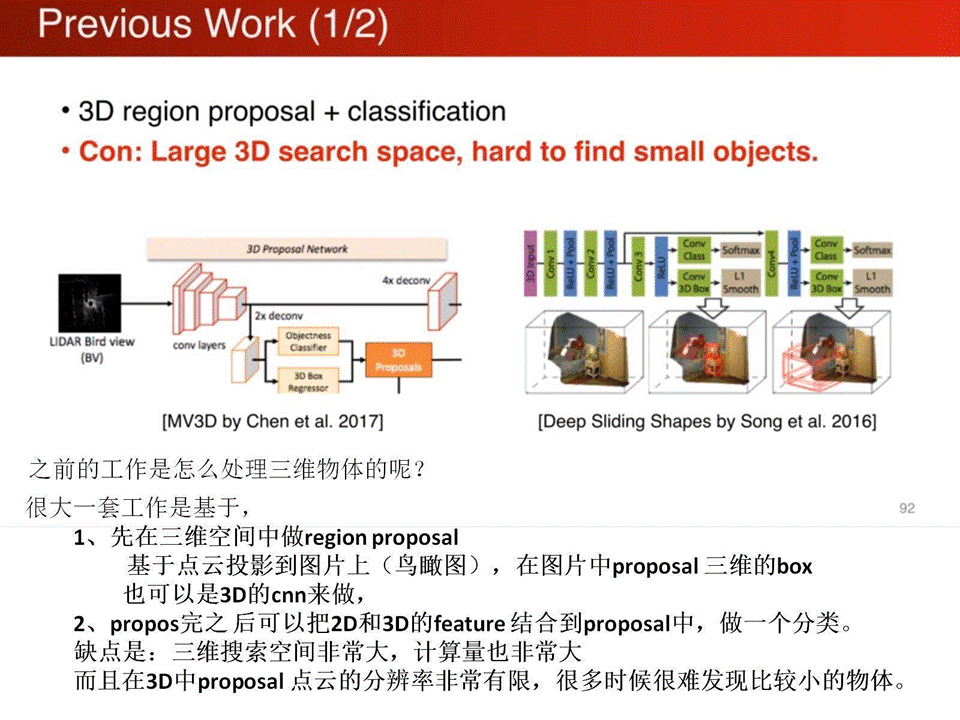
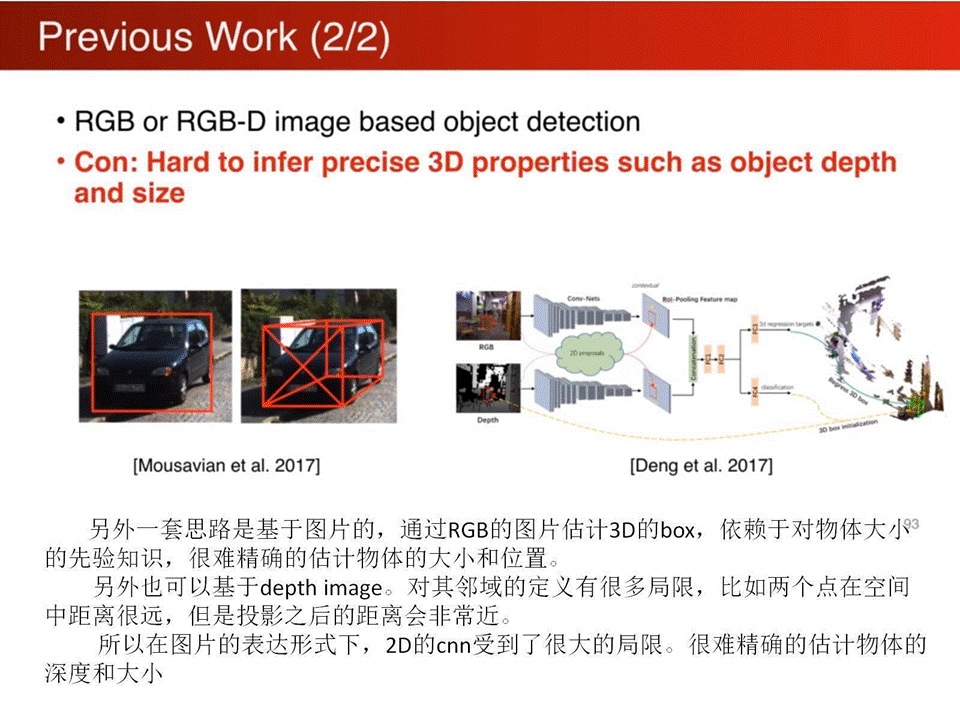
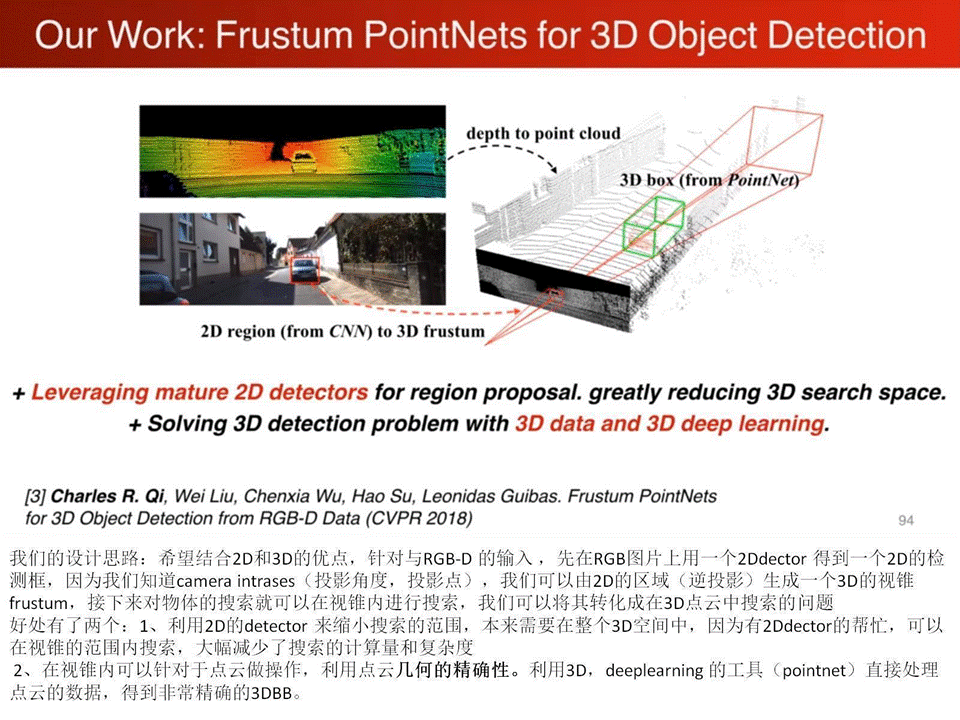
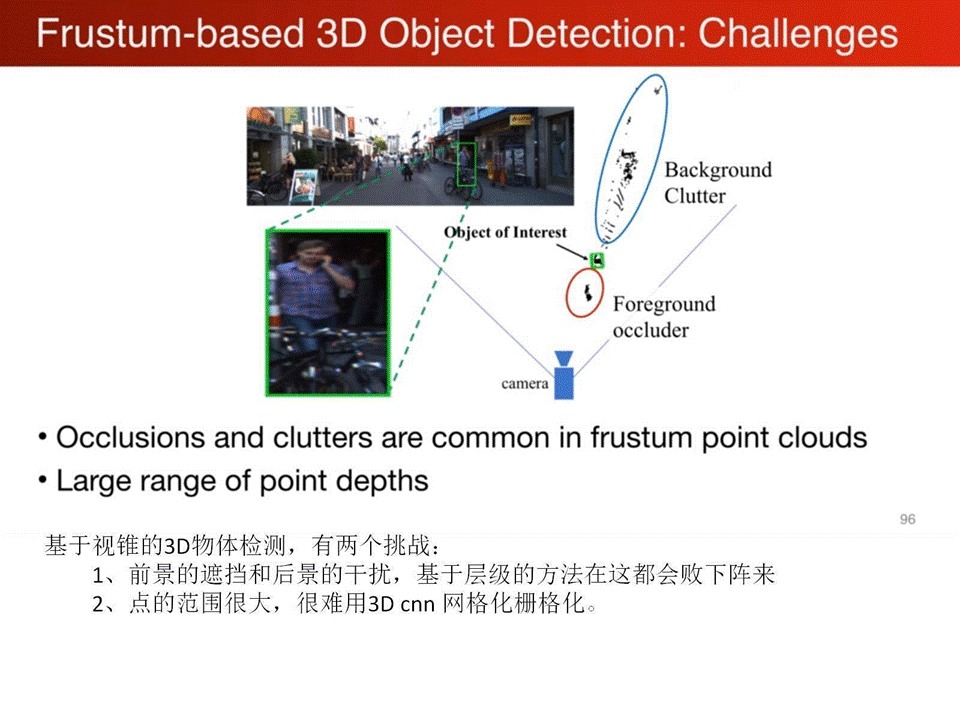
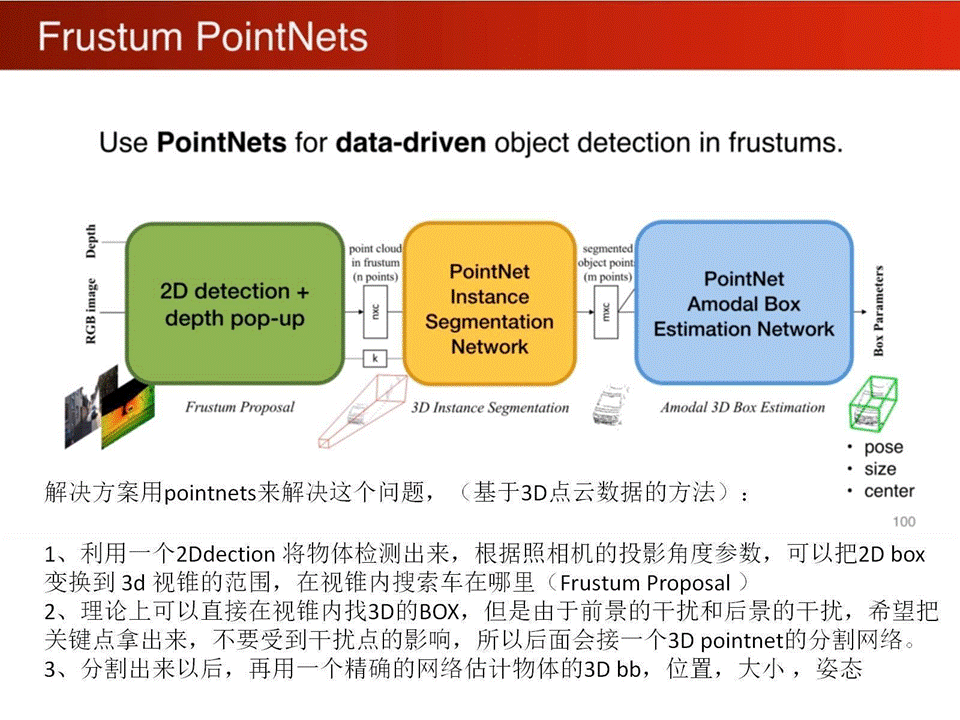
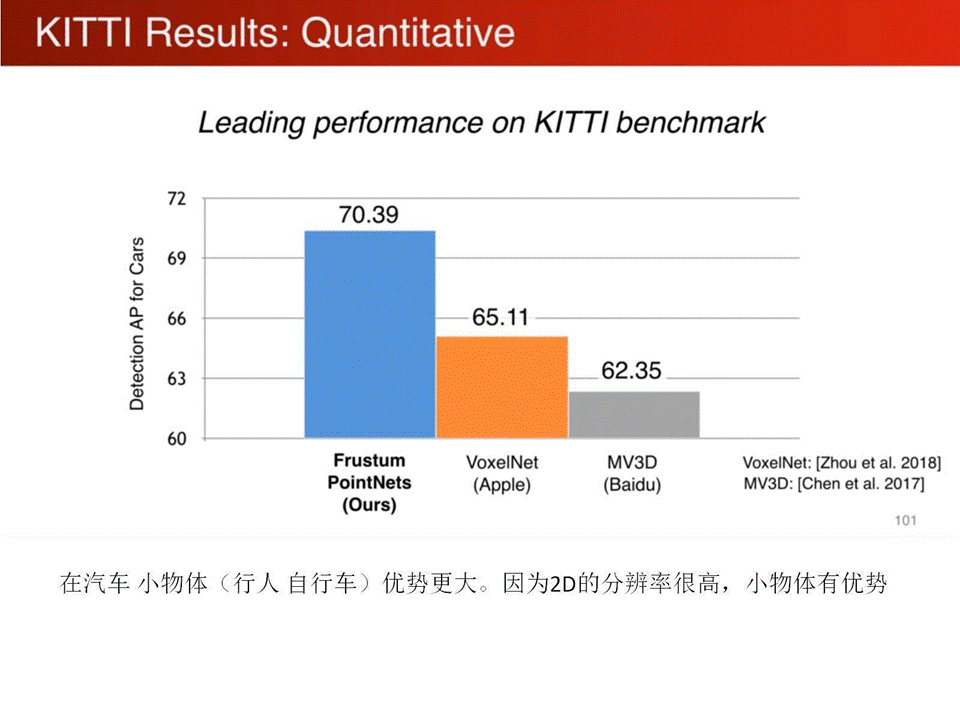
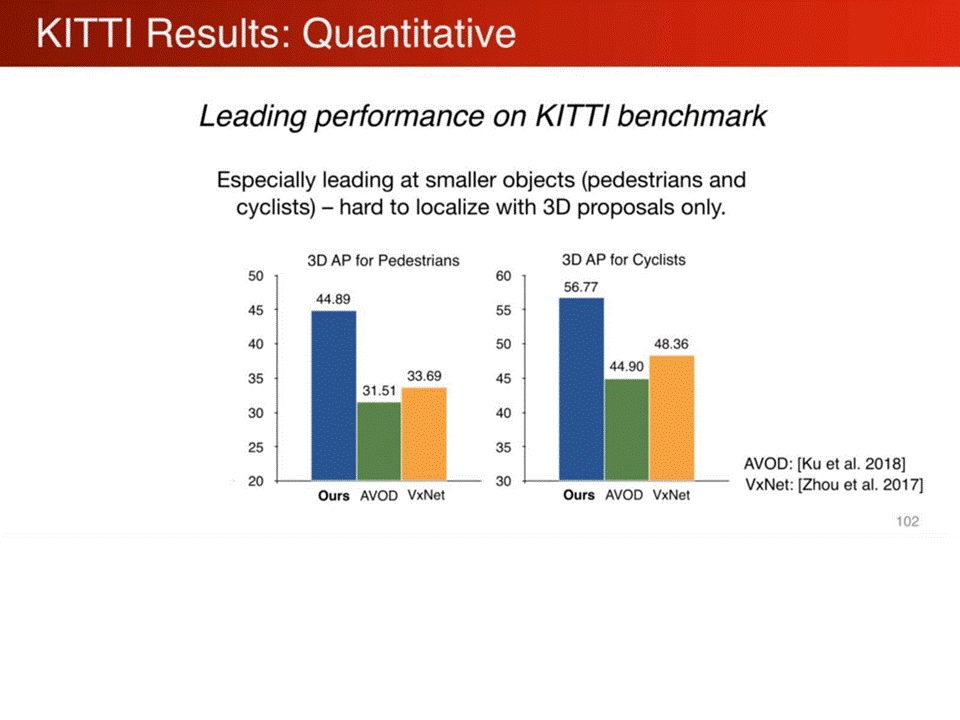
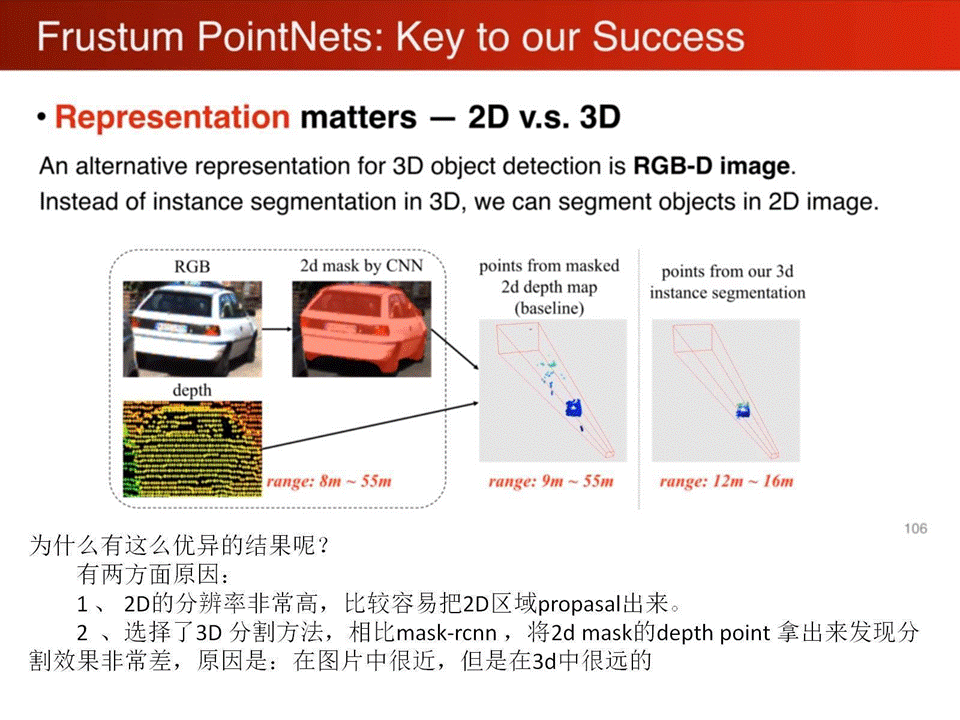
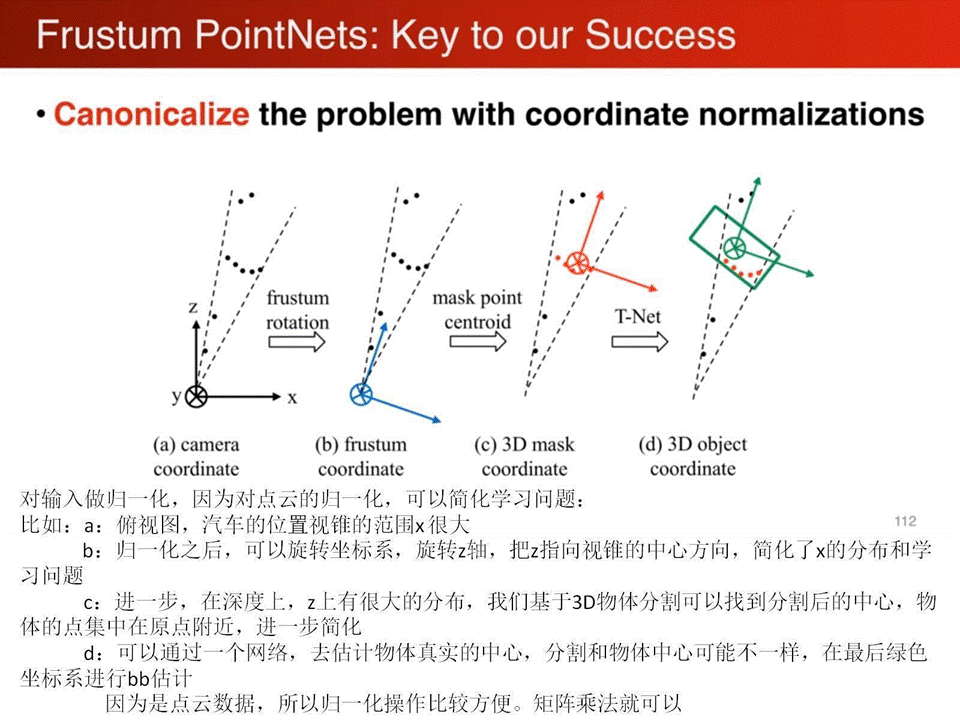
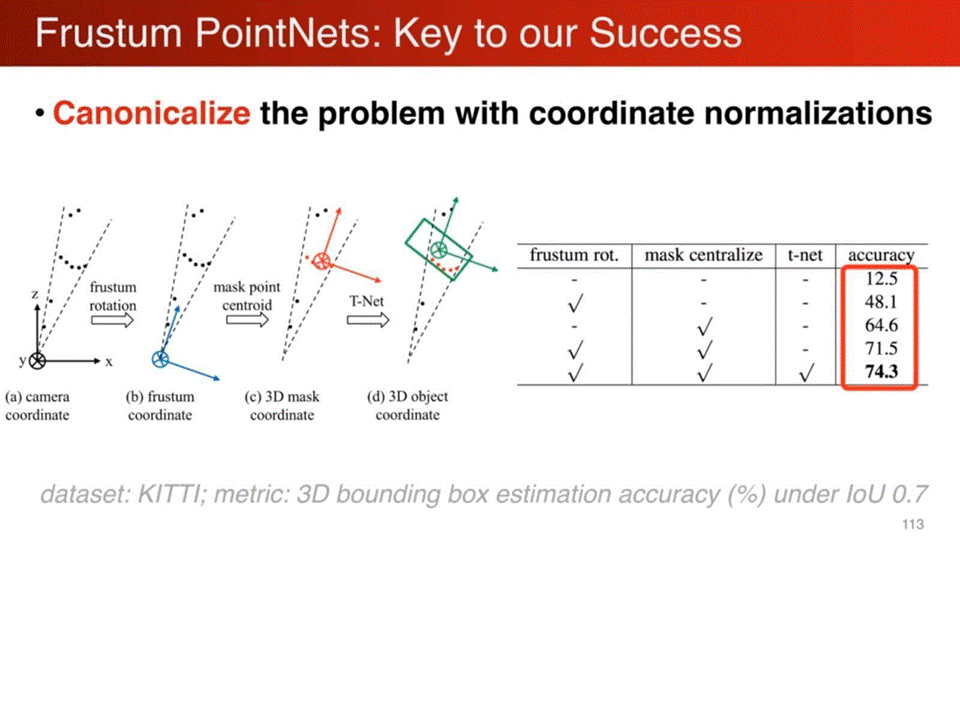
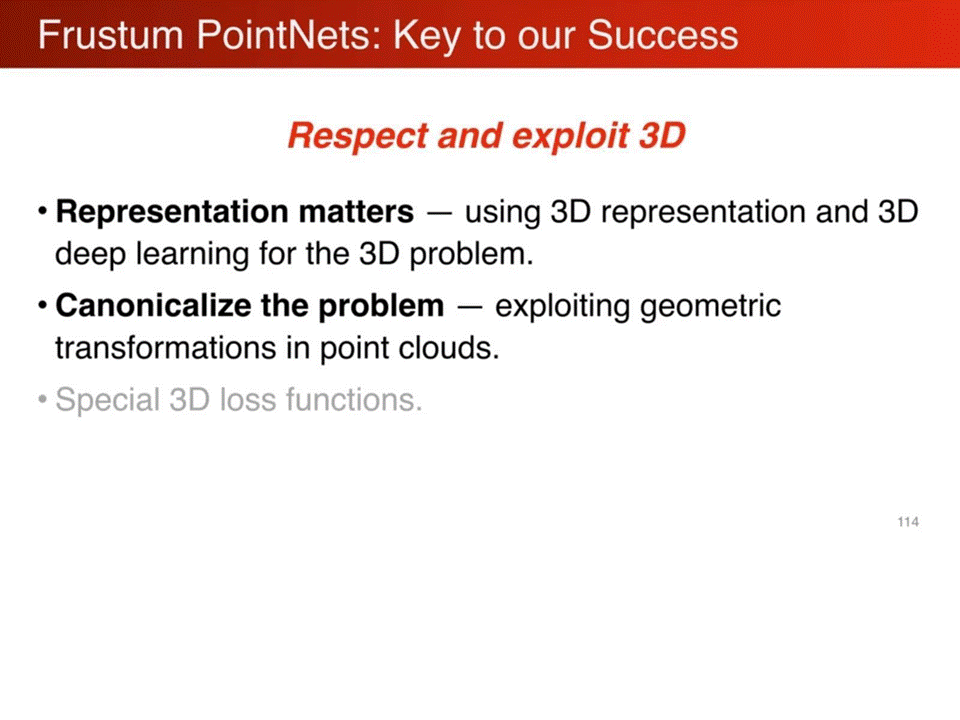
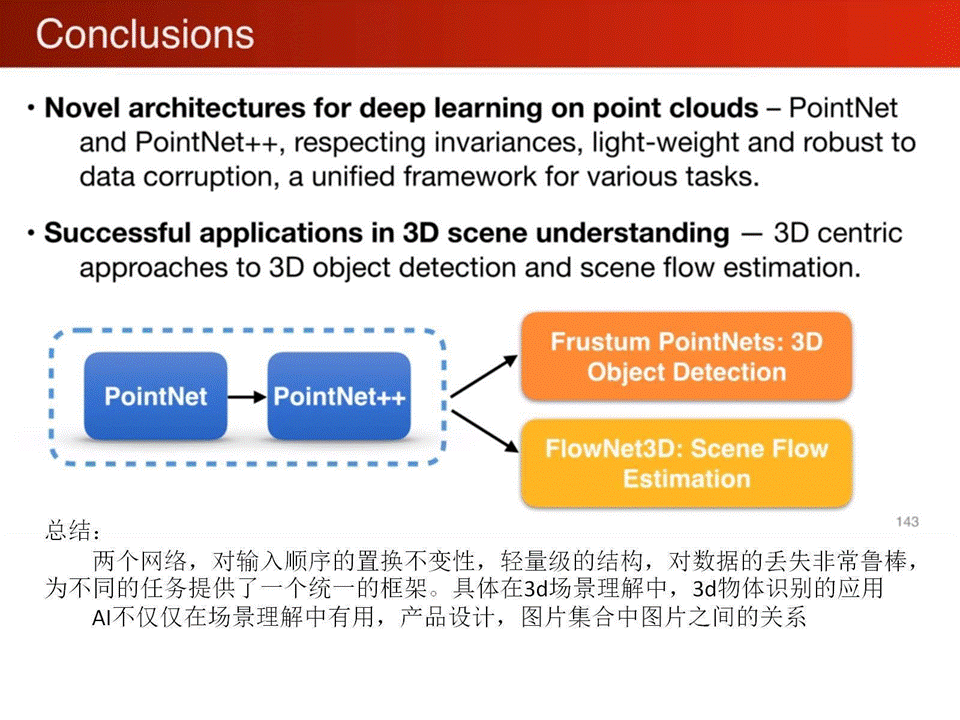
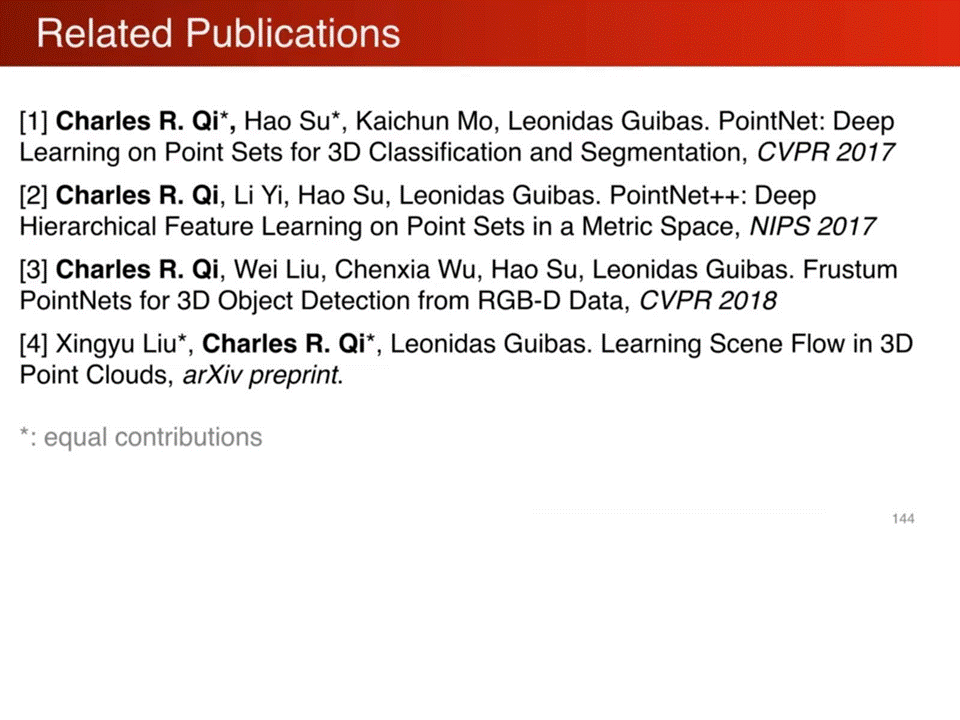
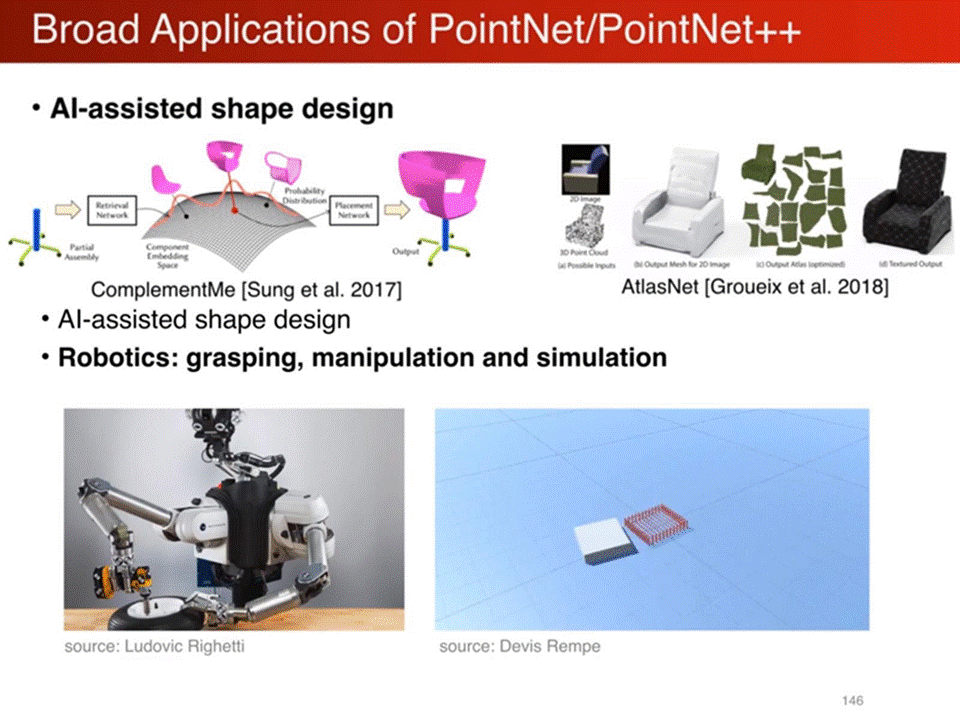
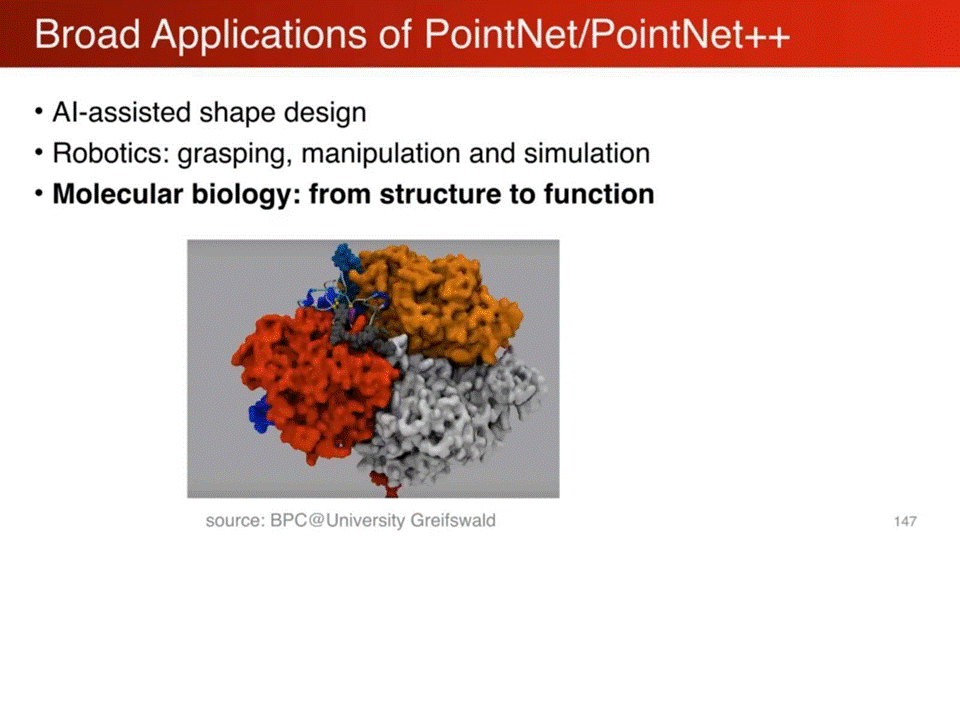
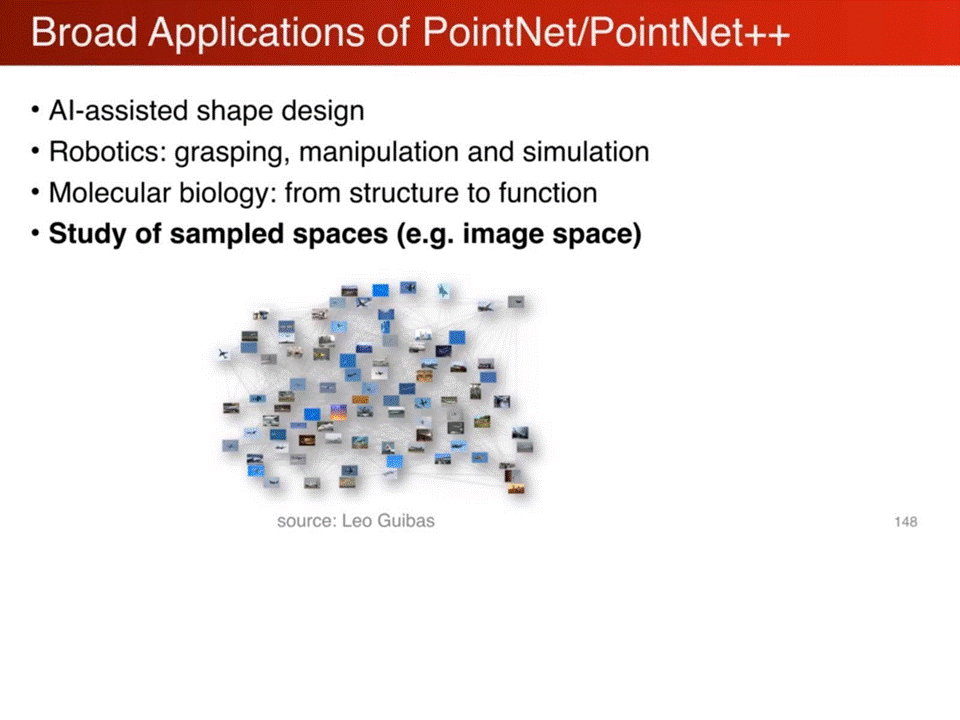
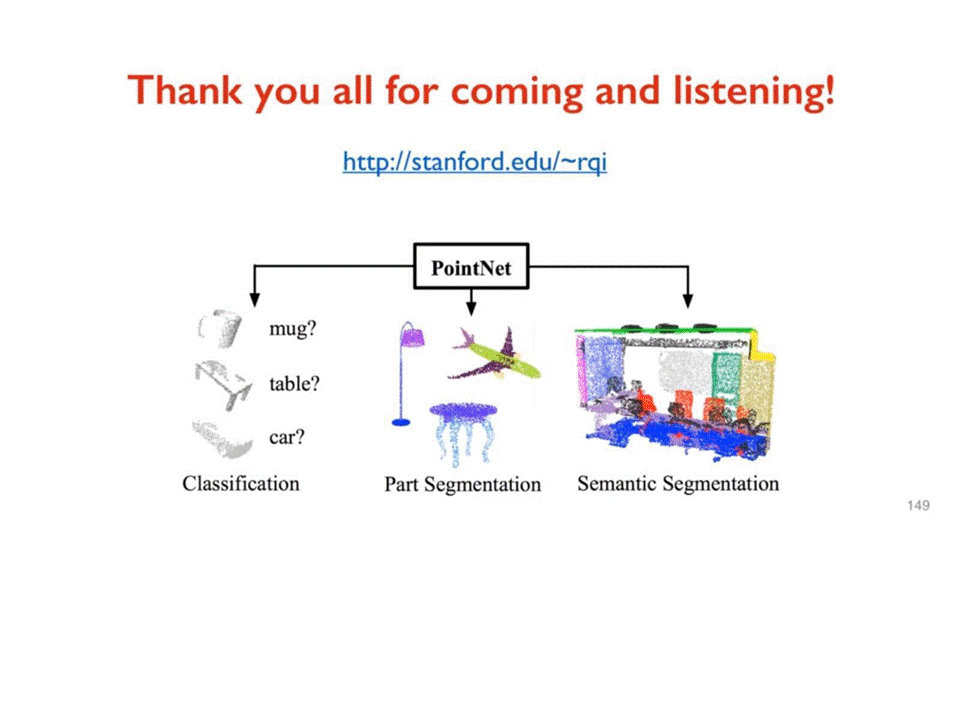
FQA:
1、pointnet没有考虑点与点之间的关系
2、关键点是可视化出来的
3、未来的主流是结合2D和3D,3D有精确的几何信息,但是它的分辨率比较低,近期传感器还不能跟上图像的分辨率,图像有很高的分辨率,还有精细的textures(纹理)
4、激光雷达采样是近密远疏,pointnet++本身有些能够处理采样不均的问题,如果你知道点云的分布,你可以加一些位置,加一些权重,调节权重。
5、未来发展趋势?point net++结果不太稳定,怎么取的最佳结果?一定程度由modelnet的datasize限制的,建议对test shape多次旋转取平均的结果。
6、pointnet++中的code中没有用到T-Net,release code 中没有用到T-Net,因为他们发现,在modelnet中T-Net对分类性能提升不大,pointnet++已经学到了局部特征,所以它对旋转不敏感。
7、pointnet++具有对局部几何变换的不变性吗?局部加一些T-Net结构是不合理的,因为局部变换了,并不能保证不同局部的变换是统一的,如果要实现统一性,实际上需要一些capsulenet 的work。
8、点云特征提取和2D的颜色信息有关吗?在最简单的假设下可以是无关的,光是xyz的坐标就已经提高了几何的信息,理论上不用其他的信息就已经完成了很多的任务,RGB是锦上添花的特征。
9、为什么用1024维这么高的数据集?bottle net layer 这一维是很关键的,太小,会限制网络的capability能力。
10、为什么采用FPS这种采样方法?因为这种采样方法是采尽可能远的点,最终实现的结果是对空间的比较均匀的降采样,希望达到均匀采样的结果,其他方法有很多,比如随机采样,但是效果可能没有FPS方法好,最近也有这方面的许多工作,这里的研究空间比较大。
11、点云的表达会是3D未来的方向吗?会是一个主要的方向,3D本身的应用场景很多,不同的场景对不同的数据类型有不同的倾向,在3D的场景理解中点云是重要的形式,在其他中就不一定了,比如建模中、可视化中、仿真中可能会倾向于不同的数据类型。
12、如何看待GCN在点云中的应用?GCN和pointnet++有很多相通的地方,两者都是在3D中寻找局部,然后在局部定义某种操作,然后形成多级的网络架构,其实是非常相似的,甚至可以相互变换。point net++的优点是,不仅限制在2D和3D,也适合3D,有的网络结构只能处理3D。