Batch Normalization: 使用tf.layers高级函数来构建带有Batch Normalization的神经网络
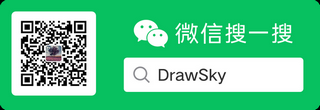



参考文献
吴恩达deeplearningai课程
课程笔记
Udacity课程
在使用tf.layers高级函数来构建神经网络中我们使用了tf.layers包构建了一个不包含有Batch Normalization结构的卷积神经网络模型作为本节模型的对比
本节中将使用tf.layers包实现包含有Batch Normalization的卷积神经网络模型
"""
向生成全连接层的'fully_connected'函数中添加Batch Normalization,我们需要以下步骤:
1.在函数声明中添加'is_training'参数,以确保可以向Batch Normalization层中传递信息
2.去除函数中bias偏置属性和激活函数
3.使用'tf.layers.batch_normalization'来标准化神经层的输出,注意,将“is_training”传递给该层,以确保网络适时更新数据集均值和方差统计信息。
4.将经过Batch Normalization后的值传递到ReLU激活函数中
"""
import tensorflow as tf
from tensorflow.examples.tutorials.mnist import input_data
mnist = input_data.read_data_sets("MNIST_data/", one_hot=True, reshape=False)
def fully_connected(prev_layer, num_units, is_training):
"""
num_units参数传递该层神经元的数量,根据prev_layer参数传入值作为该层输入创建全连接神经网络。
:param prev_layer: Tensor
该层神经元输入
:param num_units: int
该层神经元结点个数
:param is_training: bool or Tensor
表示该网络当前是否正在训练,告知Batch Normalization层是否应该更新或者使用均值或方差的分布信息
:returns Tensor
一个新的全连接神经网络层
"""
layer = tf.layers.dense(prev_layer, num_units, use_bias=False, activation=None)
layer = tf.layers.batch_normalization(layer, training=is_training)
layer = tf.nn.relu(layer)
return layer
"""
向生成卷积层的'conv_layer'函数中添加Batch Normalization,我们需要以下步骤:
1.在函数声明中添加'is_training'参数,以确保可以向Batch Normalization层中传递信息
2.去除conv2d层中bias偏置属性和激活函数
3.使用'tf.layers.batch_normalization'来标准化卷积层的输出,注意,将"is_training"传递给该层,以确保网络适时更新数据集均值和方差统计信息。
4.将经过Batch Normalization后的值传递到ReLU激活函数中
PS:和'fully_connected'函数比较,你会发现如果你使用tf.layers包函数对全连接层进行BN操作和对卷积层进行BN操作没有任何的区别,但是如果使用tf.nn包中函数实现BN会发现一些小的变动
"""
"""
我们会运用以下方法来构建神经网络的卷积层,这个卷积层很基本,我们总是使用3x3内核,ReLU激活函数,
在具有奇数深度的图层上步长为1x1,在具有偶数深度的图层上步长为2x2。在这个网络中,我们并不打算使用池化层。
PS:该版本的函数包括批量标准化操作。
"""
def conv_layer(prev_layer, layer_depth, is_training):
"""
使用给定的参数作为输入创建卷积层
:param prev_layer: Tensor
传入该层神经元作为输入
:param layer_depth: int
我们将根据网络中图层的深度设置特征图的步长和数量。
这不是实践CNN的好方法,但它可以帮助我们用很少的代码创建这个示例。
:param is_training: bool or Tensor
表示该网络当前是否正在训练,告知Batch Normalization层是否应该更新或者使用均值或方差的分布信息
:returns Tensor
一个新的卷积层
"""
strides = 2 if layer_depth%3 == 0 else 1
conv_layer = tf.layers.conv2d(prev_layer, layer_depth*4, 3, strides, 'same', use_bias=False, activation=None)
conv_layer = tf.layers.batch_normalization(conv_layer, training=is_training)
conv_layer = tf.nn.relu(conv_layer)
return conv_layer
"""
批量标准化仍然是一个新的想法,研究人员仍在发现如何最好地使用它。
一般来说,人们似乎同意删除层的偏差(因为批处理已经有了缩放和移位的术语),并且在层的非线性激活函数之前添加了批处理规范化。
然而,对于某些网络来说,使用其他的方法也能得到不错的结果
为了演示这一点,以下三个版本的conv_layer展示了实现批量标准化的其他方法。
如果您尝试使用这些函数的任何一个版本,它们都应该仍然运行良好(尽管有些版本可能仍然比其他版本更好)。
"""
# 在卷积层中使用偏置use_bias=True,在ReLU激活函数之前仍然添加了批处理规范化。
# def conv_layer(prev_layer, layer_num, is_training):
# strides = 2 if layer_num%3 == 0 else 1
# conv_layer = tf.layers.conv2d(prev_layer, layer_num*4, 3, strides, 'same', use_bias=True, activation=None)
# conv_layer = tf.layers.batch_normalization(conv_layer, training=is_training)
# conv_layer = tf.nn.relu(conv_layer)
# return conv_layer
# 在卷积层中使用偏置use_bias=True,先使用ReLU激活函数处理然后添加了批处理规范化。
# def conv_layer(prev_layer, layer_num, is_training):
# strides = 2 if layer_num % 3 == 0 else 1
# conv_layer = tf.layers.conv2d(prev_layer, layer_num*4, 3, strides, 'same', use_bias=True, activation=tf.nn.relu)
# conv_layer = tf.layers.batch_normalization(conv_layer, training=is_training)
# return conv_layer
# 在卷积层中不使用偏置use_bias=False,但先使用ReLU激活函数处理然后添加了批处理规范化。
# def conv_layer(prev_layer, layer_num, is_training):
# strides = 2 if layer_num % 3 == 0 else 1
# conv_layer = tf.layers.conv2d(prev_layer, layer_num*4, 3, strides, 'same', use_bias=False, activation=tf.nn.relu)
# conv_layer = tf.layers.batch_normalization(conv_layer, training=is_training)
# return conv_layer
"""
为了修改训练函数,我们需要做以下工作:
1.Added is_training, a placeholder to store a boolean value indicating whether or not the network is training.
添加is_training,一个用于存储布尔值的占位符,该值指示网络是否正在训练
2.Passed is_training to the conv_layer and fully_connected functions.
传递is_training到conv_layer和fully_connected函数
3.Each time we call run on the session, we added to feed_dict the appropriate value for is_training
每次调用sess.run函数时,我们都添加到feed_dict中is_training的适当值用以表示当前是正在训练还是预测
4.Moved the creation of train_opt inside a with tf.control_dependencies... statement.
This is necessary to get the normalization layers created with tf.layers.batch_normalization to update their population statistics,
which we need when performing inference.
将train_opt训练函数放进with tf.control_dependencies... 的函数结构体中
这是我们得到由tf.layers.batch_normalization创建的BN层的值所必须的操作,我们由这个操作来更新训练数据的统计分布,使在inference前向传播预测时使用正确的数据分布值
"""
def train(num_batches, batch_size, learning_rate):
# Build placeholders for the input samples and labels
# 创建输入样本和标签的占位符
inputs = tf.placeholder(tf.float32, [None, 28, 28, 1])
labels = tf.placeholder(tf.float32, [None, 10])
# Add placeholder to indicate whether or not we're training the model
# 创建占位符表明当前是否正在训练模型
is_training = tf.placeholder(tf.bool)
# Feed the inputs into a series of 20 convolutional layers
# 把输入数据填充到一系列20个卷积层的神经网络中
layer = inputs
for layer_i in range(1, 20):
layer = conv_layer(layer, layer_i, is_training)
# Flatten the output from the convolutional layers
# 将卷积层输出扁平化处理
orig_shape = layer.get_shape().as_list()
layer = tf.reshape(layer, shape=[-1, orig_shape[1]*orig_shape[2]*orig_shape[3]])
# Add one fully connected layer
# 添加一个具有100个神经元的全连接层
layer = fully_connected(layer, 100, is_training)
# Create the output layer with 1 node for each
# 为每一个类别添加一个输出节点
logits = tf.layers.dense(layer, 10)
# Define loss and training operations
# 定义loss 函数和训练操作
model_loss = tf.reduce_mean(tf.nn.sigmoid_cross_entropy_with_logits(logits=logits, labels=labels))
# Tell TensorFlow to update the population statistics while training
# 通知Tensorflow在训练时要更新均值和方差的分布
with tf.control_dependencies(tf.get_collection(tf.GraphKeys.UPDATE_OPS)):
train_opt = tf.train.AdamOptimizer(learning_rate).minimize(model_loss)
# Create operations to test accuracy
# 创建计算准确度的操作
correct_prediction = tf.equal(tf.argmax(logits, 1), tf.argmax(labels, 1))
accuracy = tf.reduce_mean(tf.cast(correct_prediction, tf.float32))
# Train and test the network
# 训练并测试网络模型
with tf.Session() as sess:
sess.run(tf.global_variables_initializer())
for batch_i in range(num_batches):
batch_xs, batch_ys = mnist.train.next_batch(batch_size)
# train this batch
# 训练样本批次
sess.run(train_opt, {inputs: batch_xs, labels: batch_ys, is_training: True})
# Periodically check the validation or training loss and accuracy
# 定期检查训练或验证集上的loss和精确度
if batch_i%100 == 0:
loss, acc = sess.run([model_loss, accuracy], {inputs: mnist.validation.images,
labels: mnist.validation.labels,
is_training: False})
print(
'Batch: {:>2}: Validation loss: {:>3.5f}, Validation accuracy: {:>3.5f}'.format(batch_i, loss, acc))
elif batch_i%25 == 0:
loss, acc = sess.run([model_loss, accuracy], {inputs: batch_xs, labels: batch_ys, is_training: False})
print('Batch: {:>2}: Training loss: {:>3.5f}, Training accuracy: {:>3.5f}'.format(batch_i, loss, acc))
# At the end, score the final accuracy for both the validation and test sets
# 最后在验证集和测试集上对模型准确率进行评分
acc = sess.run(accuracy, {inputs: mnist.validation.images,
labels: mnist.validation.labels,
is_training: False})
print('Final validation accuracy: {:>3.5f}'.format(acc))
acc = sess.run(accuracy, {inputs: mnist.test.images,
labels: mnist.test.labels,
is_training: False})
print('Final test accuracy: {:>3.5f}'.format(acc))
# Score the first 100 test images individually, just to make sure batch normalization really worked
# 对100个独立的测试图片进行评分,对比验证Batch Normalization的效果
correct = 0
for i in range(100):
correct += sess.run(accuracy, feed_dict={inputs: [mnist.test.images[i]],
labels: [mnist.test.labels[i]],
is_training: False})
print("Accuracy on 100 samples:", correct/100)
num_batches = 800 # 迭代次数
batch_size = 64 # 批处理数量
learning_rate = 0.002 # 学习率
tf.reset_default_graph()
with tf.Graph().as_default():
train(num_batches, batch_size, learning_rate)
"""
通过批量标准化,我们现在获得了出色的性能。
事实上,在仅仅500个批次之后,验证精度几乎达到94%。
还要注意输出的最后一行:100个样本的精确性。
如果这个值很低,而其他一切看起来都很好,那意味着您没有正确地实现批量标准化。
具体地说,这意味着你要么在训练时没有计算总体均值和方差,要么在推理过程中没有使用这些值。
"""
# Extracting MNIST_data/train-images-idx3-ubyte.gz
# Extracting MNIST_data/train-labels-idx1-ubyte.gz
# Extracting MNIST_data/t10k-images-idx3-ubyte.gz
# Extracting MNIST_data/t10k-labels-idx1-ubyte.gz
# 2018-03-18 18:35:03.506132: I D:Build ensorflow ensorflow-r1.4 ensorflowcoreplatformcpu_feature_guard.cc:137] Your CPU supports instructions that this TensorFlow binary was not compiled to use: AVX
# Batch: 0: Validation loss: 0.69091, Validation accuracy: 0.10700
# Batch: 25: Training loss: 0.57651, Training accuracy: 0.14062
# Batch: 50: Training loss: 0.46147, Training accuracy: 0.09375
# Batch: 75: Training loss: 0.38943, Training accuracy: 0.03125
# Batch: 100: Validation loss: 0.35058, Validation accuracy: 0.11260
# Batch: 125: Training loss: 0.33055, Training accuracy: 0.17188
# Batch: 150: Training loss: 0.32800, Training accuracy: 0.15625
# Batch: 175: Training loss: 0.34861, Training accuracy: 0.18750
# Batch: 200: Validation loss: 0.40572, Validation accuracy: 0.11260
# Batch: 225: Training loss: 0.33194, Training accuracy: 0.23438
# Batch: 250: Training loss: 0.46818, Training accuracy: 0.25000
# Batch: 275: Training loss: 0.38155, Training accuracy: 0.43750
# Batch: 300: Validation loss: 0.25433, Validation accuracy: 0.55320
# Batch: 325: Training loss: 0.17981, Training accuracy: 0.73438
# Batch: 350: Training loss: 0.18110, Training accuracy: 0.76562
# Batch: 375: Training loss: 0.06763, Training accuracy: 0.92188
# Batch: 400: Validation loss: 0.04946, Validation accuracy: 0.92360
# Batch: 425: Training loss: 0.07999, Training accuracy: 0.89062
# Batch: 450: Training loss: 0.04927, Training accuracy: 0.93750
# Batch: 475: Training loss: 0.00216, Training accuracy: 1.00000
# Batch: 500: Validation loss: 0.04071, Validation accuracy: 0.94060
# Batch: 525: Training loss: 0.01940, Training accuracy: 0.98438
# Batch: 550: Training loss: 0.05709, Training accuracy: 0.90625
# Batch: 575: Training loss: 0.04652, Training accuracy: 0.93750
# Batch: 600: Validation loss: 0.05811, Validation accuracy: 0.91580
# Batch: 625: Training loss: 0.01401, Training accuracy: 0.96875
# Batch: 650: Training loss: 0.04626, Training accuracy: 0.93750
# Batch: 675: Training loss: 0.03831, Training accuracy: 0.95312
# Batch: 700: Validation loss: 0.03709, Validation accuracy: 0.94960
# Batch: 725: Training loss: 0.00235, Training accuracy: 1.00000
# Batch: 750: Training loss: 0.02916, Training accuracy: 0.96875
# Batch: 775: Training loss: 0.01792, Training accuracy: 0.98438
# Final validation accuracy: 0.94040
# Final test accuracy: 0.93840
# Accuracy on 100 samples: 0.95