一、问题提出
由于GPY进行了纠偏,所以在采集的时候,就已经获得了质量较高的答题卡图片
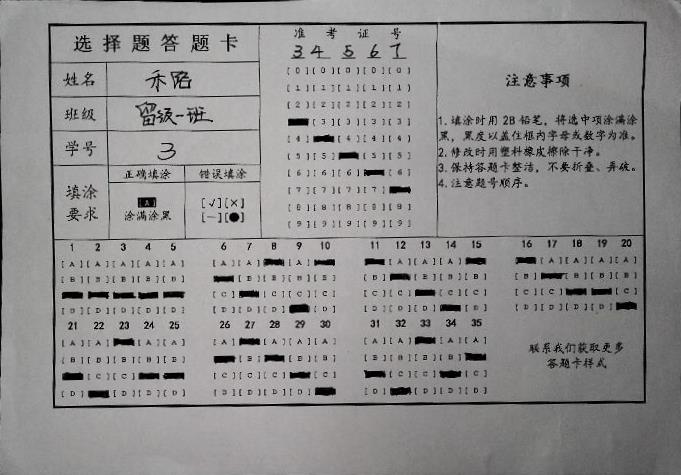
下一步就是需要从这张图片中,识别出人眼识别出来的那些信息,并且将这个过程尽可能地鲁棒化,提高识别的准确率。
二、思路探索
在从图片到数字的转变过程中,既是一个“量化”的过程,也是一个“降维”的过程,需要特定的角度非常重要。这就像很多人站在一起拍集体照,选择不同的角度能够得到这群人不同的像,高明的摄像师能够很快地找到角度,将所有的人都拍摄其中;我们图像处理程序也是同样的道理,有经验的工程师能够善于模式思考,快速找到解决方法的途径。
对于我们这里的这张答题卡图片,和之前的较为简单的答题卡想比较,有很多不同,比较两者的二值图片,就可以发现:
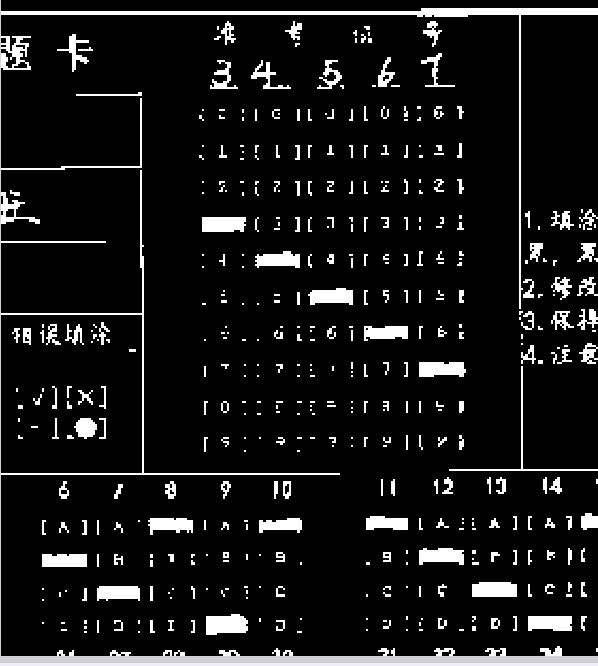
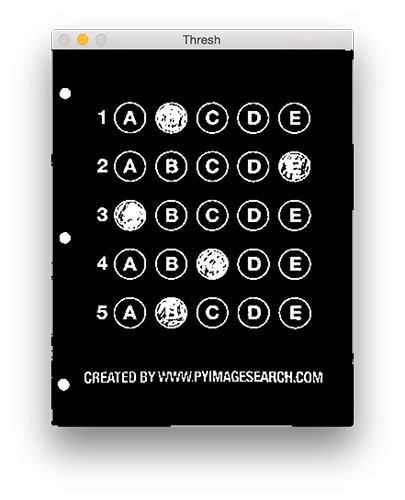
最大的不同在于没有可以供标定的基础点。因此我们必须采用其它的方法来进行定位。
此外,答题区域为矩形密集分布,因此我想到的是直接“网格化”进行处理。
三、算法过程和主要代码
step1:灰度-二值-形态学

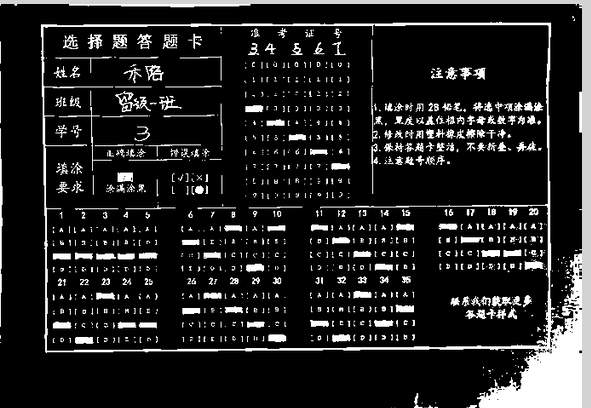
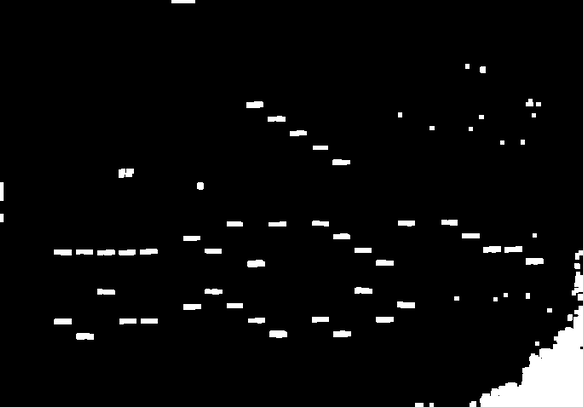
step2:轮廓分析
对识别出来的二值图像通过轮廓进一步地进行处理,得到下图的识别结果,就为下一步定量打下基础
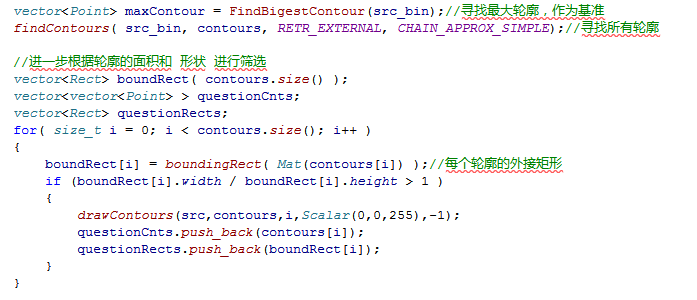
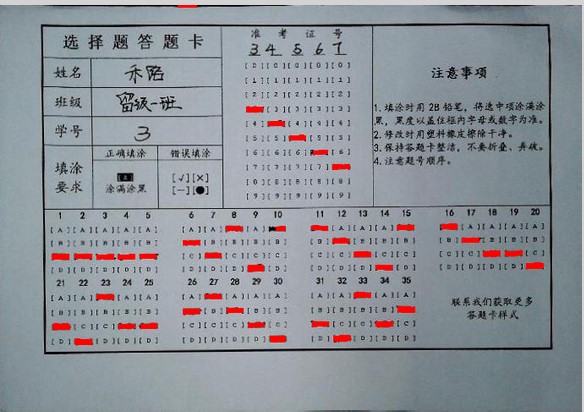
step3:模板匹配
想得到识别的结果,首先就是需要对现有的图片进行分割出来。在没有定位点的前提下,如何准确切割?
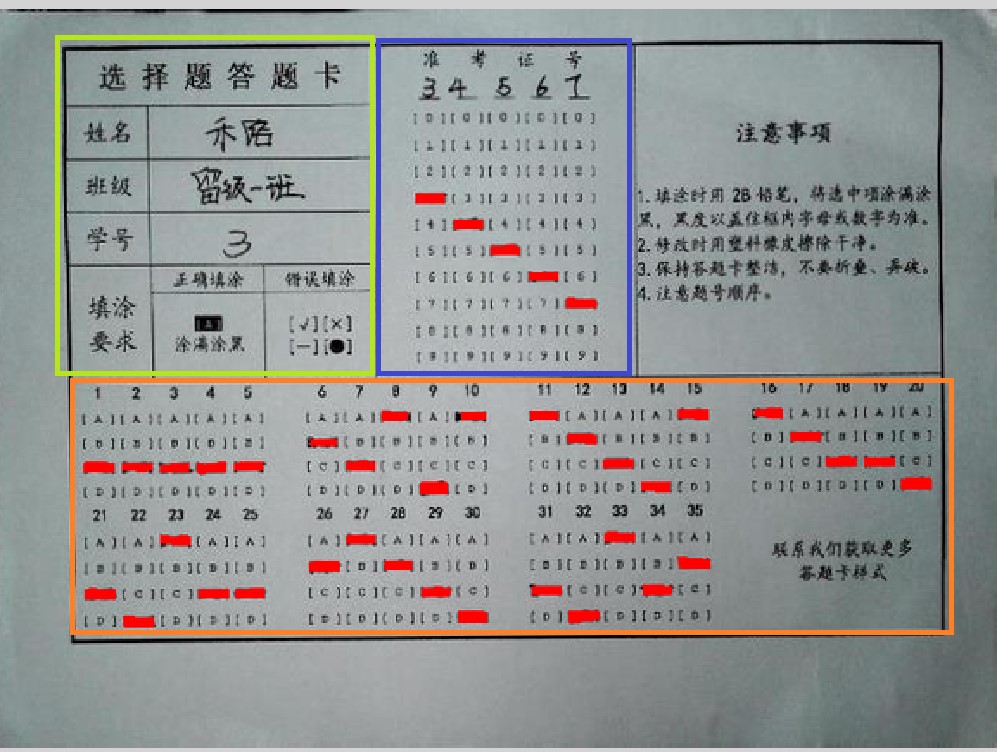
根据以前的经验,基本的思路是这样:
a、根据模板识别,准确的获得可定位地址(比如我选择使用“选择题答题卡”几个字作为模板,得到下图定位结果,注意图中白点);
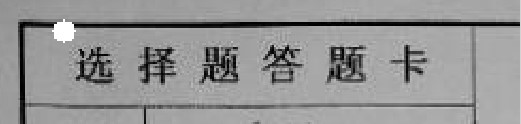
b、而后采用经典的“纵横”方法进行识别。(这里我对各个距离进行了测量和标注)
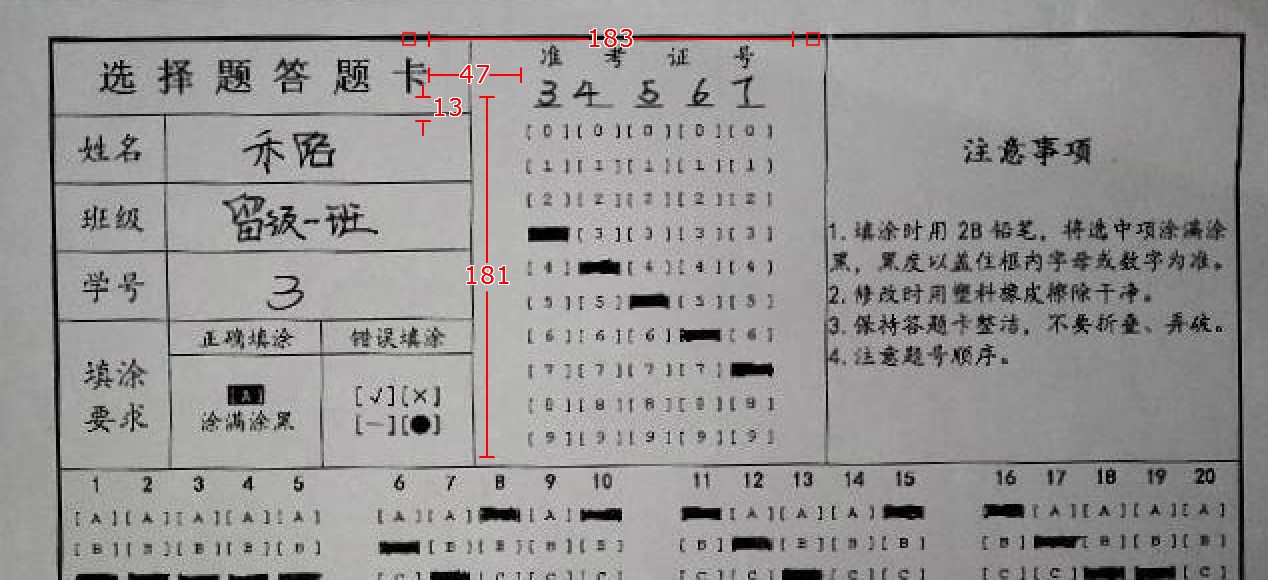
细节:
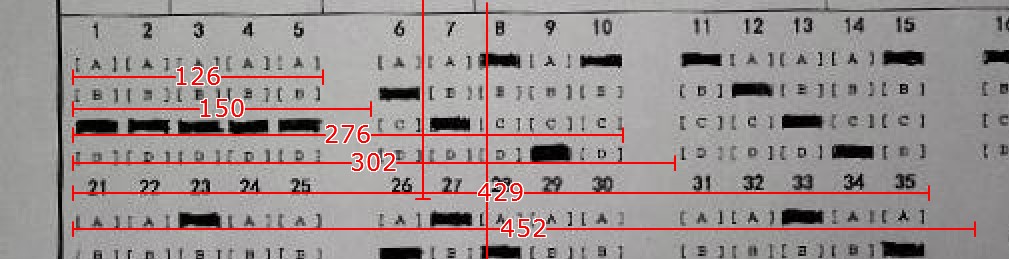
编写以下代码:
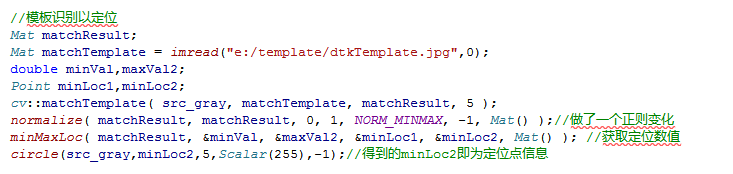
得到以下结果:

step4:分块面积识别
直接识别划分区域的面积,得到定量结果
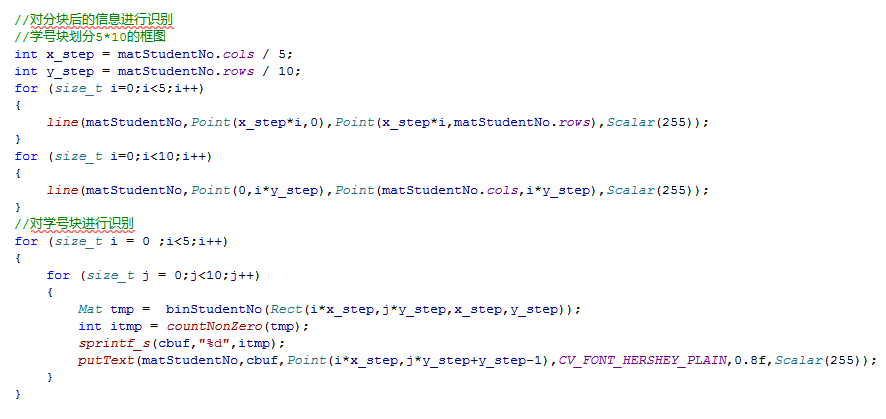
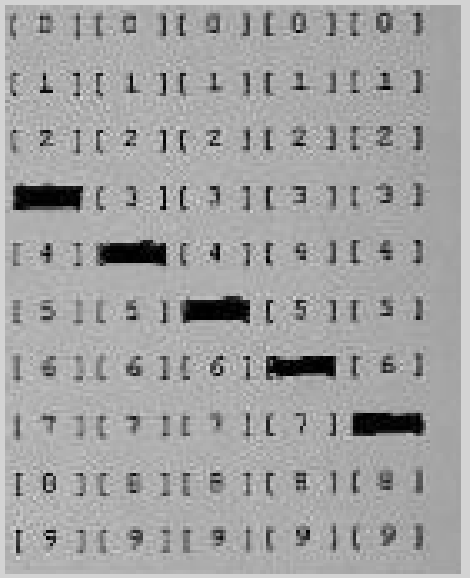
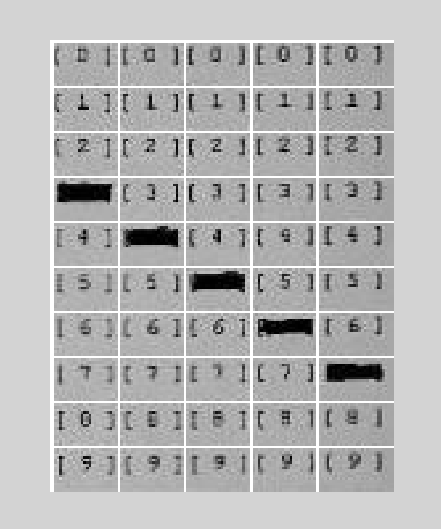
全图:
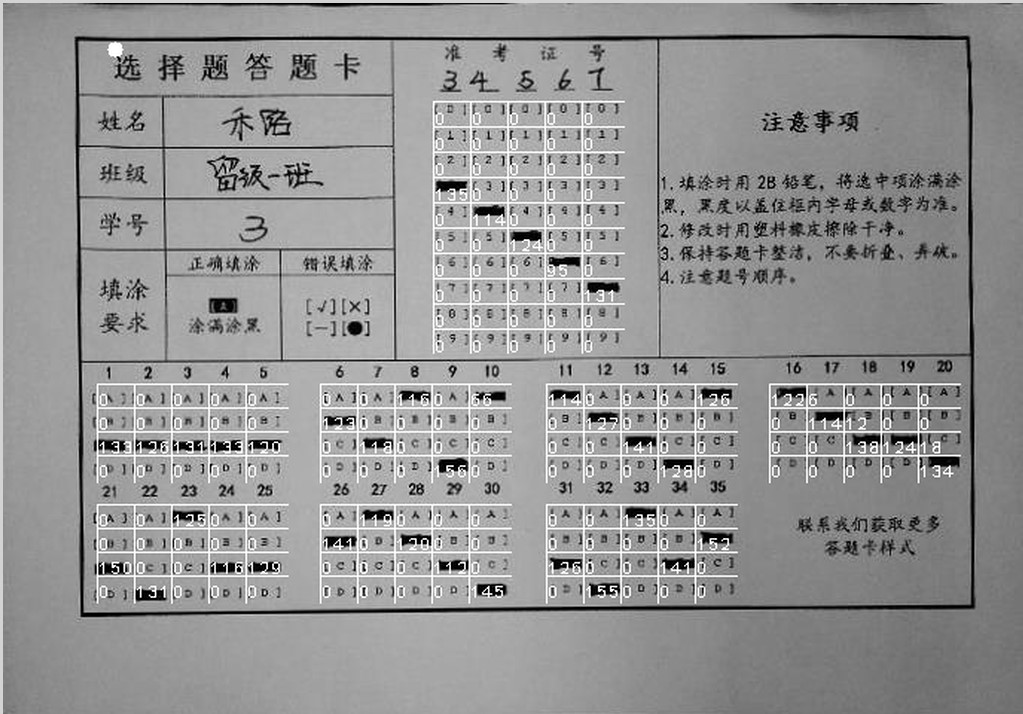
细节:
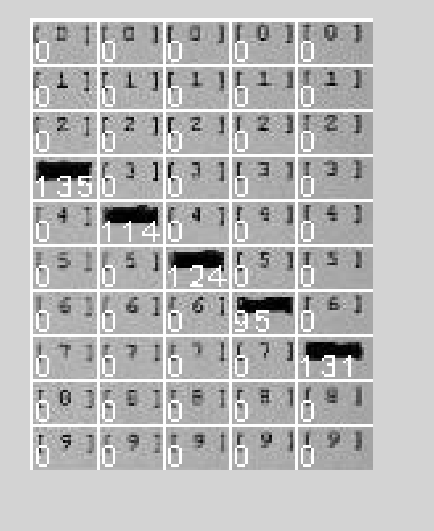
step5:转化得到识别结果
编写相关识别的结果为:
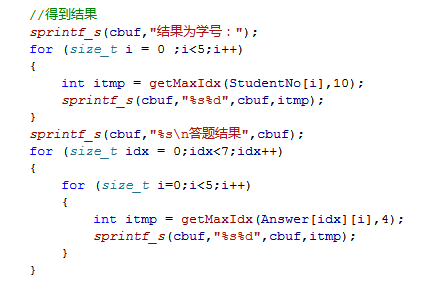
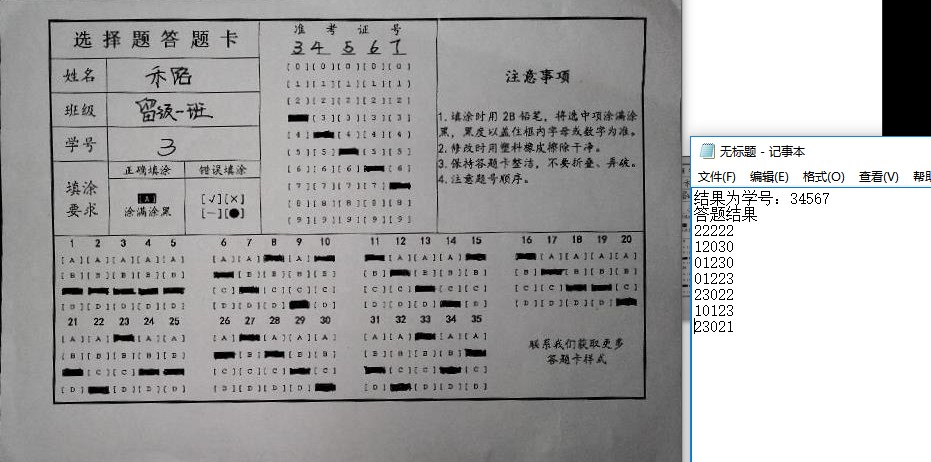
四、需要注意的地方:
1、模板识别对于不同尺度采集的图片,是否具有通用性。故我需要在不同的图片、不同的采集模式下进行探索;
2、目前识别出来的结果缺乏对答题结果“重复”“遗漏”情况分析;
3、我们看见的是彩色或者灰度图像,实际上,需要识别出来的是bin区域。在实验的过程中,我们会用到“彩色或者灰度”作为背景。
至此,复杂答题卡的算法部分基本完成。感谢阅读至此,希望有所帮助。