%% 蚁群算法及Matlab实现——TSP问题 % 《MATLAB数学建模方法与实践》(《MATLAB在数学建模中的应用》升级版),北航出版社,卓金武、王鸿钧编著. %% 数据准备 % 清空环境变量 clear all clc % 程序运行计时开始 t0 = clock; %导入数据 citys=xlsread('C题附件1.xlsx', 'B2:C31'); x=xlsread('C题附件1.xlsx','B2:B31'); y=xlsread('C题附件1.xlsx','C2:C31'); %% 计算城市间相互距离 n = size(citys,1); D = zeros(n,n); r=6371; for i = 1:n for j = 1:n if i ~= j D(i,j) = r*acos(cos(y(i))*cos(y(j))*cos(x(i)-x(j))+sin(y(i))*sin(y(j))); else D(i,j) = 1e-4; %设定的对角矩阵修正值 end end end %% 初始化参数 m = 75; % 蚂蚁数量 alpha = 1; % 信息素重要程度因子 beta = 5; % 启发函数重要程度因子 vol = 0.2; % 信息素挥发(volatilization)因子 Q = 10; % 常系数 Heu_F = 1./D; % 启发函数(heuristic function) Tau = ones(n,n); % 信息素矩阵 Table = zeros(m,n); % 路径记录表 iter = 1; % 迭代次数初值 iter_max = 100; % 最大迭代次数 Route_best = zeros(iter_max,n); % 各代最佳路径 Length_best = zeros(iter_max,1); % 各代最佳路径的长度 Length_ave = zeros(iter_max,1); % 各代路径的平均长度 Limit_iter = 0; % 程序收敛时迭代次数 %% 迭代寻找最佳路径 while iter <= iter_max % 随机产生各个蚂蚁的起点城市 start = zeros(m,1); for i = 1:m temp = 1; start(i) = temp(1); end Table(:,1) = start; % 构建解空间 citys_index = 1:n; % 逐个蚂蚁路径选择 for i = 1:m % 逐个城市路径选择 for j = 2:n tabu = Table(i,1:(j - 1)); % 已访问的城市集合(禁忌表) allow_index = ~ismember(citys_index,tabu); % 参加说明1(程序底部) allow = citys_index(allow_index); % 待访问的城市集合 P = allow; % 计算城市间转移概率 for k = 1:length(allow) P(k) = Tau(tabu(end),allow(k))^alpha * Heu_F(tabu(end),allow(k))^beta; end P = P/sum(P); % 轮盘赌法选择下一个访问城市 Pc = cumsum(P); %参加说明2(程序底部) target_index = find(Pc >= rand); target = allow(target_index(1)); Table(i,j) = target; end end % 计算各个蚂蚁的路径距离 Length = zeros(m,1); for i = 1:m Route = Table(i,:); for j = 1:(n - 1) Length(i) = Length(i) + D(Route(j),Route(j + 1)); end Length(i) = Length(i) + D(Route(n),Route(1)); end % 计算最短路径距离及平均距离 if iter == 1 [min_Length,min_index] = min(Length); Length_best(iter) = min_Length; Length_ave(iter) = mean(Length); Route_best(iter,:) = Table(min_index,:); Limit_iter = 1; else [min_Length,min_index] = min(Length); Length_best(iter) = min(Length_best(iter - 1),min_Length); Length_ave(iter) = mean(Length); if Length_best(iter) == min_Length Route_best(iter,:) = Table(min_index,:); Limit_iter = iter; else Route_best(iter,:) = Route_best((iter-1),:); end end % 更新信息素 Delta_Tau = zeros(n,n); % 逐个蚂蚁计算 for i = 1:m % 逐个城市计算 for j = 1:(n - 1) Delta_Tau(Table(i,j),Table(i,j+1)) = Delta_Tau(Table(i,j),Table(i,j+1)) + Q/Length(i); end Delta_Tau(Table(i,n),Table(i,1)) = Delta_Tau(Table(i,n),Table(i,1)) + Q/Length(i); end Tau = (1-vol) * Tau + Delta_Tau; % 迭代次数加1,清空路径记录表 iter = iter + 1; Table = zeros(m,n); end %% 结果显示 [Shortest_Length,index] = min(Length_best); Shortest_Route = Route_best(index,:); Time_Cost=etime(clock,t0); disp(['最短距离:' num2str(Shortest_Length)]); disp(['最短路径:' num2str([Shortest_Route Shortest_Route(1)])]); disp(['收敛迭代次数:' num2str(Limit_iter)]); disp(['程序执行时间:' num2str(Time_Cost) '秒']); %% 绘图 figure(1) plot([citys(Shortest_Route,1);citys(Shortest_Route(1),1)],... %三点省略符为Matlab续行符 [citys(Shortest_Route,2);citys(Shortest_Route(1),2)],'o-'); grid on for i = 1:size(citys,1) text(citys(i,1),citys(i,2),[' ' num2str(i)]); end text(citys(Shortest_Route(1),1),citys(Shortest_Route(1),2),' 起点'); text(citys(Shortest_Route(end),1),citys(Shortest_Route(end),2),' 终点'); xlabel('城市位置横坐标') ylabel('城市位置纵坐标') title(['ACA最优化路径(最短距离:' num2str(Shortest_Length) ')']) figure(2) plot(1:iter_max,Length_best,'b') legend('最短距离') xlabel('迭代次数') ylabel('距离') title('算法收敛轨迹') %-------------------------------------------------------------------------- %% 程序解释或说明 % 1. ismember函数判断一个变量中的元素是否在另一个变量中出现,返回0-1矩阵; % 2. cumsum函数用于求变量中累加元素的和,如A=[1, 2, 3, 4, 5], 那么cumsum(A)=[1, 3, 6, 10, 15]。
运行结果
最短距离:433.5578
最短路径:1 2 8 3 10 7 9 11 12 13 14 15 16 17 28 6 4 5 29 23 22 25 24 30 27 26 20 19 18 21 1
收敛迭代次数:5
程序执行时间:9.502秒
退火算法
clear clc num=xlsread('C题附件1.xlsx', 'B2:C31'); x=xlsread('C题附件1.xlsx','B2:B31'); y=xlsread('C题附件1.xlsx','C2:C31'); a = 0.99; % 温度衰减函数的参数 t0 = 97; tf = 3; t = t0; Markov_length = 10000; % Markov链长度 coordinates = [num,x,y]; coordinates(:,1) = []; amount = size(coordinates,1); % 城市的数目 %% 计算城市间相互距离 n = size(num,1); D = zeros(n,n); r=6371; for i = 1:n for j = 1:n if i ~= j D(i,j) = r*acos(cos(y(i))*cos(y(j))*cos(x(i)-x(j))+sin(y(i))*sin(y(j))); else D(i,j) = 1e-4; %设定的对角矩阵修正值 end end end sol_new = 1:amount; % 产生初始解 % sol_new是每次产生的新解;sol_current是当前解;sol_best是冷却中的最好解; E_current = inf;E_best = inf; % E_current是当前解对应的回路距离; % E_new是新解的回路距离; % E_best是最优解的 sol_current = sol_new; sol_best = sol_new; p = 1; while t>=tf for r=1:Markov_length % Markov链长度 % 产生随机扰动 if (rand < 0.5) % 随机决定是进行两交换还是三交换 % 两交换 ind1 = 0; ind2 = 0; while (ind1 == ind2) ind1 = ceil(rand.*amount); ind2 = ceil(rand.*amount); end tmp1 = sol_new(ind1); sol_new(ind1) = sol_new(ind2); sol_new(ind2) = tmp1; else % 三交换 ind1 = 0; ind2 = 0; ind3 = 0; while (ind1 == ind2) || (ind1 == ind3) ... || (ind2 == ind3) || (abs(ind1-ind2) == 1) ind1 = ceil(rand.*amount); ind2 = ceil(rand.*amount); ind3 = ceil(rand.*amount); end tmp1 = ind1;tmp2 = ind2;tmp3 = ind3; % 确保ind1 < ind2 < ind3 if (ind1 < ind2) && (ind2 < ind3) ; elseif (ind1 < ind3) && (ind3 < ind2) ind2 = tmp3;ind3 = tmp2; elseif (ind2 < ind1) && (ind1 < ind3) ind1 = tmp2;ind2 = tmp1; elseif (ind2 < ind3) && (ind3 < ind1) ind1 = tmp2;ind2 = tmp3; ind3 = tmp1; elseif (ind3 < ind1) && (ind1 < ind2) ind1 = tmp3;ind2 = tmp1; ind3 = tmp2; elseif (ind3 < ind2) && (ind2 < ind1) ind1 = tmp3;ind2 = tmp2; ind3 = tmp1; end tmplist1 = sol_new((ind1+1):(ind2-1)); sol_new((ind1+1):(ind1+ind3-ind2+1)) = ... sol_new((ind2):(ind3)); sol_new((ind1+ind3-ind2+2):ind3) = ... tmplist1; end %检查是否满足约束 % 计算目标函数值(即内能) E_new = 0; for i = 1 : (amount-1) E_new = E_new + ... D(sol_new(i),sol_new(i+1)); end % 再算上从最后一个城市到第一个城市的距离 E_new = E_new + ... D(sol_new(amount),sol_new(1)); if E_new < E_current E_current = E_new; sol_current = sol_new; if E_new < E_best % 把冷却过程中最好的解保存下来 E_best = E_new; sol_best = sol_new; end else % 若新解的目标函数值小于当前解的, % 则仅以一定概率接受新解 if rand < exp(-(E_new-E_current)./t) E_current = E_new; sol_current = sol_new; else sol_new = sol_current; end end end t=t.*a; % 控制参数t(温度)减少为原来的a倍 end disp('最优解为:') disp(sol_best) disp('最短距离:') disp(E_best)
运行结果:
最优解为:
1 至 22 列
1 至 22 列
1 5 18 21 19 20 26 27 30 24 25 22 23 29 4 6 11 13 14 17 28 16 15 12
23 至 30 列
9 7 10 3 8 2
最短距离:
405.2805
405.2805
贪心算法
n = 30 ; %用于记录点数 best = 1:1:n; %生成一个用来存储点顺序的矩阵 handle = 1:1:n; x=xlsread('C题附件1.xlsx','B2:B31'); y=xlsread('C题附件1.xlsx','C2:C31'); r=6371; D = zeros(n) ; for i = 1 : n for j = 1 : n D(i,j) = r*acos(cos(y(i))*cos(y(j))*cos(x(i)-x(j))+sin(y(i))*sin(y(j))); %距离矩阵 end end best(1) = 1; %默认起点 num = 1; for a = 1:(n-2) %需要n-2次判断 handle(:,1)=[]; %上一次最优点的数据裁掉 dis = zeros(1,(n-a)); %用来存剩下各个点的距离 for b = 1:(n-a) %用来获取剩下各个点的距离 dis(b) = D (num , handle(b)); end num1 = find( dis == min(dis) ); %得到最优点所在检索 t = handle(1); %将最优点与最前面的点位置进行交换 handle(1) = handle(num1); handle(num1) = t; num = handle(1); %获取下次进行操作的数 best(a+1) = handle(1); %将最优点存入best数组 end best(n) = handle(num1); %补上最后一个点 plot(x(best),y(best),'-+') ; %用'+'标出点并用实线连接得到最优路径 grid on disp(best)
运行结果:
最短路径:
1 至 22 列
1 2 8 10 3 4 6 11 9 7 12 13 14 17 16 15 28 5 29 23 22 24
23 至 30 列
25 20 26 30 27 19 21 18
最短距离:484.4739
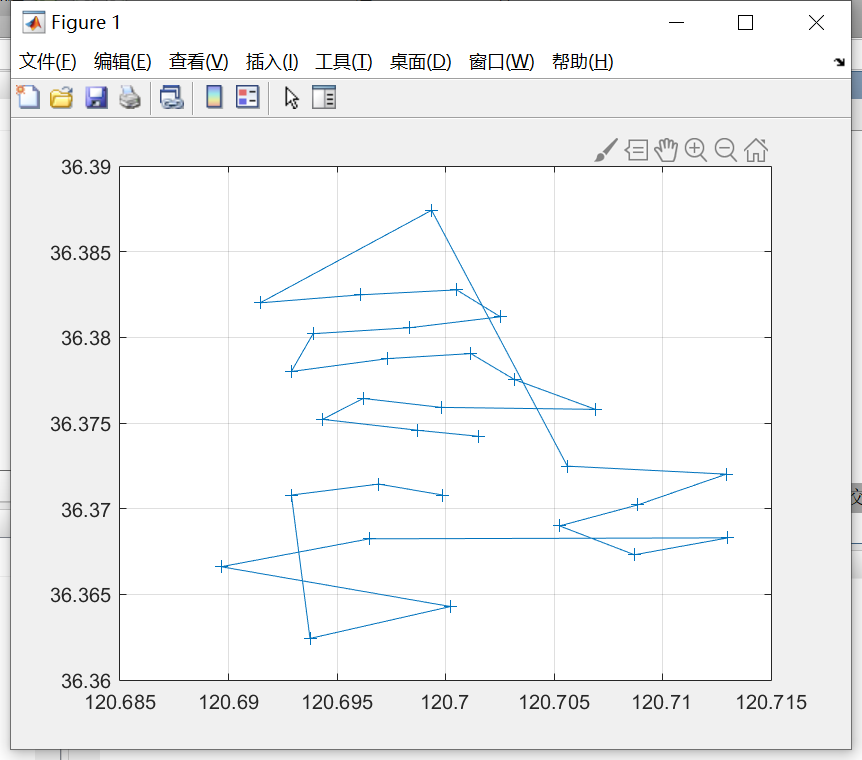
遗传算法
运行结果:
最优路径:1 7 9 12 11 17 28 16 15 13 14 6 8 4 23 22 24 20 30 27 26 25 21 18 29 2 3 10 5 19
最短距离:597.9907
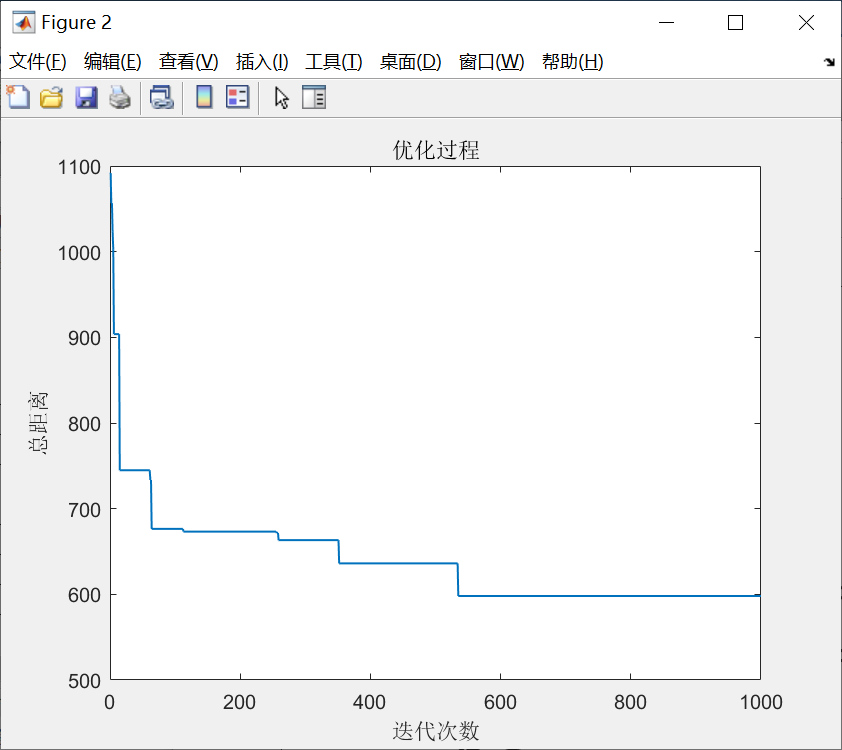
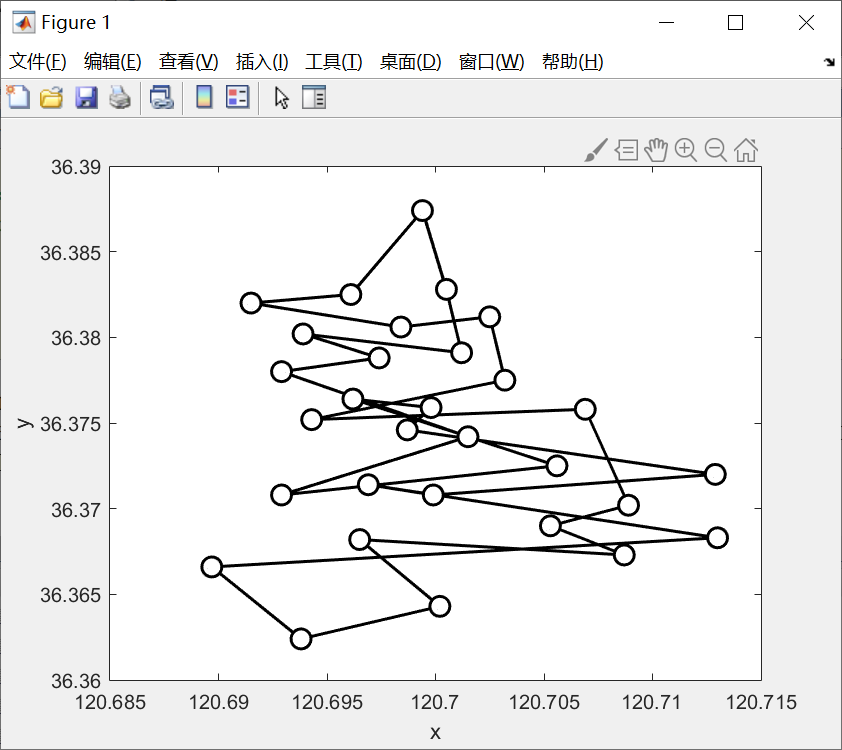
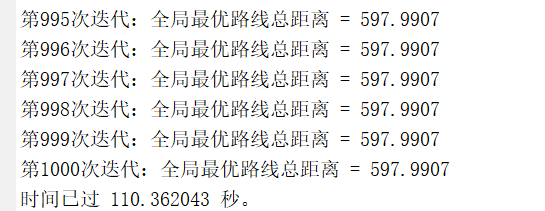