MobileNetV2: Inverted Residuals and Linear Bottlenecks, CVPR 2018.
谷歌公司
MobileNet V1 结构非常简单, 发明了 Depthwise 和 Pointwise 卷积,但是没有使用RestNet里的residual learning;另一方面,Depthwise Conv确实是大大降低了计算量,但实际中,发现不少训练出来的kernel是空的。
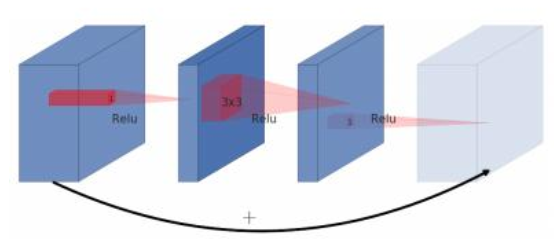
ResNet 中的 bottleneck (两头粗,中间细,所以得名),先用1x1卷积把通道数由256降到64,然后进行3x3卷积,不然中间3x3卷积计算量太大。所以bottleneck是两边宽中间窄(也是名字的由来)。
但是在 MobilenetV2中,反了过来,输入是24维,输出也是24维,但中间我们扩展了6倍。网络是中间胖、两头窄,所以叫做 Inverted residule block。
其中的原理是:通道越少,卷积层的乘法计算量就越小。那么如果整个网络都是低维的通道,那么整体计算速度就会很快。然而,这样效果并不好,没有办法提取到整体的足够多的信息。所以,如果提取特征数据的话,我们可能更希望有高维的通道来做这个事情。V2就设计这样一个结构来达到平衡。
V2中首先扩展维度,然后用depthwise conv来提取特征,最后再压缩数据,让网络变小。
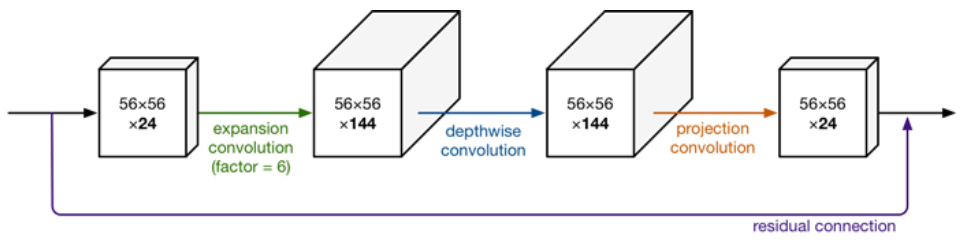
网络的整体结构如下,同时,性能进一步得到了提升。
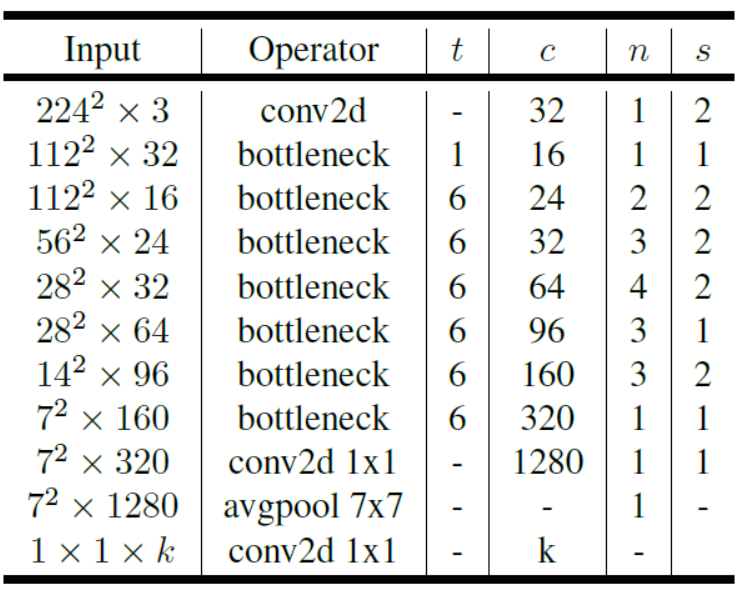
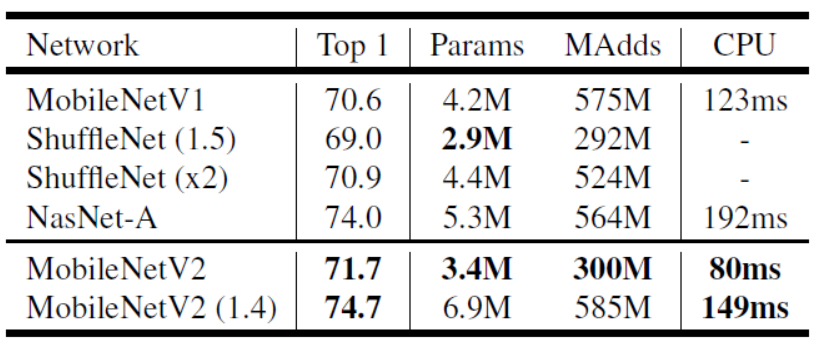
代码如下,也比较容易理解:
# 通过 expansion 增大 feature map 的数量
planes = expansion * in_planes
self.conv1 = nn.Conv2d(in_planes, planes, kernel_size=1, stride=1,
padding=0, bias=False)
self.bn1 = nn.BatchNorm2d(planes)
self.conv2 = nn.Conv2d(planes, planes, kernel_size=3, stride=stride,
padding=1, groups=planes, bias=False)
self.bn2 = nn.BatchNorm2d(planes)
self.conv3 = nn.Conv2d(planes, out_planes, kernel_size=1, stride=1,
padding=0, bias=False)
self.bn3 = nn.BatchNorm2d(out_planes)