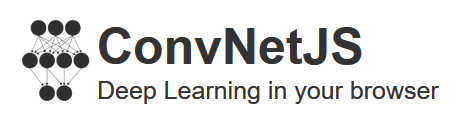
这只是一个展示项目,但是能够帮助直观地看到一些东西
地址:https://cs.stanford.edu/people/karpathy/convnetjs/demo/cifar10.html
layer_defs = [];
layer_defs.push({type:'input', out_sx:32, out_sy:32, out_depth:3});
layer_defs.push({type:'conv', sx:5, filters:16, stride:1, pad:2, activation:'relu'});
layer_defs.push({type:'pool', sx:2, stride:2});
layer_defs.push({type:'conv', sx:5, filters:20, stride:1, pad:2, activation:'relu'});
layer_defs.push({type:'pool', sx:2, stride:2});
layer_defs.push({type:'conv', sx:5, filters:20, stride:1, pad:2, activation:'relu'});
layer_defs.push({type:'pool', sx:2, stride:2});
layer_defs.push({type:'softmax', num_classes:10});
net = new convnetjs.Net();
net.makeLayers(layer_defs);
网络的构造就是conv-pool-conv-pool这样的过程。

原始图片输入
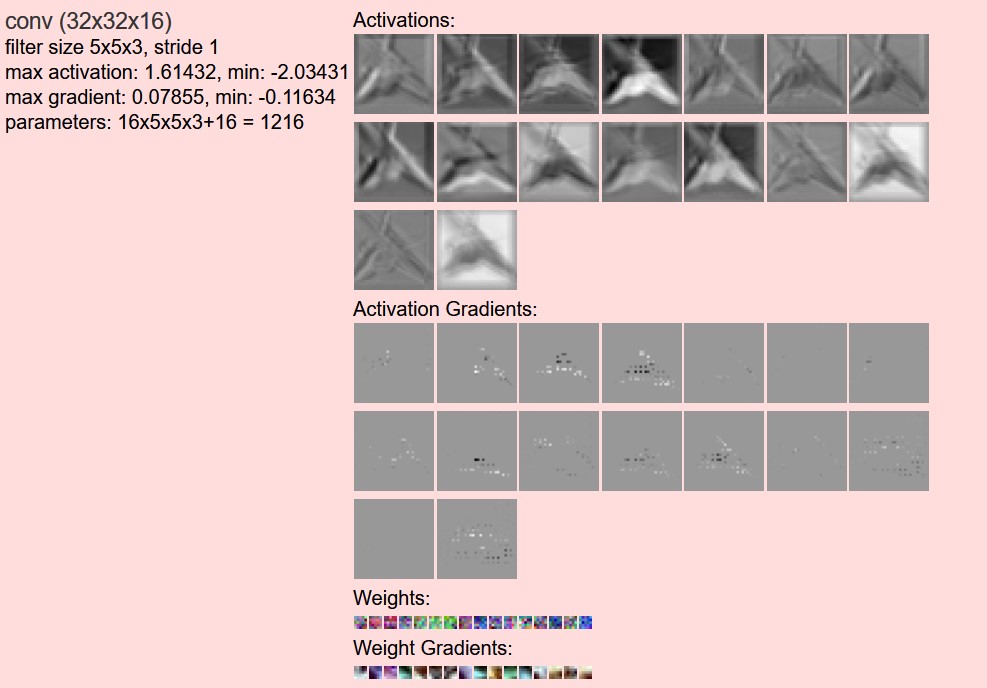
第一层卷积
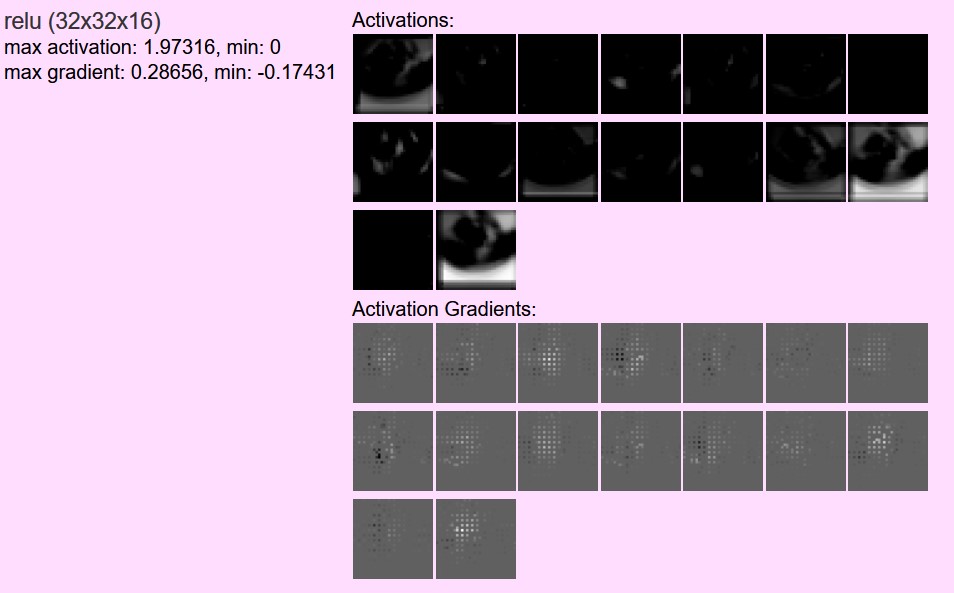
和relu
第一层pool
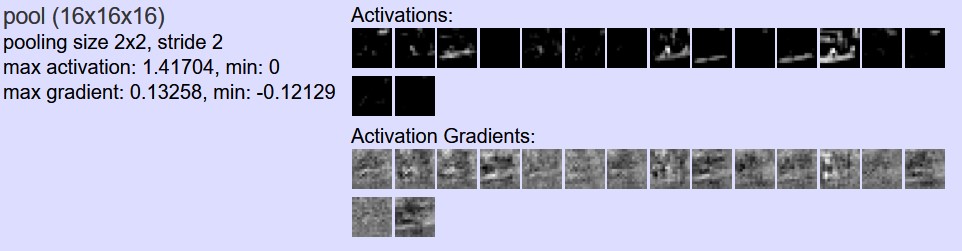
可以返现,图片的大小降低明显
第二次
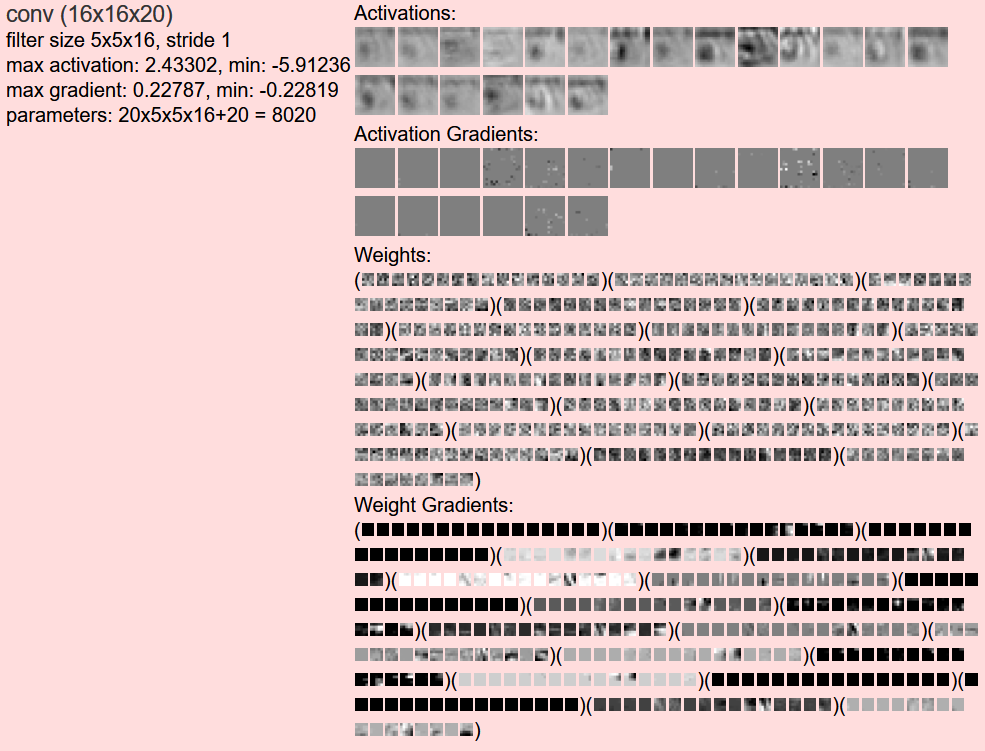
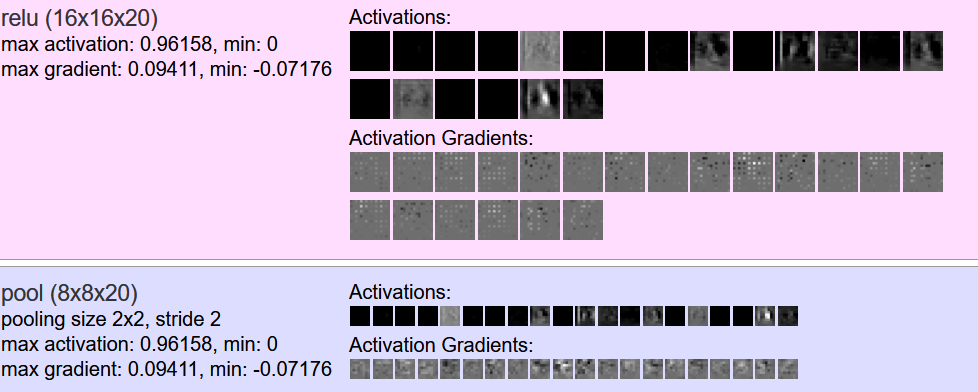
第三次
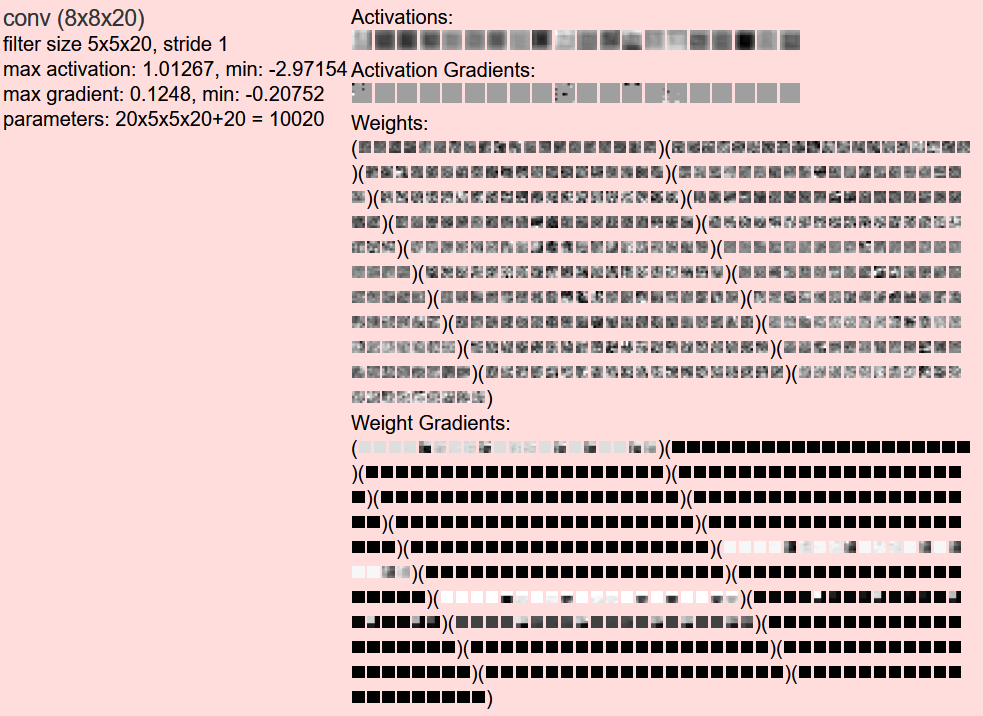
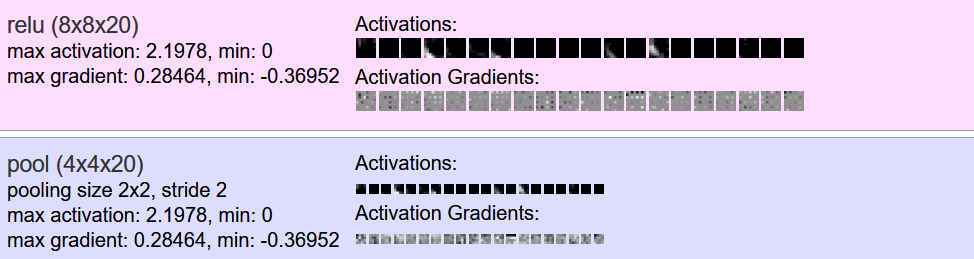
最后,全连接并softmax
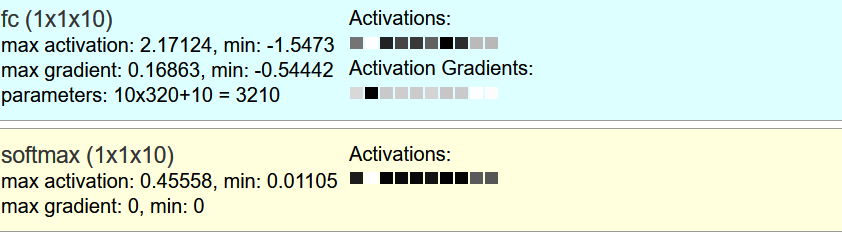
结束了。基本能够理解。
可以预见的一点是,在这样一个发展迅速的时代,拥有数据分析背景、能力,特别是深度学习技术,将使工程师具备价值。要想办法克服显示的瓶颈,成为这样的人。毕竟,真正的牛人是创造规则的。