In this chapter, we will cover:
- Detecting image contours with the Canny operator
- Detecting lines in images with the Hough transform
- Fitting a line to a set of points
- Extracting the components' contours
- Computing components' shape descriptors
- Detecting image contours with the Canny operator
The Canny algorithm is implemented in OpenCV by the function cv::Canny. As will be explained, this algorithm requires the specification of two thresholds. The call to the function is therefore as follows:
// Apply Canny algorithm cv::Mat contours; cv::Canny(image, // gray-level image contours, // output contours 125, // low threshold 350); // high threshold
When applied on the following image: The result is as follows:
Note that to obtain an image as shown in the preceding screenshot, we had to invert the black and white values since the normal result represents contours by non-zero pixels. The inverted representation, which is nicer to print on a page, is simply produced as follows:
cv::Mat contoursInv; // inverted image cv::threshold(contours,contoursInv, 128, // values below this 255, // becomes this cv::THRESH_BINARY_INV);
Detecting lines in images with the Hough transform
With the Hough transform, lines are represented using the following equation:
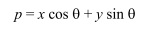
The output of the cv::HoughLinesfunction is a vector of cv::Vec2felements, each of them being a pair of floating point values which represents the parameters of a detected line (ρ , θ).
cv::Mat image = cv:: imread("../road.jpg" , 0 ); if (! image.data ) { return 0 ; } cv ::namedWindow( "Original Image" ); cv ::imshow( "Original Image" , image); // Apply Canny algorithm cv ::Mat contours; cv ::Canny( image, contours , 125 , 350 ); cv ::namedWindow( "Canny edges" ); cv ::imshow( "Canny edges" , contours); cv ::Mat result( contours.rows ,contours. cols,CV_8U ,cv:: Scalar(255 )); image .copyTo( result); // Hough transform for line detection std ::vector< cv::Vec2f > lines; cv ::HoughLines( contours, lines , 1, PI / 180 , // step size 80); // minimum number of votes std ::vector< cv::Vec2f >::const_iterator it = lines.begin (); while ( it != lines .end()) { float rho = (*it )[0]; // first element is distance rho float theta = (*it )[1]; // second element is angle theta if ( theta < PI /4. || theta > 3.* PI/4. ) { // ~vertical line // point of intersection of the line with first row cv ::Point pt1( rho / cos (theta), 0); // point of intersection of the line with last row cv ::Point pt2(( rho - result .rows * sin(theta )) / cos(theta ), result. rows); // draw a while line cv ::line( result, pt1 , pt2, cv::Scalar (255), 1); } else { //~horizontal line // point of intersection of the line with first column cv ::Point pt1( 0, rho / sin( theta)); // point of intersection of the line with last column cv ::Point pt2( result.cols , ( rho - result .cols * cos(theta )) / sin(theta )); // draw a white line cv ::line( result, pt1 , pt2, cv::Scalar (255), 1); } ++it; } cv ::namedWindow( "Detected lines with hough" ); cv ::imshow( "Detected lines with hough" , result);
gets the following results:
As it can be seen, the Hough transform simply looks for an alignment of edge pixels across the image. This can potentially create some false detection due to an incidental pixel alignment, or multiple detections when several lines pass through the same alignment of pixels.
To overcome some of these problems, and to allow line segments to be detected (that is, with end points), a variant of the transform has been proposed. This is the Probabilistic Hough transform and it is implemented in OpenCV as function cv::HoughLinesP. We use it here to create our LineFinderclass that encapsulates the function parameters:
linefinder.hpp:
#if ! defined LINE_FINDER #define LINE_FINDER #include <opencv2/core/core.hpp> #include <opencv2/highgui/highgui.hpp> #include <opencv2/imgproc/imgproc.hpp> #include <vector> #define PI 3.1415926 class LineFinder { private: // original image cv ::Mat img; // vector containing the end points // of the detected lines std ::vector< cv::Vec4i > lines; // accumulator resolution parameters double deltaRho; double deltaTheta; // minimum number of votes that a line // must receive before being considered int minVote; // min length of a line double minLength; // max allowed gap along the line double maxGap; public: // Default accumulator resolution is 1 pixel by 1 degree // no gap, no minimum length LineFinder () : deltaRho(1 ), deltaTheta( PI / 180), minVote (10), minLength(0. ), maxGap( 0.) {} // Set the resolution of the accumulator void setAccResolution( double dRho, double dTheta ) { deltaRho = dRho; deltaTheta = dTheta; } // Set the minimum number of votes void setMinVote( int minV) { minVote = minV; } // Set line length and gap void setLineLengthAndGap( double length, double gap ) { minLength = length; maxGap = gap; } // Apply probabilistic Hough Transform std ::vector< cv::Vec4i > findLines( cv::Mat &binary) { lines .clear(); cv ::HoughLinesP( binary, lines , deltaRho , deltaTheta, minVote, minLength , maxGap); return lines; } // Draw the detected lines on image void drawDetectedLines( cv::Mat &image, cv ::Scalar color = cv::Scalar (255, 255, 255)) { // Draw the lines std ::vector< cv::Vec4i >::const_iterator it2 = lines.begin (); while ( it2 != lines .end()){ cv ::Point pt1((* it2)[0 ], (* it2)[1 ]); cv ::Point pt2((* it2)[2 ], (* it2)[3 ]); cv ::line( image, pt1 , pt2, color); ++ it2; } } }; #endif
main.cpp:
// Create LineFinder instance LineFinder finder ; // Set probabilistic Hough parameters finder .setLineLengthAndGap( 100, 20); finder .setMinVote( 80); // Detect lines and draw them std ::vector< cv::Vec4i > linesP = finder.findLines (contours); finder .drawDetectedLines( image); cv ::namedWindow( "Detected Lines with HoughP" ); cv ::imshow( "Detected Lines with HoughP" , image);
result:
Detecting circles
In the case of circles, the corresponding parametric equation is:
In the case of circles, the corresponding parametric equation is:
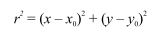
image = cv ::imread( "../chariot.jpg" , 0 ); cv ::GaussianBlur( image, image , cv:: Size(5 , 5 ), 1.5 ); std ::vector< cv::Vec3f > circles; cv ::HoughCircles( image, circles , CV_HOUGH_GRADIENT, 2, // accumulator resolution (size of the image / 2) 50, // minimum distance between two circles 200, // Canny high threshold 100, // minimum number of votes 25, 100); // min and max radius std ::vector< cv::Vec3f >::const_iterator itc = circles.begin (); while ( itc != circles .end()) { cv ::circle( image, cv ::Point((* itc)[0 ], (* itc)[1 ]), // circle centre (*itc)[ 2], // circle radius cv ::Scalar( 255), // color 2 // thickness ); ++ itc; } cv ::namedWindow( "Detected Circles" ); cv ::imshow( "Detected Circles" , image);
result:
Fitting a line to a set of points
// Fitting a line to a set of points int n = 0; // we select line 0 // black image cv ::Mat oneline( contours.size (), CV_8U, cv::Scalar (0)); // white line cv ::line( oneline, cv ::Point( linesP[n ][0], linesP[n ][1]), cv ::Point( linesP[n ][2], linesP[n ][3]), cv ::Scalar( 255), 5); // contours And white line cv ::bitwise_and( contours, oneline , oneline); cv ::namedWindow( "One line" ); cv ::imshow( "One line" , oneline);
std::vector <cv:: Point> points ; // Iterate over the pixels to obtain all point positions for ( int y = 0; y < oneline .rows; y++) { // row y uchar *rowPtr = oneline.ptr <uchar>( y); for ( int x = 0; x < oneline .cols; x++) { // column x // if on a contour if ( rowPtr[x ]) { points .push_back( cv::Point (x, y)); } } } cv ::Vec4f line; cv ::fitLine( cv::Mat (points), line, CV_DIST_L2 , // distance type 0, // not used with L2 distance 0.01, 0.01 // accuracy ); int x0 = line[2 ]; // a point on the line int y0 = line[3 ]; int x1 = x0 - 200 * line[0 ]; // add a vector of length 200 int y1 = y0 - 200 * line[1 ]; // using the unit vector image = cv:: imread("../road.jpg" , 0 ); cv ::line( image, cv ::Point( x0, y0 ), cv:: Point(x1 , y1), cv::Scalar (0), 3); cv ::namedWindow( "Estimated line" ); cv ::imshow( "Estimated line" , image);
Extracting the components' contours
cv::Mat image = cv:: imread("../binaryGroup.bmp" , 0 ); if (! image.data ) { return 0 ; } cv ::namedWindow( "Binary Group" ); cv ::imshow( "Binary Group" , image); std ::vector< std::vector <cv:: Point>> contours ; cv ::findContours( image, contours , // a vector of contours CV_RETR_EXTERNAL , // retrieve the external contours CV_CHAIN_APPROX_NONE // all pixels of each contours ); // Draw black contours on a white image cv ::Mat result( image.size (), CV_8U, cv::Scalar (255)); cv ::drawContours( result, contours , -1, // draw all contours cv ::Scalar( 0), // in black 2 // with a thickness of 2 ); cv ::namedWindow( "Contours" ); cv ::imshow( "Contours" , result); //Eliminate too short or too long contours int cmin = 100; // minimum contour length int cmax = 1000; //maximum contour length std ::vector< std::vector <cv:: Point>>::const_iterator itc = contours. begin(); while ( itc != contours .end()) { if ( itc->size () < cmin || itc ->size() > cmax ) { itc = contours. erase(itc ); } else ++itc; } // draw contours on the original image cv ::Mat original = cv::imread ("../group.jpg"); cv ::drawContours( original, contours , - 1, cv ::Scalar( 255), 2); cv ::namedWindow( "Contours on Animals" ); cv ::imshow( "Contours on Animals" , original);
Computing components' shape descriptors
// draw contours on the white image result .setTo( cv::Scalar (255)); cv ::drawContours( result, contours , -1, // draw all contours cv ::Scalar( 0), // in black 2 // with a thickness of 2 ); cv ::namedWindow( "Contours on Animals" ); cv ::imshow( "Contours on Animals" , result); // Computing components' shape descriptor--------------------------- // testing the bounding box cv ::Rect r0 = cv::boundingRect (cv:: Mat(contours [0])); cv ::rectangle( result, r0 , cv:: Scalar(0 ), 2 ); // testing the enclosing circle float radius; cv ::Point2f center; cv ::minEnclosingCircle( cv::Mat (contours[ 1]), center , radius); cv ::circle( result, cv ::Point( center), static_cast<int >(radius), cv::Scalar (0), 2); // testing the approximate polygon std ::vector< cv::Point > poly; cv ::approxPolyDP( cv::Mat (contours[ 2]), poly , 5 , true ); // Iterate over each segment and draw it std ::vector< cv::Point >::const_iterator itp = poly.begin (); while ( itp != (poly. end() - 1 )) { cv ::line( result, *itp, *(itp + 1 ), cv:: Scalar(0 ), 2 ); ++ itp; } // last point linked to first point cv ::line( result, *(poly. begin()), *(poly. end() - 1 ), cv:: Scalar(20 ), 2 ); // testing the convex hull std ::vector< cv::Point > hull; cv ::convexHull( cv::Mat (contours[ 3]), hull ); // testing the moments iterate over all contours itc = contours. begin(); while ( itc != contours .end()) { // compute all moments cv ::Moments mom = cv::moments (cv:: Mat(*itc ++)); // draw mass center cv ::circle( result, // position of mass center converted to integer cv ::Point( mom.m10 / mom. m00, mom .m01 / mom.m00 ), 2, cv ::Scalar( 0), 2 // draw black dot ); } cv ::namedWindow( "Some shape descriptors" ); cv ::imshow( "Some shape descriptors" , result);