Map负责过滤分发,reduce归并整理,从map输出到reduce输入就是shuffle过程。
实现的功能
分区
决定当前key交给哪个reduce处理
默认:按照key的hash值对reduce的个数取余进行分区
分组
将相同key的value合并
排序
按照key对每一个keyvalue进行排序,字典排序
过程
map端shuffle
spill阶段:溢写
每一个map task处理的结果会进入环形缓冲区(内存100M)
分区
对每一条key进行分区(标上交给哪个reduce)
hadoop 1 reduce0 hive 1 reduce0 spark 1 reduce1 hadoop 1 reduce0 hbase 1 reduce1
排序
按照key排序,将相同分区的数据进行分区内排序
hadoop 1 reduce0 hadoop 1 reduce0 hive 1 reduce0 hbase 1 reduce1 spark 1 reduce1
溢写
当整个缓冲区达到阈值80%,开始进行溢写
将当前分区排序后的数据写入磁盘变成一个文件file1
最终生成多个spill小文件
可以在mapred-site.xml中设置内存的大小和溢写的阈值
在mapred-site.xml中设置内存的大小 <property> <name>mapreduce.task.io.sort.mb</name> <value>100</value> </property> 在mapred-site.xml中设置内存溢写的阈值 <property> <name>mapreduce.task.io.sort.spill.percent</name> <value>0.8</value> </property>
merge:合并
将spill生成的多个小文件进行合并
排序:将相同分区的数据进行分区内排序,实现comparator比较器进行比较。最终形成一个文件。
file1 hadoop 1 reduce0 hadoop 1 reduce0 hive 1 reduce0 hbase 1 reduce1 spark 1 reduce1 file2 hadoop 1 reduce0 hadoop 1 reduce0 hive 1 reduce0 hbase 1 reduce1 spark 1 reduce1 end_file: hadoop 1 reduce0 hadoop 1 reduce0 hadoop 1 reduce0 hadoop 1 reduce0 hive 1 reduce0 hive 1 reduce0 hbase 1 reduce1 hbase 1 reduce1 spark 1 reduce1 spark 1 reduce1
map task 结束,通知app master,app master通知reduce拉取数据
reduce端shuffle
map task1 hadoop 1 reduce0 hadoop 1 reduce0 hadoop 1 reduce0 hadoop 1 reduce0 hive 1 reduce0 hive 1 reduce0 hbase 1 reduce1 hbase 1 reduce1 spark 1 reduce1 spark 1 reduce1 map task2 hadoop 1 reduce0 hadoop 1 reduce0 hadoop 1 reduce0 hadoop 1 reduce0 hive 1 reduce0 hive 1 reduce0 hbase 1 reduce1 hbase 1 reduce1 spark 1 reduce1 spark 1 reduce1
reduce启动多个线程通过http到每台机器上拉取属于自己分区的数据
reduce0: hadoop 1 reduce0 hadoop 1 reduce0 hadoop 1 reduce0 hadoop 1 reduce0 hadoop 1 reduce0 hadoop 1 reduce0 hadoop 1 reduce0 hadoop 1 reduce0 hive 1 reduce0 hive 1 reduce0 hive 1 reduce0 hive 1 reduce0
merge:合并,将每个map task的结果中属于自己的分区数据进行合并
排序:对整体属于我分区的数据进行排序
分组:对相同key的value进行合并,使用comparable完成比较。
hadoop,list<1,1,1,1,1,1,1,1> hive,list<1,1,1,1>
优化
combine
在map阶段提前进行一次合并。一般等同于提前执行reduce
job.setCombinerClass(WCReduce.class);
compress
压缩中间结果集,减少磁盘IO以及网络IO
压缩配置方式
1.default:所有hadoop中默认的配置项 2.site:用于自定义配置文件,如果修改以后必须重启生效 3.conf对象配置每个程序的自定义配置 4.运行时通过参数实现用户自定义配置 bin/yarn jar xx.jar -Dmapreduce.map.output.compress=true -Dmapreduce.map.output.compress.codec=org.apache.hadoop.io.compress.Lz4Codec main_class input_path ouput_path
查看本地库支持哪些压缩
bin/hadoop checknative
通过conf配置对象配置压缩
public static void main(String[] args) { Configuration configuration = new Configuration(); //配置map中间结果集压缩 configuration.set("mapreduce.map.output.compress","true"); configuration.set("mapreduce.map.output.compress.codec", "org.apache.hadoop.io.compress.Lz4Codec"); //配置reduce结果集压缩 configuration.set("mapreduce.output.fileoutputformat.compress","true"); configuration.set("mapreduce.output.fileoutputformat.compress.codec", "org.apache.hadoop.io.compress.Lz4Codec"); try { int status = ToolRunner.run(configuration, new MRDriver(), args); System.exit(status); } catch (Exception e) { e.printStackTrace(); } }
通过自定义配置文件site-xml
针对Map Output数据进行压缩设置
对于MR程序来说:
提交任务的时候使用参数配置
提交任务的时候使用参数配置
mapreduce.map.output.compress=true mapreduce.map.output.compress.codec=org.apache.hadoop.io.compress.SnappyCodec
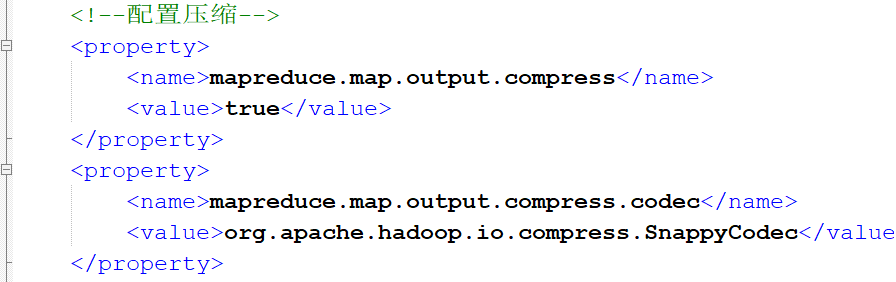
运行官方MapReduce案例 wordcount 可在Yarn上看到